A Theory for Emergence of Complex Skills in Language Models
CoRR(2023)
摘要
A major driver of AI products today is the fact that new skills emerge in language models when their parameter set and training corpora are scaled up. This phenomenon is poorly understood, and a mechanistic explanation via mathematical analysis of gradient-based training seems difficult. The current paper takes a different approach, analysing emergence using the famous (and empirical) Scaling Laws of LLMs and a simple statistical framework. Contributions include: (a) A statistical framework that relates cross-entropy loss of LLMs to competence on the basic skills that underlie language tasks. (b) Mathematical analysis showing that the Scaling Laws imply a strong form of inductive bias that allows the pre-trained model to learn very efficiently. We informally call this {\em slingshot generalization} since naively viewed it appears to give competence levels at skills that violate usual generalization theory. (c) A key example of slingshot generalization, that competence at executing tasks involving $k$-tuples of skills emerges essentially at the same scaling and same rate as competence on the elementary skills themselves.
更多查看译文
关键词
complex skills,emergence,language,models
AI 理解论文
溯源树
样例
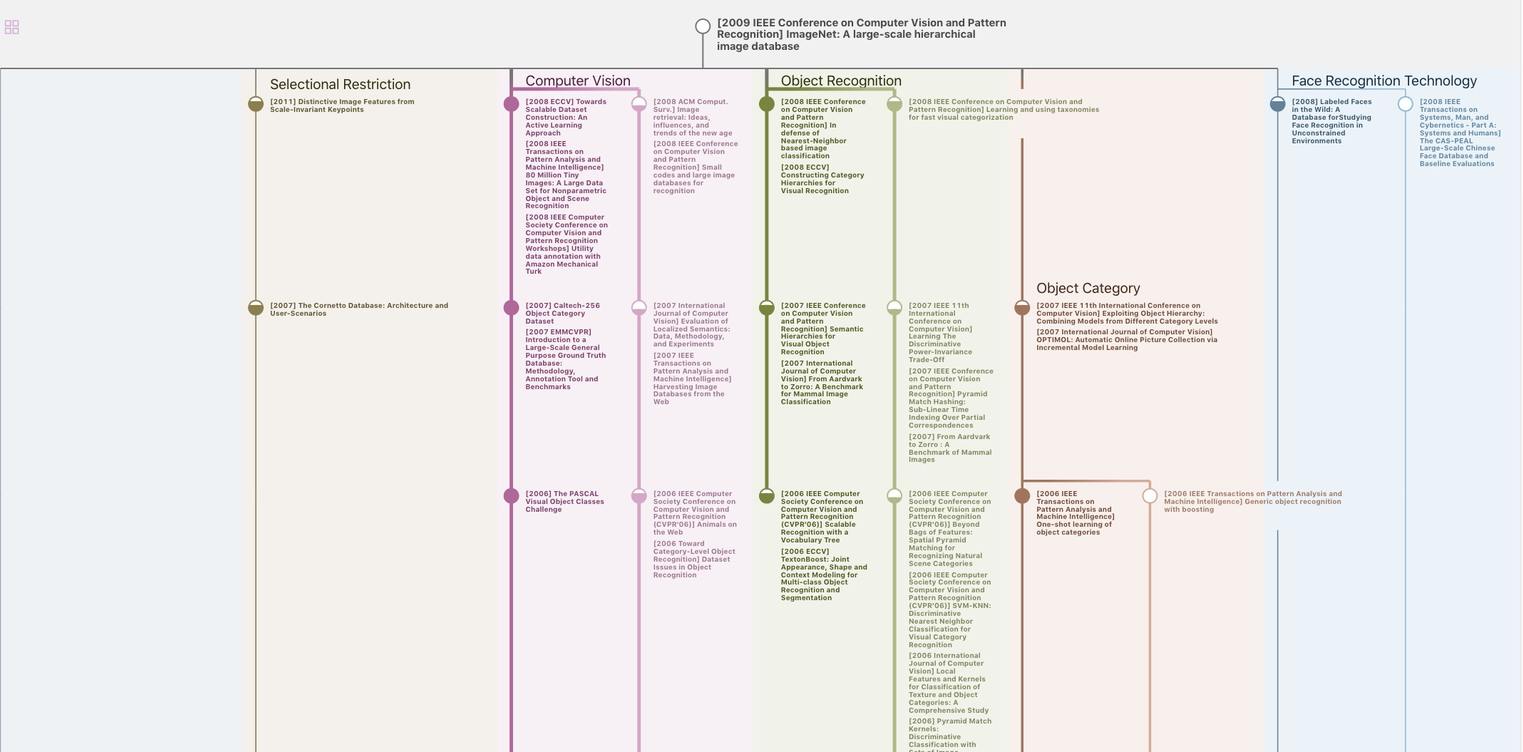
生成溯源树,研究论文发展脉络
Chat Paper
正在生成论文摘要