Uncertainty and Lack of Information Affecting the Training of Machine Learning Algorithms for Fault Prediction of Cable-Joints
I2MTC(2023)
摘要
Artificial intelligence (AI) and machine learning algorithms are becoming more and more popular in power system applications. Their benefits have been proven in many different fields, and power systems will benefit from them too. For example, AI allows the analysis of huge amounts of data which could not be treated otherwise. Furthermore, well-trained algorithms may automatize operations which typically need a dedicated operator. A limit to the implementation of AI in power systems is the uncertainty. It can be divided into three main contributions: the uncertainty of the algorithms results, the uncertainty of the input data, and the lack of experience, information, or unique solutions associated to a specific application. Therefore, this paper aims to include the latter two uncertainty contributions into machine learning algorithms. Their output is used for the fault prediction of medium voltage cable joints, providing information about their health status. As a matter of fact, it is still not possible to exactly predict the cable joint fault. Therefore, such an application is a significant case study to address the uncertainty contributions affecting the training of machine learning algorithms. The obtained results clearly demonstrate the effect of the sources of uncertainty on the algorithm performance.
更多查看译文
关键词
uncertainty, precision, machine learning, cablejoint, fault prediction
AI 理解论文
溯源树
样例
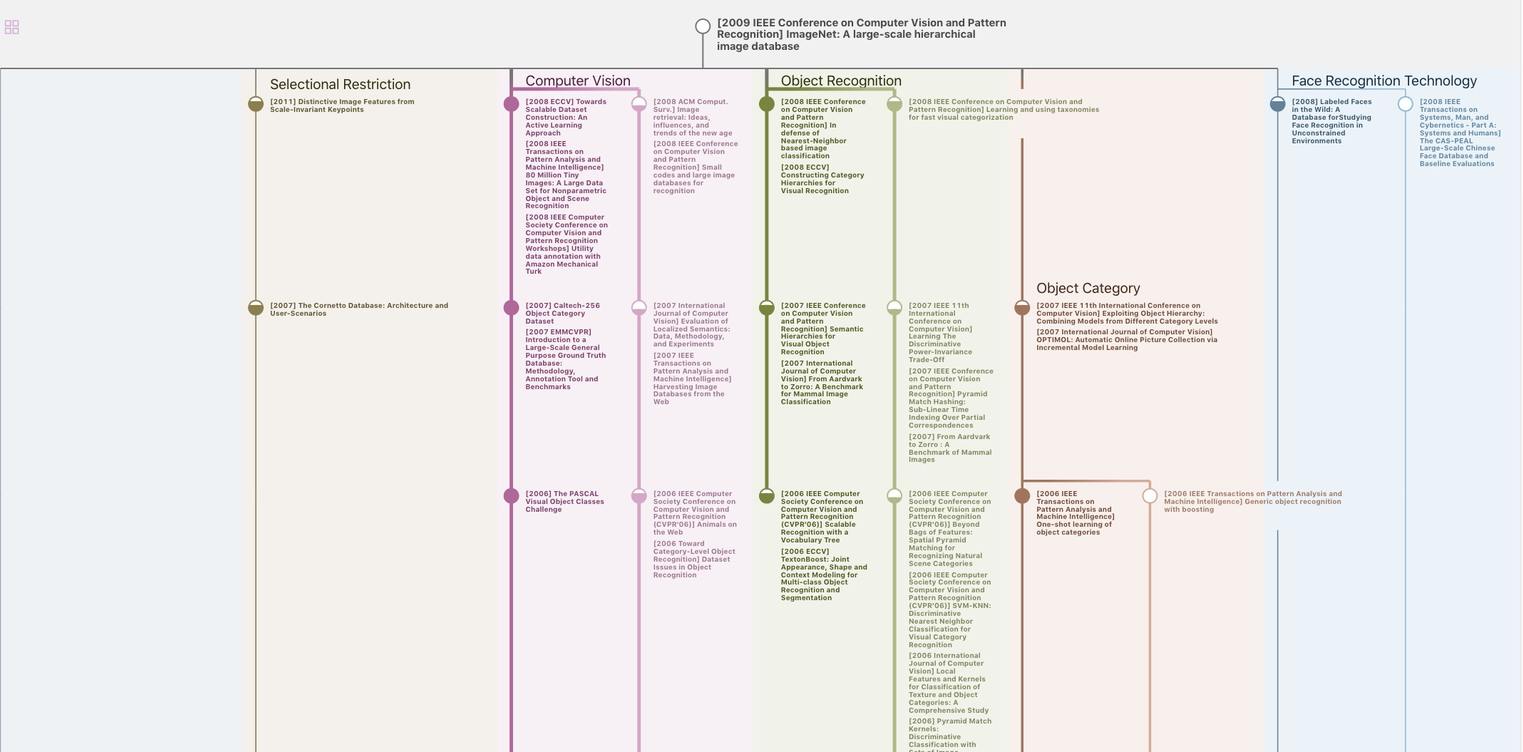
生成溯源树,研究论文发展脉络
Chat Paper
正在生成论文摘要