FinPT: Financial Risk Prediction with Profile Tuning on Pretrained Foundation Models
CoRR(2023)
摘要
Financial risk prediction plays a crucial role in the financial sector. Machine learning methods have been widely applied for automatically detecting potential risks and thus saving the cost of labor. However, the development in this field is lagging behind in recent years by the following two facts: 1) the algorithms used are somewhat outdated, especially in the context of the fast advance of generative AI and large language models (LLMs); 2) the lack of a unified and open-sourced financial benchmark has impeded the related research for years. To tackle these issues, we propose FinPT and FinBench: the former is a novel approach for financial risk prediction that conduct Profile Tuning on large pretrained foundation models, and the latter is a set of high-quality datasets on financial risks such as default, fraud, and churn. In FinPT, we fill the financial tabular data into the pre-defined instruction template, obtain natural-language customer profiles by prompting LLMs, and fine-tune large foundation models with the profile text to make predictions. We demonstrate the effectiveness of the proposed FinPT by experimenting with a range of representative strong baselines on FinBench. The analytical studies further deepen the understanding of LLMs for financial risk prediction.
更多查看译文
关键词
financial risk prediction,risk prediction,foundation models,profile tuning
AI 理解论文
溯源树
样例
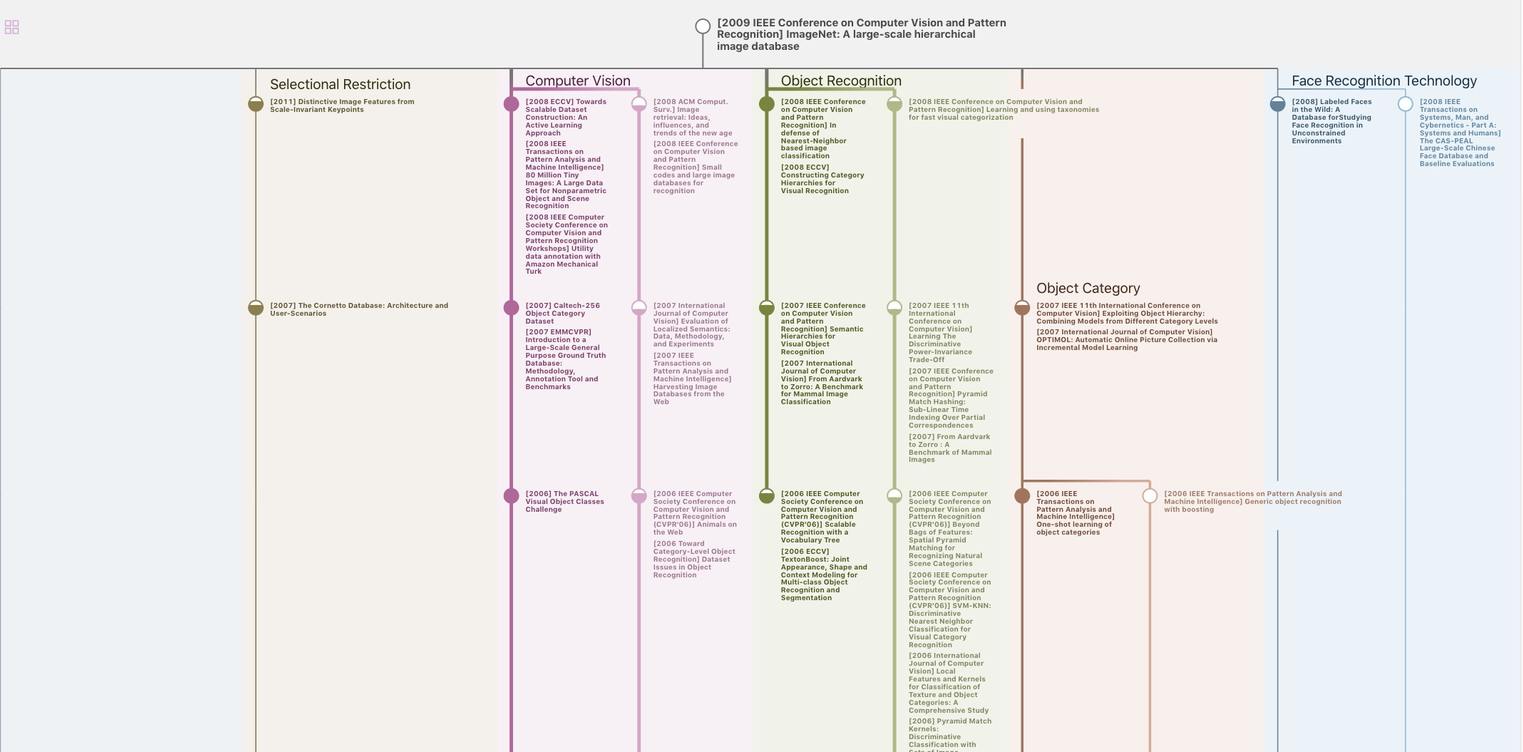
生成溯源树,研究论文发展脉络
Chat Paper
正在生成论文摘要