Multi-growth stage plant recognition: A case study of Palmer amaranth (Amaranthus palmeri) in cotton (Gossypium hirsutum)
COMPUTERS AND ELECTRONICS IN AGRICULTURE(2024)
摘要
Many advanced image -based precision agricultural technologies for plant breeding, field crop research, and sitespecific crop management hinge on the reliable detection and phenotyping of plants across highly variable morphological growth stages. Convolutional neural networks (CNNs) have shown promise for image -based plant phenotyping and weed recognition, but the ability to recognize growth stages, often with stark differences in appearance, is uncertain. Palmer amaranth (Amaranthus palmeri) is a particularly challenging weed plant in cotton (Gossypium hirsutum) production; due to high genetic diversity, it exhibits highly variable plant morphology across growth stages over a growing season, as well as between plants at a given growth stage. This paper investigates eight -class growth stage recognition of A. palmeri in cotton as a challenging detection case study for You Only Look Once (YOLO) architectures. In total, 26 architecture variants from YOLO v3, v5, v6, v6 3.0, v7, and v8 are compared on an eight -class growth stage dataset of A. palmeri. The highest mean average precision (mAP@[0.5:0.951) for recognition of all growth stage classes was 47.34% achieved by v8 -X, with interclass confusion across visually similar classes. With all growth stages grouped as a single class, performance increased, with a maximum mAP@[0.5:0.951 of 67.05% achieved by v7 -Original. Single -class recall of up to 81.42% was achieved by v5 -X, and precision of up to 89.72% was achieved by v8 -X. Class activation maps (CAM) were used to understand model attention on the complex dataset. Fewer classes, grouped by visual or size features improved performance over the ground -truth eight -class dataset. Successful growth stage detection highlights the substantial opportunity for improving plant phenotyping and weed recognition technologies with open -source object detection architectures. Additionally, the first open -access, benchmarking growth stage dataset 'Palmer amaranth growth stages - 8 (PAGS8)' is presented, which is available here: https://weed-ai.sydne y.edu.au/datasets/5c78d067-8750-4803-9cbe-57df8fae55e4.
更多查看译文
关键词
High throughput phenotyping,Deep learning,Precision weed management,Computer vision,Open-access dataset Benchmark
AI 理解论文
溯源树
样例
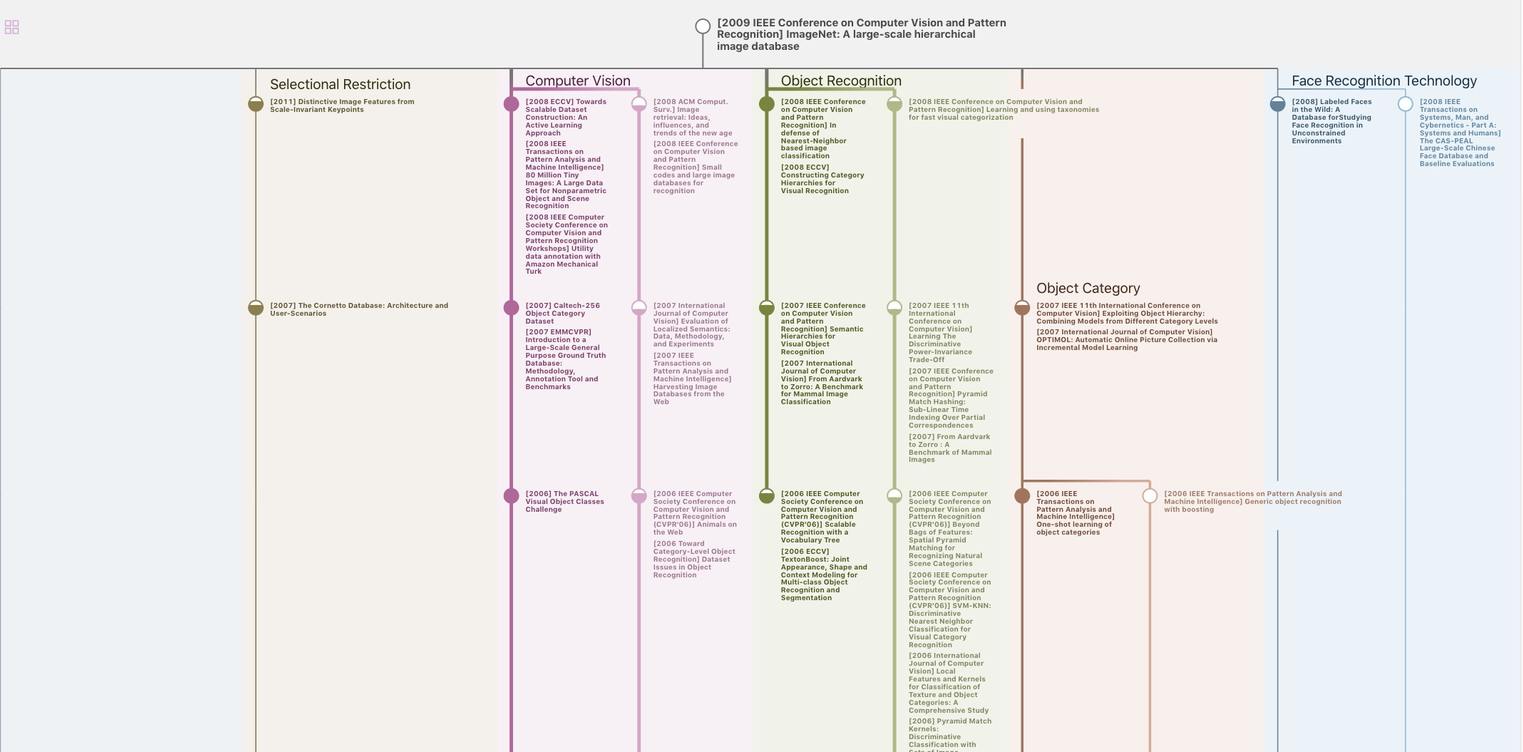
生成溯源树,研究论文发展脉络
Chat Paper
正在生成论文摘要