Guaranteed Optimal Generative Modeling with Maximum Deviation from the Empirical Distribution
CoRR(2023)
摘要
Generative modeling is a widely-used machine learning method with various applications in scientific and industrial fields. Its primary objective is to simulate new examples drawn from an unknown distribution given training data while ensuring diversity and avoiding replication of examples from the training data. This paper presents theoretical insights into training a generative model with two properties: (i) the error of replacing the true data-generating distribution with the trained data-generating distribution should optimally converge to zero as the sample size approaches infinity, and (ii) the trained data-generating distribution should be far enough from any distribution replicating examples in the training data. We provide non-asymptotic results in the form of finite sample risk bounds that quantify these properties and depend on relevant parameters such as sample size, the dimension of the ambient space, and the dimension of the latent space. Our results are applicable to general integral probability metrics used to quantify errors in probability distribution spaces, with the Wasserstein-$1$ distance being the central example. We also include numerical examples to illustrate our theoretical findings.
更多查看译文
关键词
optimal generative modeling,maximum deviation,distribution
AI 理解论文
溯源树
样例
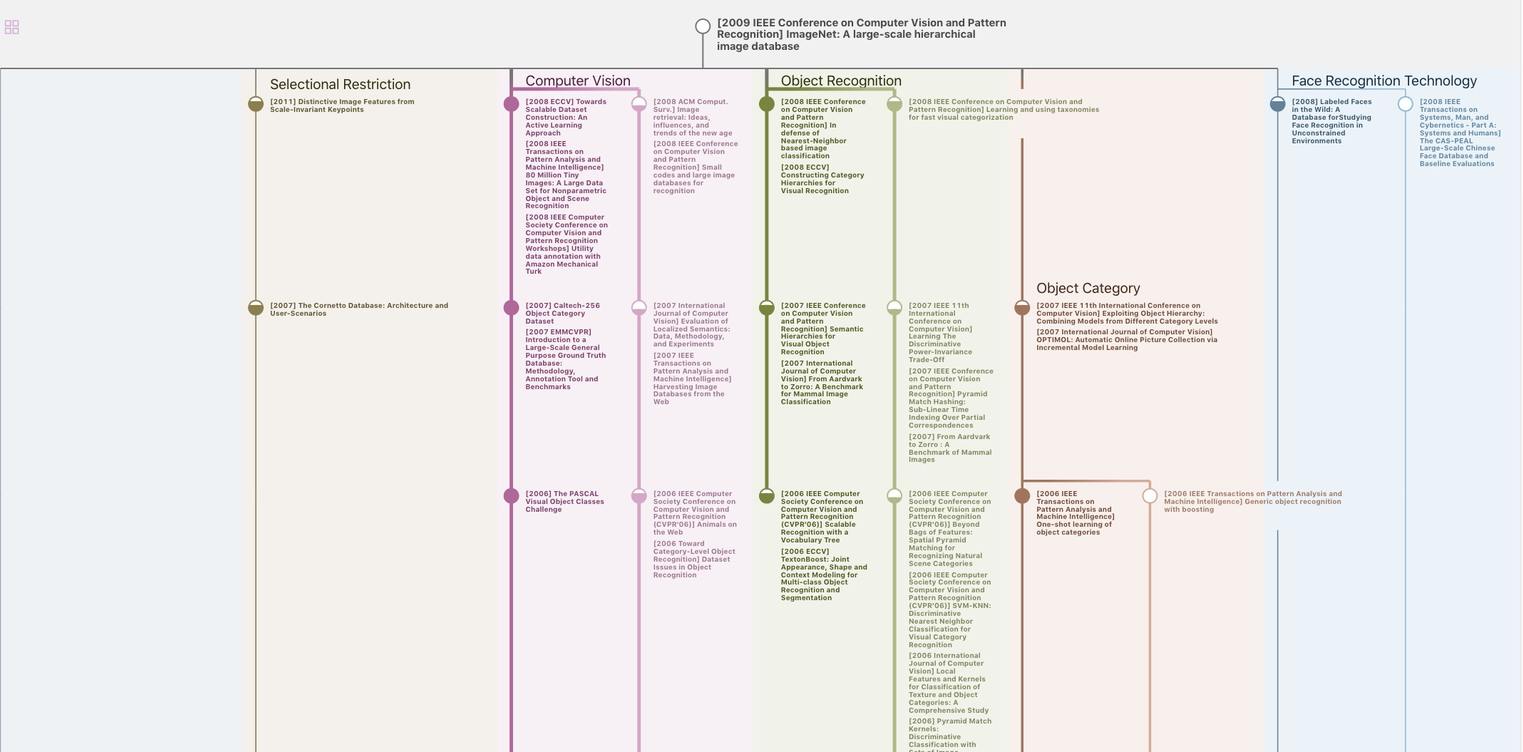
生成溯源树,研究论文发展脉络
Chat Paper
正在生成论文摘要