A Contrastive Method for Continual Generalized Zero-Shot Learning.
IEA/AIE (1)(2023)
摘要
Generalized zero-shot learning (GZSL) aims to train a model that can classify seen and unseen samples based on semantic information. Continual learning, as one of the factors distinguishing artificial intelligence, has received more and more attention in recent years. In this paper, we propose a deep learning model based on a conditional generative model and a contrastive learning framework that can continuously learn from incoming data. In the training phase, knowledge distillation and generative replay mechanisms are used to accumulate past knowledge. In the testing phase, the test samples are mapped to the embedding space to perform generalized zero-shot classification task. Our model not only does not require additional buffers to store data so that it will not cause data leakage problems, but also through experiments show that our model can achieve state-of-the-art results on three benchmark datasets. The source code of the proposed model is available at: https://github.com/liangchen976/CCGZSL.
更多查看译文
关键词
learning,contrastive method,zero-shot
AI 理解论文
溯源树
样例
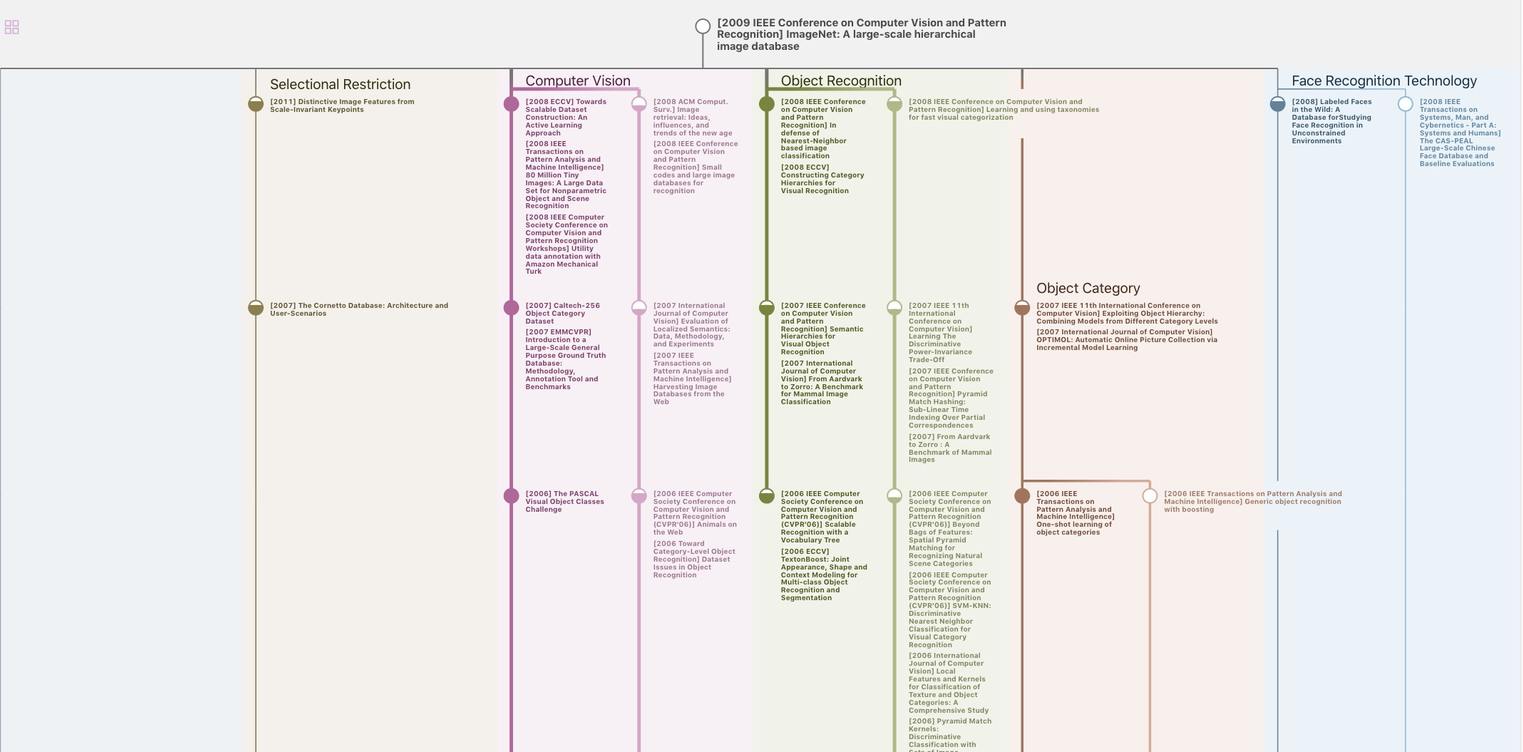
生成溯源树,研究论文发展脉络
Chat Paper
正在生成论文摘要