Toward Head Computed Tomography Image Reconstruction Standardization With Deep-Learning-Assisted Automatic Detection
IEEE TRANSACTIONS ON INSTRUMENTATION AND MEASUREMENT(2024)
摘要
Three-dimensional reconstruction of head computed tomography (CT) images elucidates the intricate spatial relationships of tissue structures, thereby assisting in accurate diagnosis. Nonetheless, securing an optimal head CT scan without deviation is challenging in clinical settings, owing to poor positioning by technicians, patient's physical constraints, or CT scanner tilt angle restrictions. Manual formatting and reconstruction not only introduce subjectivity but also strain time and labor resources. To address these issues, we propose an efficient automatic head CT images 3-D reconstruction method, improving accuracy and repeatability, as well as diminishing manual intervention. Our approach uses a deep-learning-based object detection algorithm, identifying and evaluating orbitomeatal line landmarks to automatically reformat the images prior to reconstruction. Given the dearth of existing evaluations of object detection algorithms in the context of head CT images, we compared 12 methods from both theoretical and experimental perspectives. By exploring their precision, efficiency, and robustness, we singled out the lightweight YOLOv8 as the aptest algorithm for our task, with an mAP of 92.77% and impressive robustness against class imbalance. Our qualitative evaluation of standardized reconstruction results demonstrates the clinical practicability and validity of our method.
更多查看译文
关键词
3-D reconstruction,deep learning,head computed tomography (CT) images,object detection,orbitomeatal line landmarks
AI 理解论文
溯源树
样例
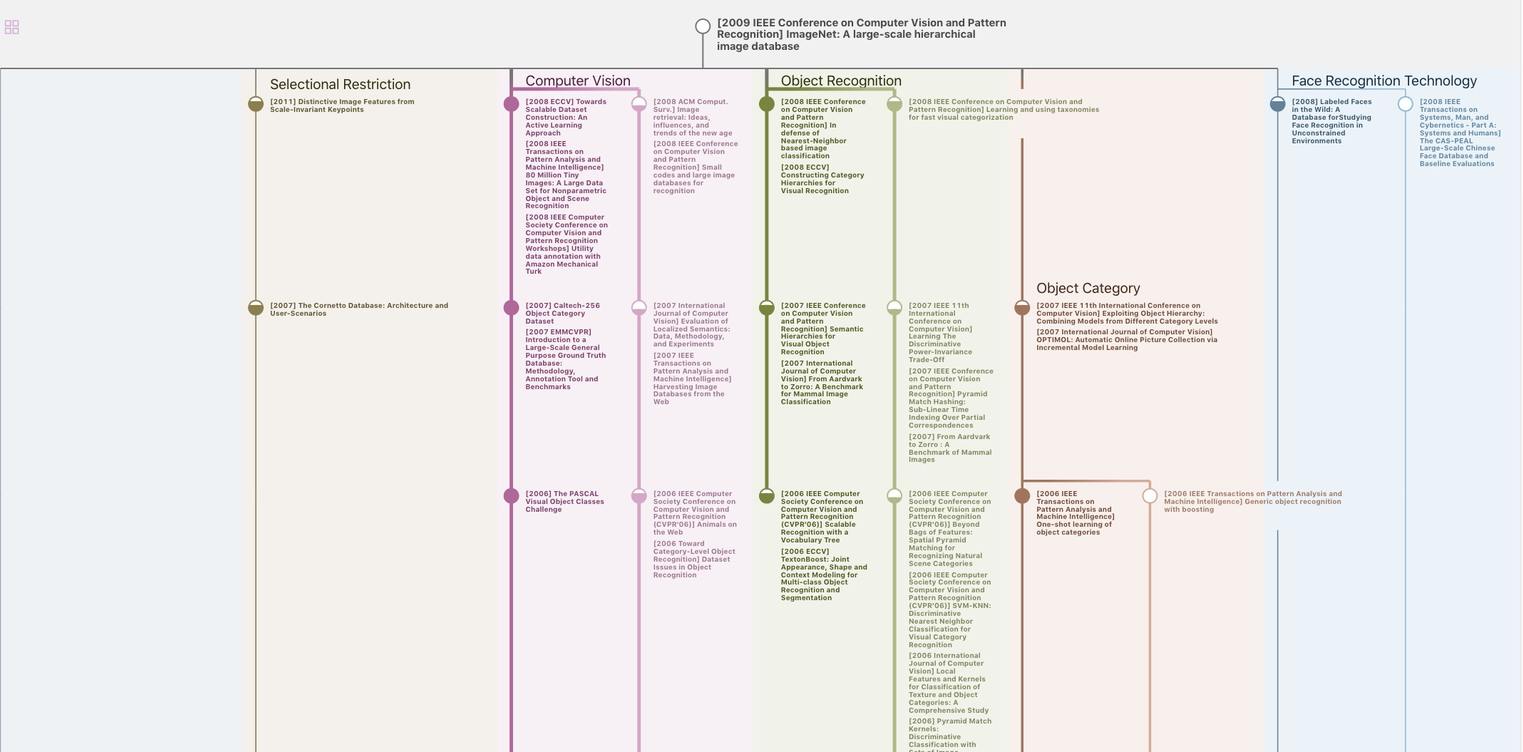
生成溯源树,研究论文发展脉络
Chat Paper
正在生成论文摘要