Enhancing Neonatal Pain Assessment Transparency via Explanatory Training Examples Identification
2023 IEEE 36TH INTERNATIONAL SYMPOSIUM ON COMPUTER-BASED MEDICAL SYSTEMS, CBMS(2023)
摘要
Deep Learning (DL)-based solutions have shown promising performance in assessing neonatal pain. However, the occlusion of the visual modality (face and body) is common in clinical settings due to several factors, including a prone sleeping position, low light, or swaddling. In such scenarios, other pain signals, such as audio signals, can be used as the major behavioral signs of pain. Although DL-based methods are proposed to assess pain from audio, these methods lack transparency and explainability (black box), which can decrease the user's trust in the automated decision. In this work, we visualize the neonate's audio signal as a spectrogram image to classify it as pain or no pain and present an instance-based approach for explaining the decision of the black-box model. Further, this work provides an analysis of the most helpful and harmful training instances using an influence score followed by assessing their impact on pain prediction. Experimental results demonstrate that the proposed approach can detect and remove harmful instances, eventually leading to a compressed dataset. Our results also show that the proposed work can add explainability to the current DL-based pain detection methods, which can enhance users' trust and provide a viable approach toward pain assessment in clinical settings.
更多查看译文
关键词
Influence Function,Explainability,Neonatal Pain,Deep Neural Networks
AI 理解论文
溯源树
样例
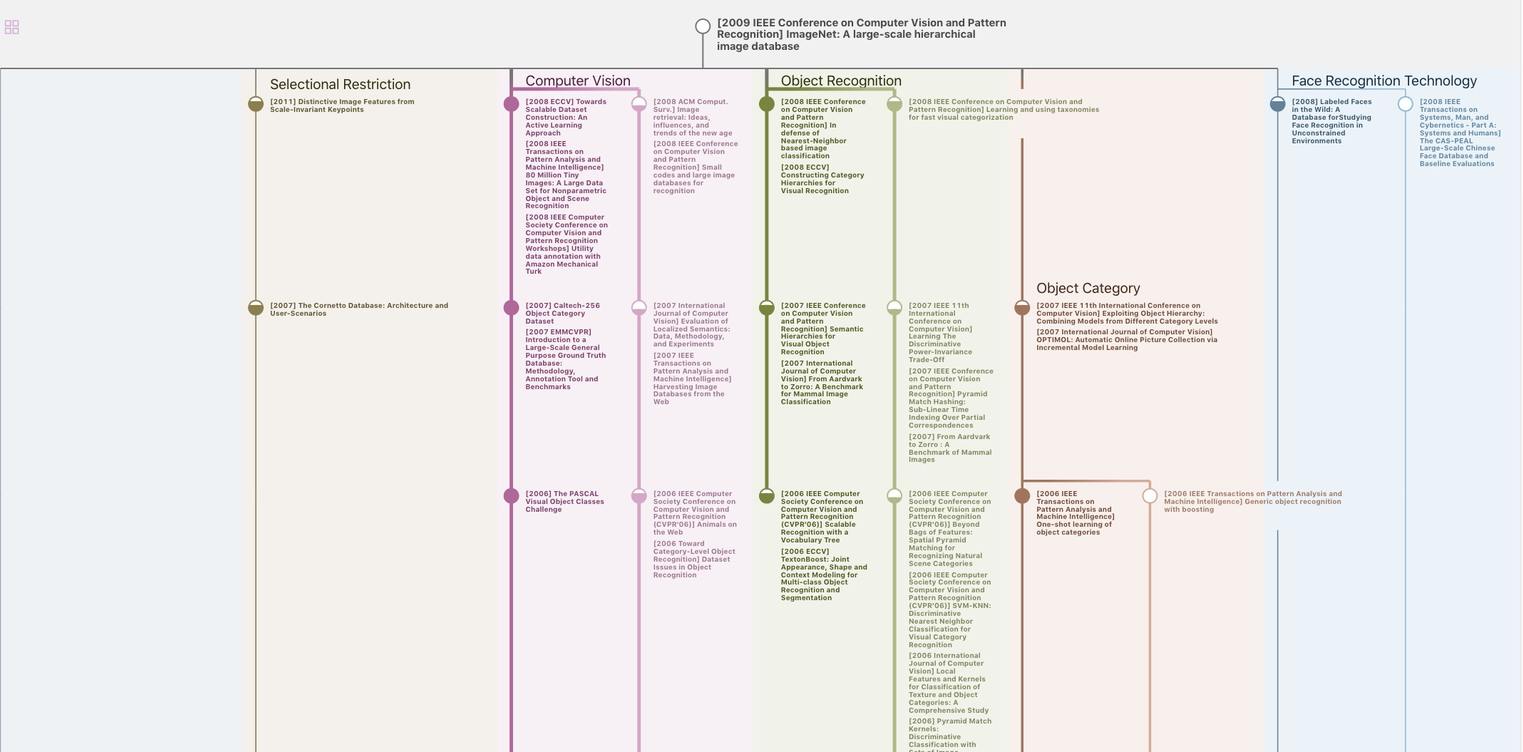
生成溯源树,研究论文发展脉络
Chat Paper
正在生成论文摘要