Semi-supervised Multi-structure Segmentation in Chest X-Ray Imaging
2023 IEEE 36TH INTERNATIONAL SYMPOSIUM ON COMPUTER-BASED MEDICAL SYSTEMS, CBMS(2023)
摘要
The importance of X-Ray imaging analysis is paramount for healthcare institutions since it is the main imaging modality for patient diagnosis, and deep learning can be used to aid clinicians in image diagnosis or structure segmentation. In recent years, several articles demonstrate the capability that deep learning models have in classifying and segmenting chest x-ray images if trained in an annotated dataset. Unfortunately, for segmentation tasks, only a few relatively small datasets have annotations, which poses a problem for the training of robust deep learning strategies. In this work, a semi-supervised approach is developed which consists of using available information regarding other anatomical structures to guide the segmentation when the groundtruth segmentation for a given structure is not available. This semi-supervised is compared with a fully-supervised approach for the tasks of lung segmentation and for multi-structure segmentation (lungs, heart and clavicles) in chest x-ray images. The semi-supervised lung predictions are evaluated visually and show relevant improvements, therefore this approach could be used to improve performance in external datasets with missing groundtruth. The multi-structure predictions show an improvement in mean absolute and Hausdorff distances when compared to a fully supervised approach and visual analysis of the segmentations shows that false positive predictions are removed. In conclusion, the developed method results in a new strategy that can help solve the problem of missing annotations and increase the quality of predictions in new datasets.
更多查看译文
关键词
multi-structure semantic segmentation,chest radiography,deep learning,semi-supervised
AI 理解论文
溯源树
样例
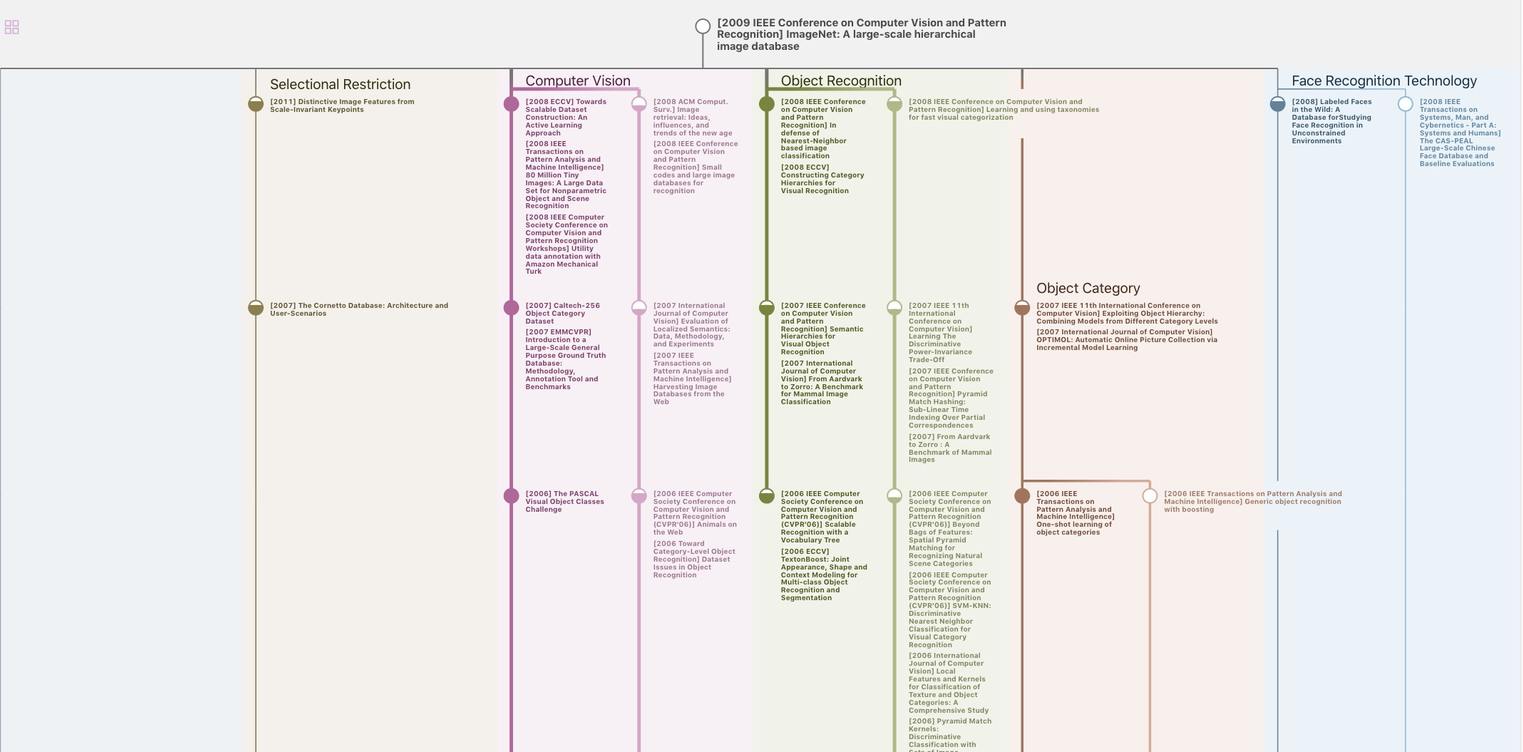
生成溯源树,研究论文发展脉络
Chat Paper
正在生成论文摘要