EEG-Based Subject-Independent Depression Detection Using Dynamic Convolution and Feature Adaptation.
ICSI (2)(2023)
摘要
Depression is a debilitating condition that can seriously impact quality of life, and existing clinical diagnoses are often complicated and dependent on physician experience. Recently, research on EEG-based major depressive disorder (MDD) detection has achieved good performance. However, subject-independent depression detection (i.e., diagnosis of a person never met) remains challenging due to large inter-subject discrepancies in EEG signal distribution. To address this, we propose an EEG-based depression detection model (DCAAN) that incorporates dynamic convolution, adversarial domain adaptation, and association domain adaptation. Dynamic convolution is introduced in the feature extractor to enhance model expression capability. Furthermore, to generalize the model across subjects, adversarial domain adaptation is used to achieve marginal distribution domain adaptation and association domain adaptation is used to achieve conditional distribution domain adaptation. Based on experimentation, our model achieved 86.85% accuracy in subject-independent MDD detection using the multimodal open mental disorder analysis (MODMA) dataset, confirming the considerable potential of the proposed method.
更多查看译文
关键词
depression,dynamic convolution,eeg-based,subject-independent
AI 理解论文
溯源树
样例
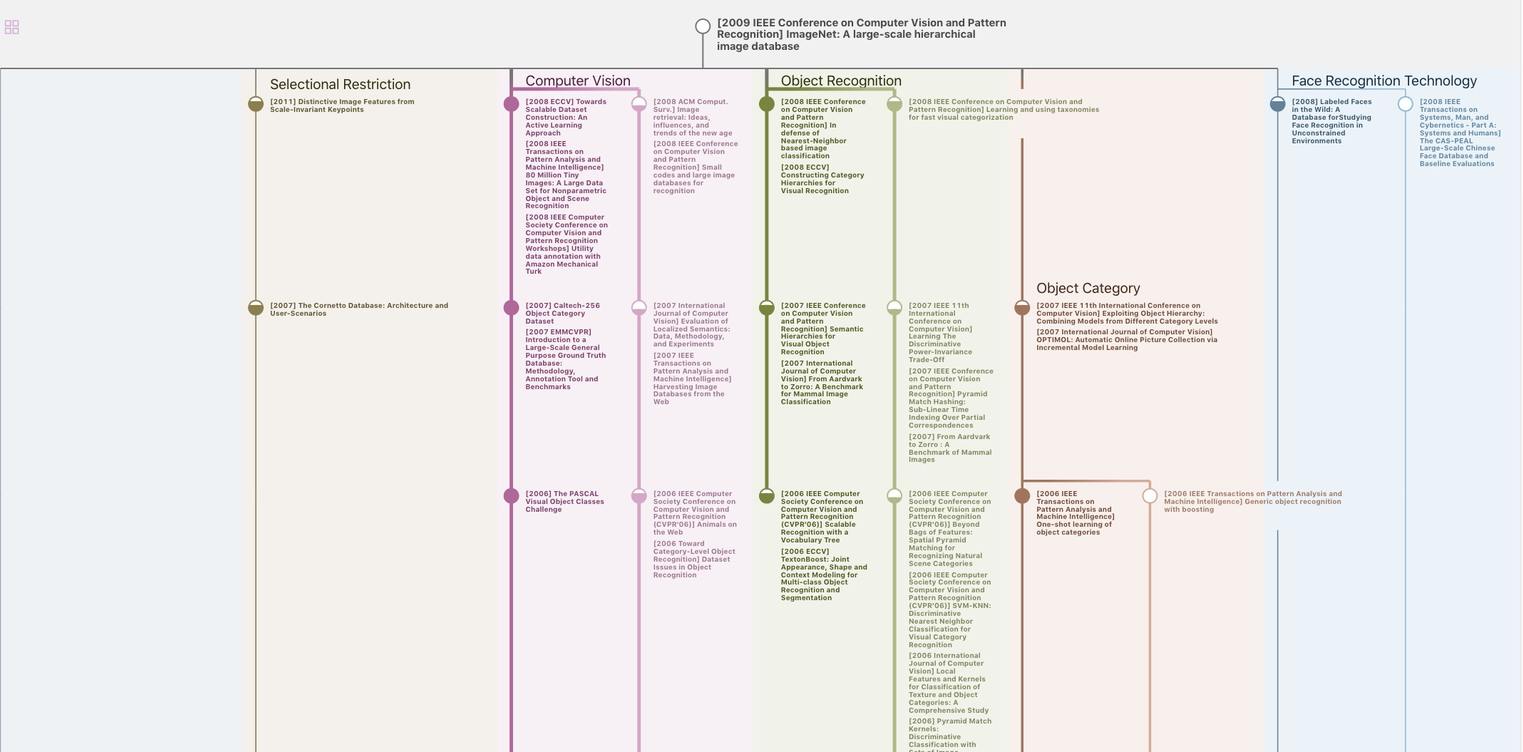
生成溯源树,研究论文发展脉络
Chat Paper
正在生成论文摘要