Data Quality Matters: A Case Study of Obsolete Comment Detection.
ICSE(2023)
摘要
Machine learning methods have achieved great success in many software engineering tasks. However, as a data-driven paradigm, how would the data quality impact the effectiveness of these methods remains largely unexplored. In this paper, we explore this problem under the context of just-in-time obsolete comment detection. Specifically, we first conduct data cleaning on the existing benchmark dataset, and empirically observe that with only 0.22% label corrections and even 15.0% fewer data, the existing obsolete comment detection approaches can achieve up to 10.7% relative accuracy improvement. To further mitigate the data quality issues, we propose an adversarial learning framework to simultaneously estimate the data quality and make the final predictions. Experimental evaluations show that this adversarial learning framework can further improve the relative accuracy by up to 18.1% compared to the state-of-the-art method. Although our current results are from the obsolete comment detection problem, we believe that the proposed two-phase solution, which handles the data quality issues through both the data aspect and the algorithm aspect, is also generalizable and applicable to other machine learning based software engineering tasks.
更多查看译文
关键词
Obsolete comment detection,machine learning for software engineering,data quality
AI 理解论文
溯源树
样例
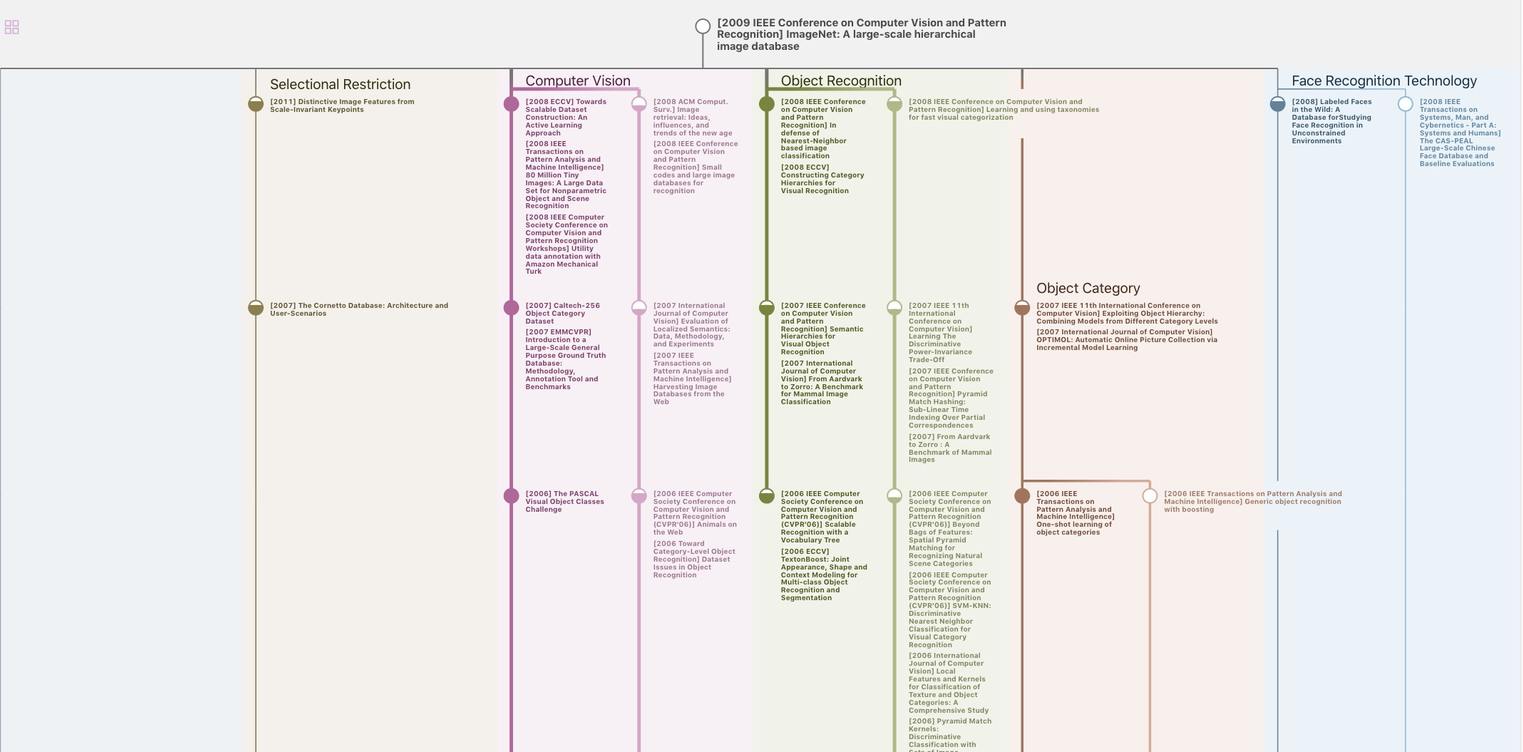
生成溯源树,研究论文发展脉络
Chat Paper
正在生成论文摘要