Interpretable Stereotype Identification through Reasoning
CoRR(2023)
摘要
Given that language models are trained on vast datasets that may contain inherent biases, there is a potential danger of inadvertently perpetuating systemic discrimination. Consequently, it becomes essential to examine and address biases in language models, integrating fairness into their development to ensure these models are equitable and free from bias. In this work, we demonstrate the importance of reasoning in zero-shot stereotype identification based on Vicuna-13B-v1.3. While we do observe improved accuracy by scaling from 13B to 33B, we show that the performance gain from reasoning significantly exceeds the gain from scaling up. Our findings suggest that reasoning could be a key factor that enables LLMs to trescend the scaling law on out-of-domain tasks such as stereotype identification. Additionally, through a qualitative analysis of select reasoning traces, we highlight how reasoning enhances not just accuracy but also the interpretability of the decision.
更多查看译文
关键词
reasoning,identification
AI 理解论文
溯源树
样例
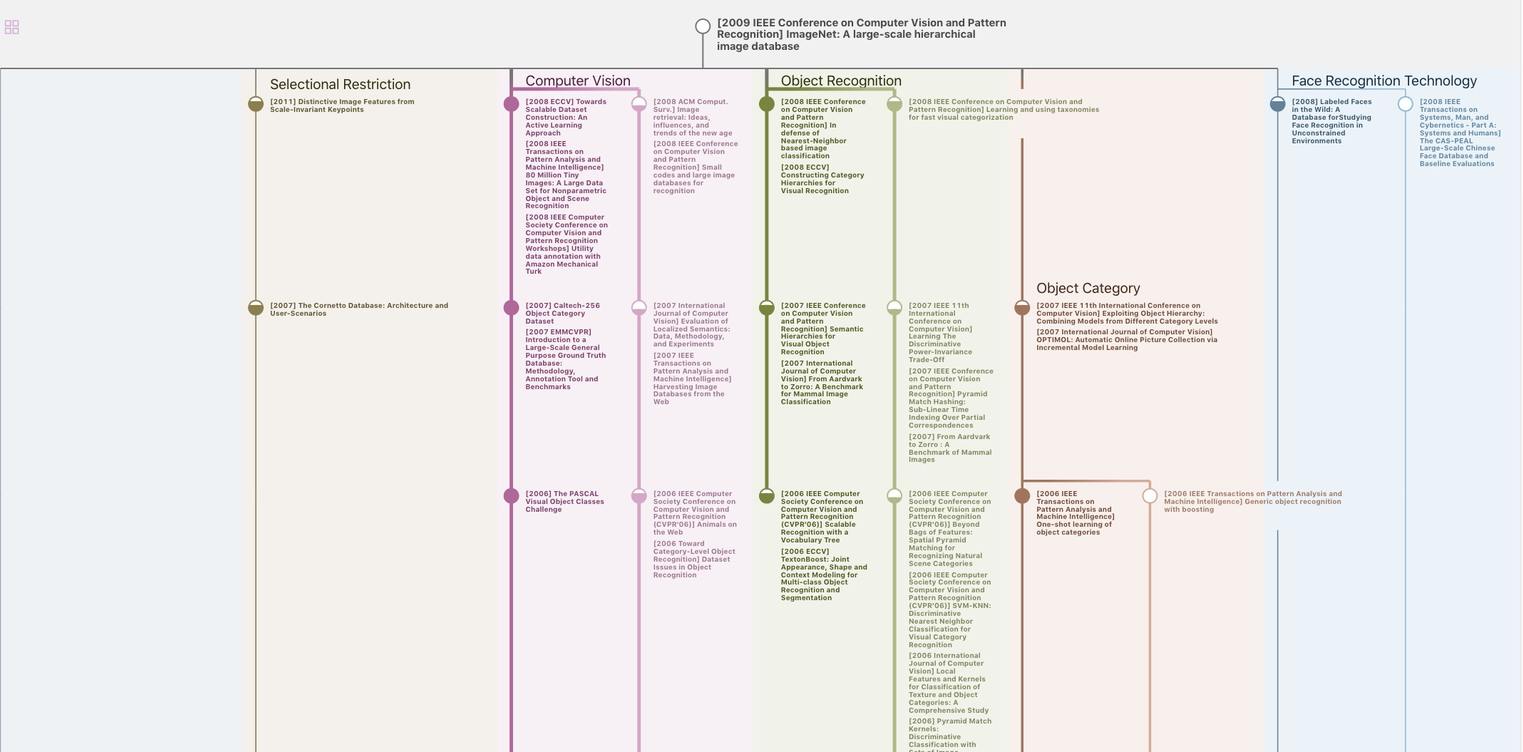
生成溯源树,研究论文发展脉络
Chat Paper
正在生成论文摘要