Degree-Normalization Improves Random-Walk-Based Embedding Accuracy in PPI Graphs.
IWBBIO (2)(2023)
摘要
Among the many proposed solutions in graph embedding, traditional random walk-based embedding methods have shown their promise in several fields. However, when the graph contains high-degree nodes, random walks often neglect low- or middle-degree nodes and tend to prefer stepping through high-degree ones instead. This results in random-walk samples providing a very accurate topological representation of neighbourhoods surrounding high-degree nodes, which contrasts with a coarse-grained representation of neighbourhoods surrounding middle and low-degree nodes. This in turn affects the performance of the subsequent predictive models, which tend to overfit high-degree nodes and/or edges having high-degree nodes as one of the vertices. We propose a solution to this problem, which relies on a degree normalization approach. Experiments with popular RW-based embedding methods applied to edge prediction problems involving eight protein-protein interaction (PPI) graphs from the STRING database show the effectiveness of the proposed approach: degree normalization not only improves predictions but also provides more stable results, suggesting that our proposal has a regularization effect leading to a more robust convergence.
更多查看译文
关键词
graphs,degree-normalization,random-walk-based
AI 理解论文
溯源树
样例
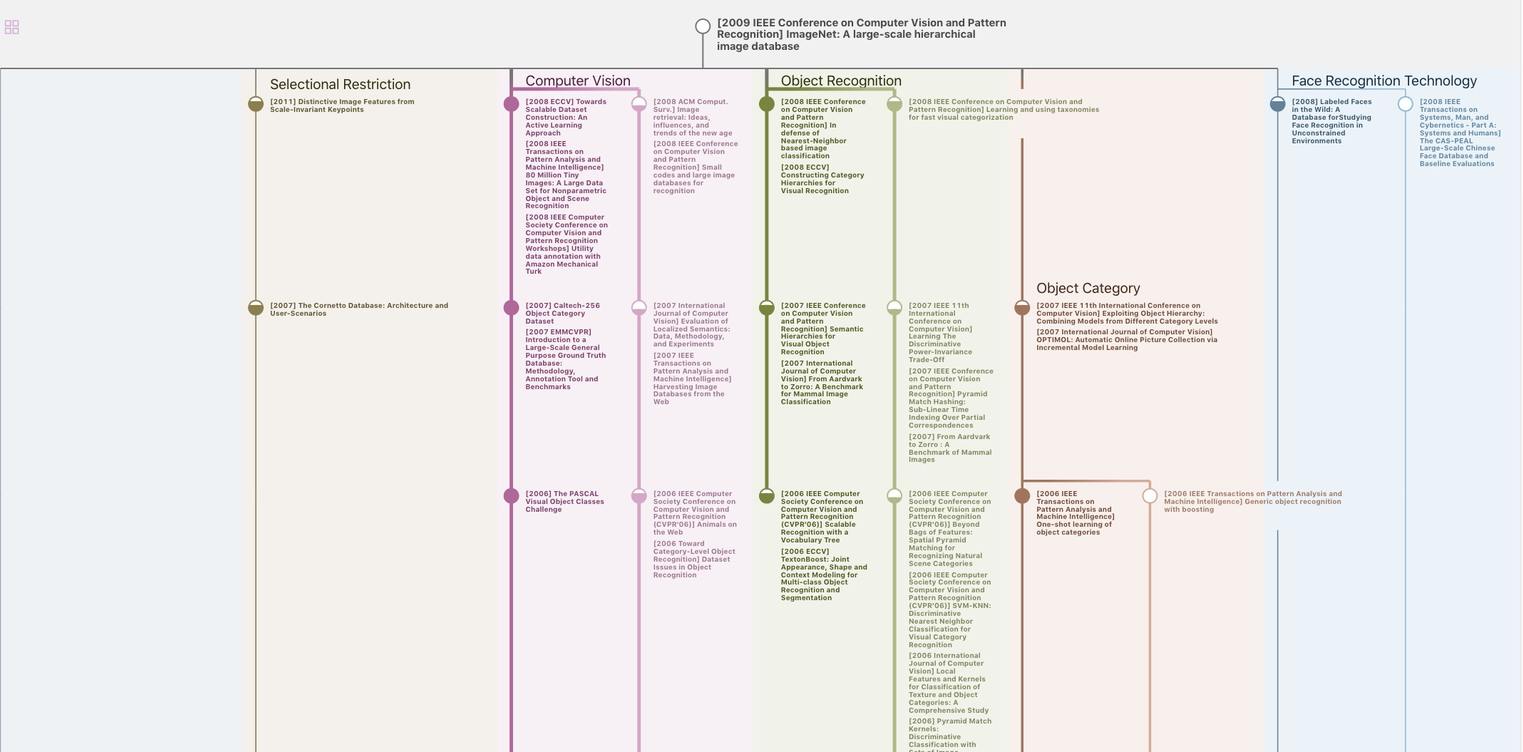
生成溯源树,研究论文发展脉络
Chat Paper
正在生成论文摘要