An Effective LSTM-DDPM Scheme for Energy Theft Detection and Forecasting in Smart Grid
CoRR(2023)
摘要
Energy theft detection (ETD) and energy consumption forecasting (ECF) are two interconnected challenges in smart grid systems. Addressing these issues collectively is crucial for ensuring system security. This paper addresses the interconnected challenges of ETD and ECF in smart grid systems. The proposed solution combines long short-term memory (LSTM) and a denoising diffusion probabilistic model (DDPM) to generate input reconstruction and forecasting. By leveraging the reconstruction and forecasting errors, the system identifies instances of energy theft, with the methods based on reconstruction error and forecasting error complementing each other in detecting different types of attacks. Through extensive experiments on real-world and synthetic datasets, the proposed scheme outperforms baseline methods in ETD and ECF problems. The ensemble method significantly enhances ETD performance, accurately detecting energy theft attacks that baseline methods fail to detect. The research offers a comprehensive and effective solution for addressing ETD and ECF challenges, demonstrating promising results and improved security in smart grid systems.
更多查看译文
关键词
energy theft detection,forecasting
AI 理解论文
溯源树
样例
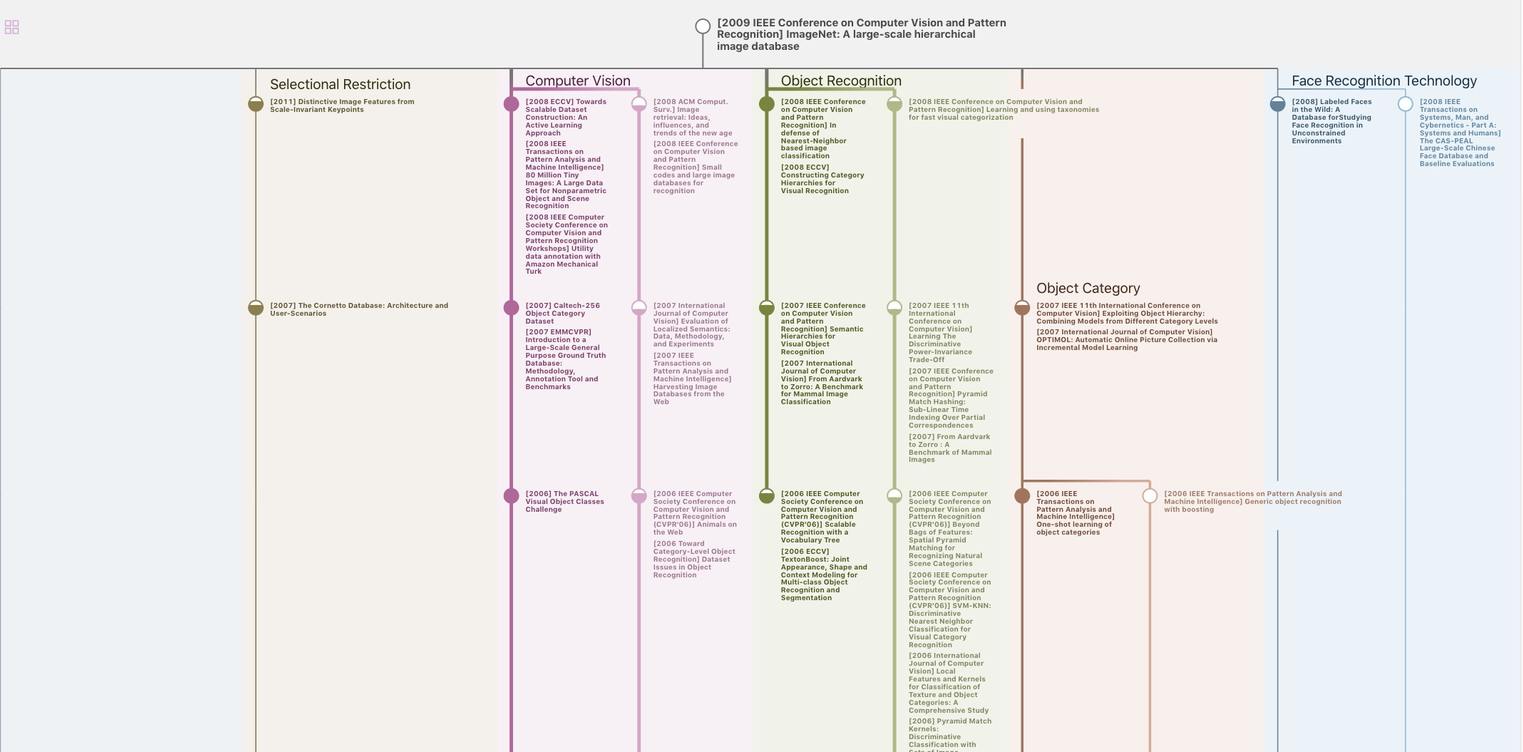
生成溯源树,研究论文发展脉络
Chat Paper
正在生成论文摘要