Uncertainty-Guided Spatial Pruning Architecture for Efficient Frame Interpolation
CoRR(2023)
摘要
The video frame interpolation (VFI) model applies the convolution operation to all locations, leading to redundant computations in regions with easy motion. We can use dynamic spatial pruning method to skip redundant computation, but this method cannot properly identify easy regions in VFI tasks without supervision. In this paper, we develop an Uncertainty-Guided Spatial Pruning (UGSP) architecture to skip redundant computation for efficient frame interpolation dynamically. Specifically, pixels with low uncertainty indicate easy regions, where the calculation can be reduced without bringing undesirable visual results. Therefore, we utilize uncertainty-generated mask labels to guide our UGSP in properly locating the easy region. Furthermore, we propose a self-contrast training strategy that leverages an auxiliary non-pruning branch to improve the performance of our UGSP. Extensive experiments show that UGSP maintains performance but reduces FLOPs by 34%/52%/30% compared to baseline without pruning on Vimeo90K/UCF101/MiddleBury datasets. In addition, our method achieves state-of-the-art performance with lower FLOPs on multiple benchmarks.
更多查看译文
关键词
spatial pruning architecture,efficient frame
AI 理解论文
溯源树
样例
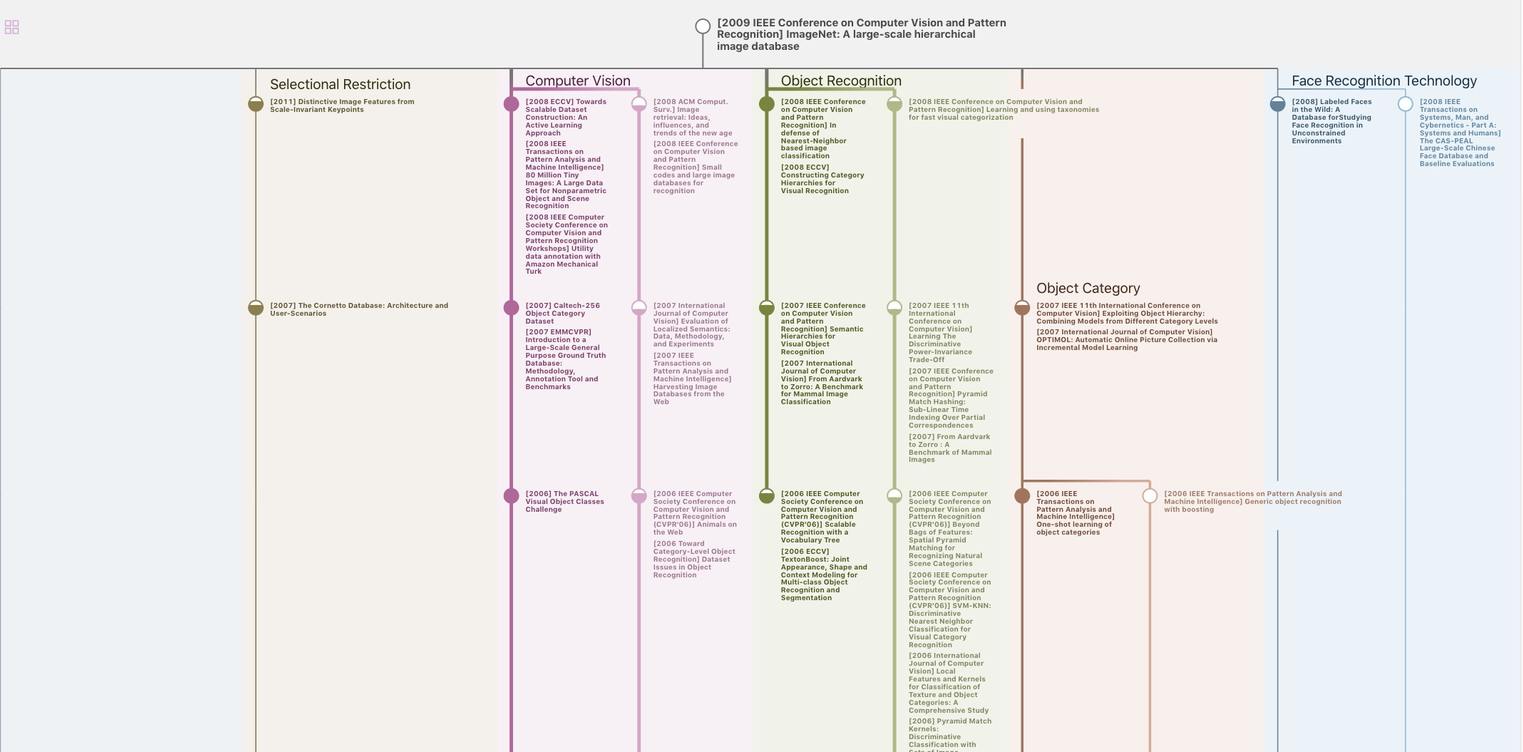
生成溯源树,研究论文发展脉络
Chat Paper
正在生成论文摘要