Comparing normalizing flows and diffusion models for prosody and acoustic modelling in text-to-speech
CoRR(2023)
摘要
Neural text-to-speech systems are often optimized on L1/L2 losses, which make strong assumptions about the distributions of the target data space. Aiming to improve those assumptions, Normalizing Flows and Diffusion Probabilistic Models were recently proposed as alternatives. In this paper, we compare traditional L1/L2-based approaches to diffusion and flow-based approaches for the tasks of prosody and mel-spectrogram prediction for text-to-speech synthesis. We use a prosody model to generate log-f0 and duration features, which are used to condition an acoustic model that generates mel-spectrograms. Experimental results demonstrate that the flow-based model achieves the best performance for spectrogram prediction, improving over equivalent diffusion and L1 models. Meanwhile, both diffusion and flow-based prosody predictors result in significant improvements over a typical L2-trained prosody models.
更多查看译文
关键词
acoustic modelling,normalizing flows,prosody,diffusion models,text-to-speech
AI 理解论文
溯源树
样例
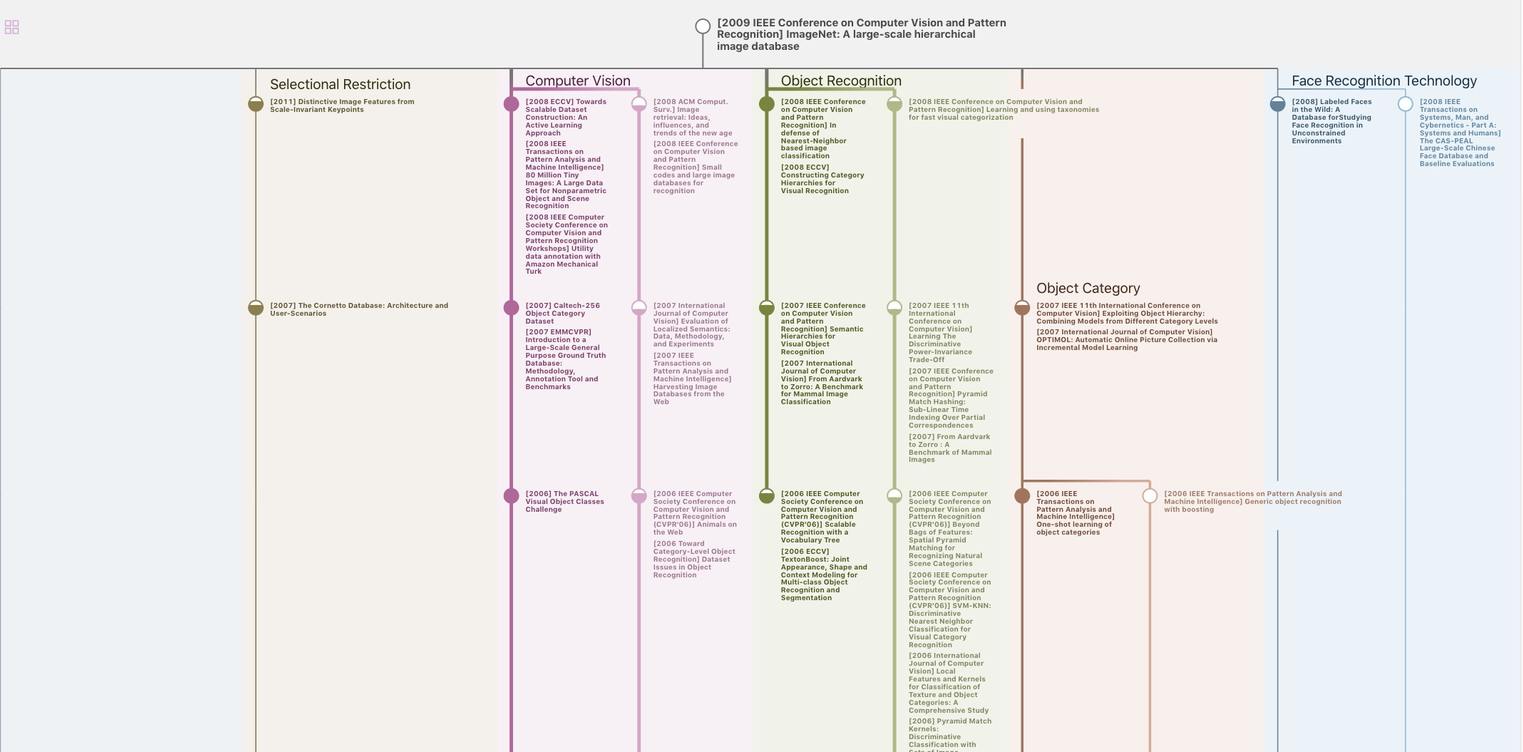
生成溯源树,研究论文发展脉络
Chat Paper
正在生成论文摘要