Solving Job-Shop Scheduling Problem via Deep Reinforcement Learning with Attention Model.
IEA/AIE (2)(2023)
摘要
The job-shop scheduling problem (JSP), as an NP-hard problem, is widely applied in real-world production scheduling problems, such as assembly plants, chemical production, and semiconductor production. Although some deep reinforcement learning (DRL) methods for scheduling problems are recently developed, these methods are limited to tackling various-size problems. For this purpose, we propose a DRL method with an attention model to solve JSP. We formulate JSP as a Markovian decision process and design a size-agnostic policy network based on an attention model. Experimental results show that our method can automatically produce a dispatching scheme for various-size JSP without any expert knowledge, which surpasses traditional priority dispatching rules and the state-of-the-art DRL method.
更多查看译文
关键词
scheduling,deep reinforcement learning,attention model,job-shop
AI 理解论文
溯源树
样例
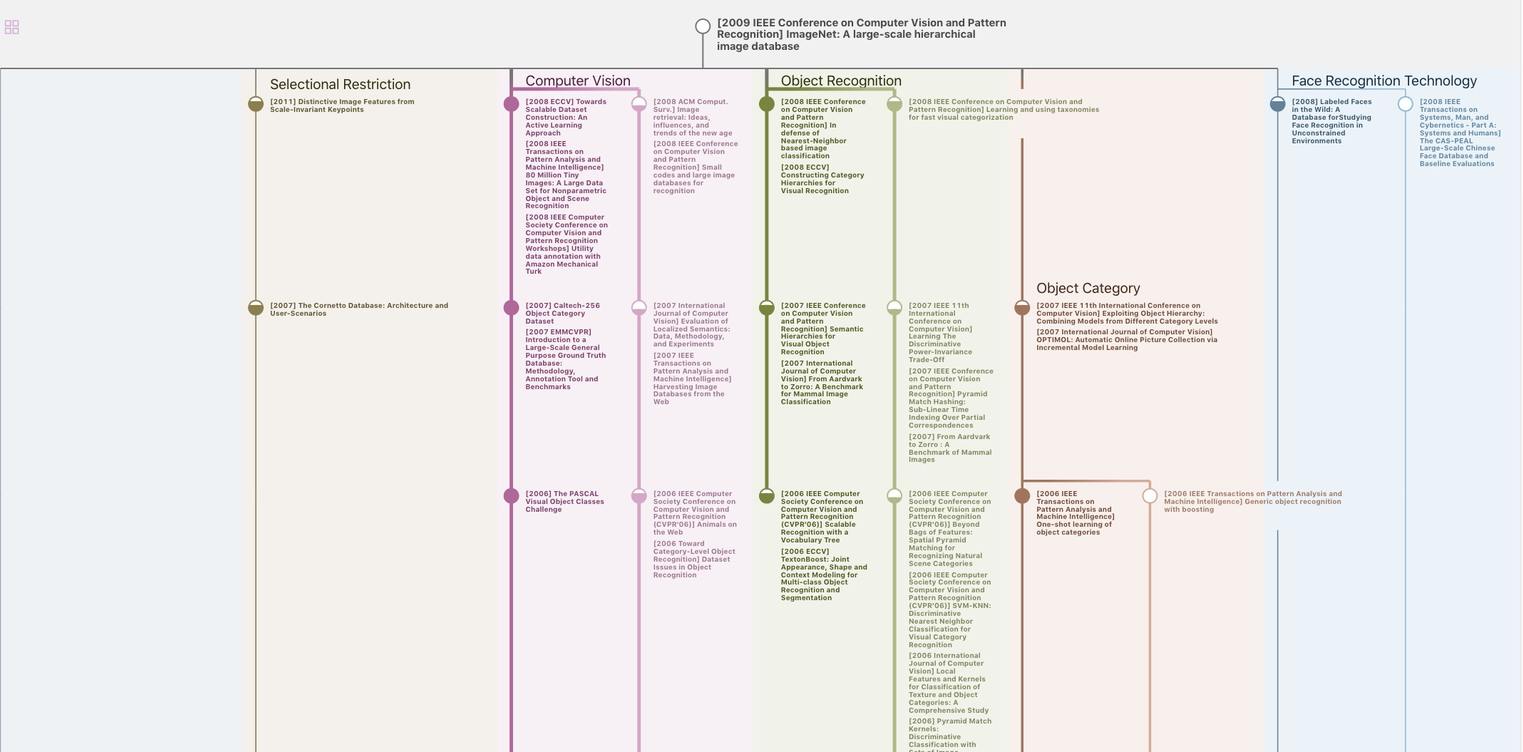
生成溯源树,研究论文发展脉络
Chat Paper
正在生成论文摘要