Evaluating Fairness Metrics.
BIAS(2023)
摘要
Artificial Intelligence systems add significant value to decision-making. However, the systems must be fair because bias creeps into the system from sources like data and preprocessing algorithms. In this work, we explore fairness metrics discussing the shortfalls and benefits of each metric. The fairness metrics are demographic, statistical, and game theoretic. We find that the demographic fairness metrics are independent of the actual target value and hence have limited use. In contrast, the statistical fairness metrics can provide the thresholds to maximize fairness. The Minimax criterion was used to guide the search and help recommend the best model where the error among protected groups was minimum.
更多查看译文
关键词
fairness metrics
AI 理解论文
溯源树
样例
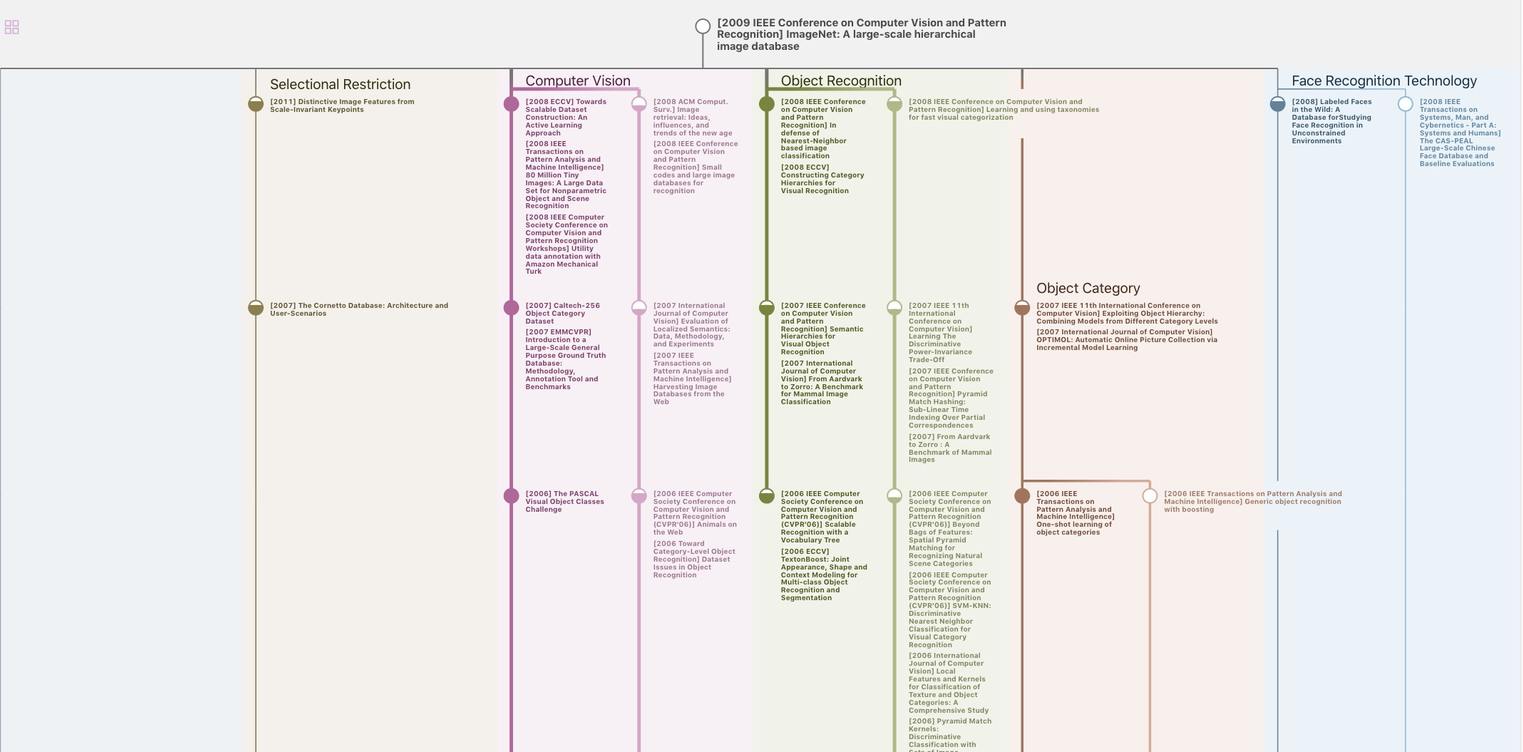
生成溯源树,研究论文发展脉络
Chat Paper
正在生成论文摘要