Don't be so negative! Score-based Generative Modeling with Oracle-assisted Guidance
CoRR(2023)
摘要
The maximum likelihood principle advocates parameter estimation via optimization of the data likelihood function. Models estimated in this way can exhibit a variety of generalization characteristics dictated by, e.g. architecture, parameterization, and optimization bias. This work addresses model learning in a setting where there further exists side-information in the form of an oracle that can label samples as being outside the support of the true data generating distribution. Specifically we develop a new denoising diffusion probabilistic modeling (DDPM) methodology, Gen-neG, that leverages this additional side-information. Our approach builds on generative adversarial networks (GANs) and discriminator guidance in diffusion models to guide the generation process towards the positive support region indicated by the oracle. We empirically establish the utility of Gen-neG in applications including collision avoidance in self-driving simulators and safety-guarded human motion generation.
更多查看译文
关键词
generative modeling,guidance,score-based,oracle-assisted
AI 理解论文
溯源树
样例
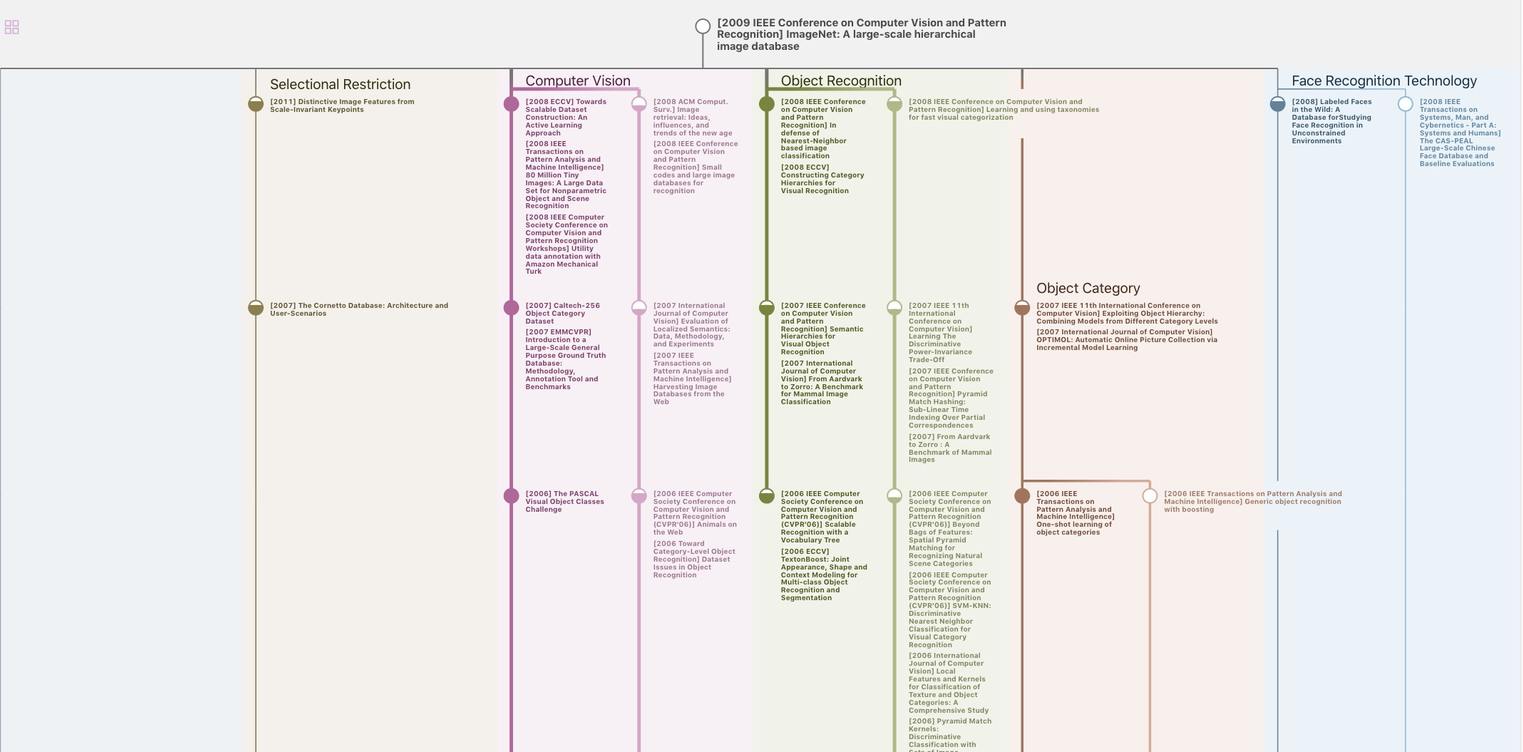
生成溯源树,研究论文发展脉络
Chat Paper
正在生成论文摘要