Lookbehind-SAM: k steps back, 1 step forward
CoRR(2023)
摘要
Sharpness-aware minimization (SAM) methods have gained increasing popularity
by formulating the problem of minimizing both loss value and loss sharpness as
a minimax objective. In this work, we increase the efficiency of the
maximization and minimization parts of SAM's objective to achieve a better
loss-sharpness trade-off. By taking inspiration from the Lookahead optimizer,
which uses multiple descent steps ahead, we propose Lookbehind, which performs
multiple ascent steps behind to enhance the maximization step of SAM and find a
worst-case perturbation with higher loss. Then, to mitigate the variance in the
descent step arising from the gathered gradients across the multiple ascent
steps, we employ linear interpolation to refine the minimization step.
Lookbehind leads to a myriad of benefits across a variety of tasks.
Particularly, we show increased generalization performance, greater robustness
against noisy weights, as well as improved learning and less catastrophic
forgetting in lifelong learning settings.
更多查看译文
关键词
steps
AI 理解论文
溯源树
样例
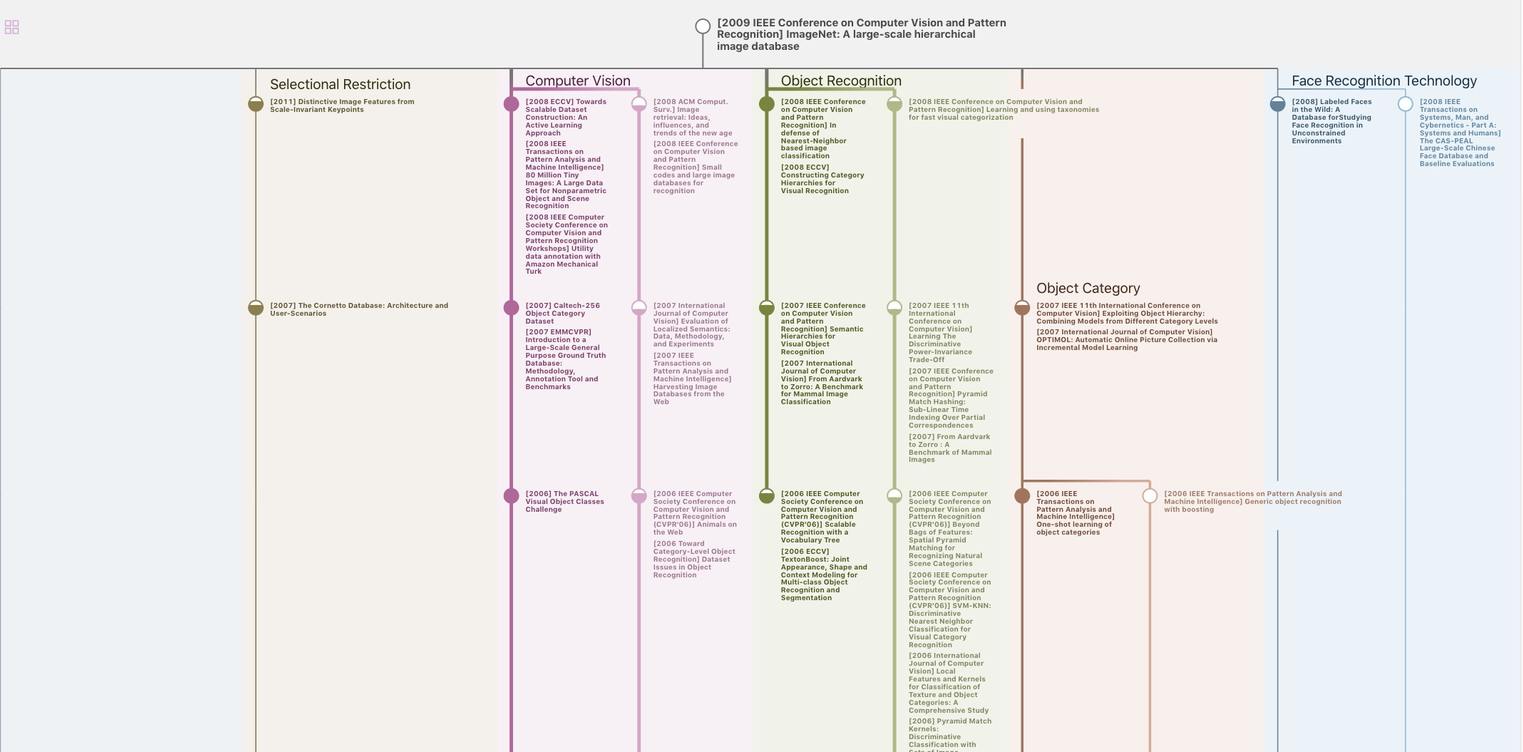
生成溯源树,研究论文发展脉络
Chat Paper
正在生成论文摘要