Privacy Assessment of Synthetic Patient Data
2023 IEEE 36TH INTERNATIONAL SYMPOSIUM ON COMPUTER-BASED MEDICAL SYSTEMS, CBMS(2023)
摘要
In this paper, we quantify the privacy gain of synthetic patient data drawn from two generative models, MST and PrivBayes, which is based on real anonymized primary care patient data. This evaluation is implemented for two types of inference attacks, namely membership and attribute inference attacks using a new toolbox, TAPAS. The aim is to quantitatively evaluate the privacy gain of each attack where these two differentially private generators and different threat models are used with a focus on black-box knowledge. The evaluation that was carried out in this paper demonstrates that vulnerabilities of synthetic patient data depend on the different attack scenarios, threat models, and algorithms used to generate the synthetic patient data. It was shown empirically that although the synthetic patient data achieved high privacy gain in most attack scenarios, it does not behave uniformly against adversarial attacks, and some records and outliers remain vulnerable depending on the attack scenario. Moreover, it was shown that the PrivBayes generator is the more robust generator in comparison to MST in terms of the privacy-preservation of synthetic data.
更多查看译文
关键词
synthetic data,adversarial attacks,privacy auditing,generative models
AI 理解论文
溯源树
样例
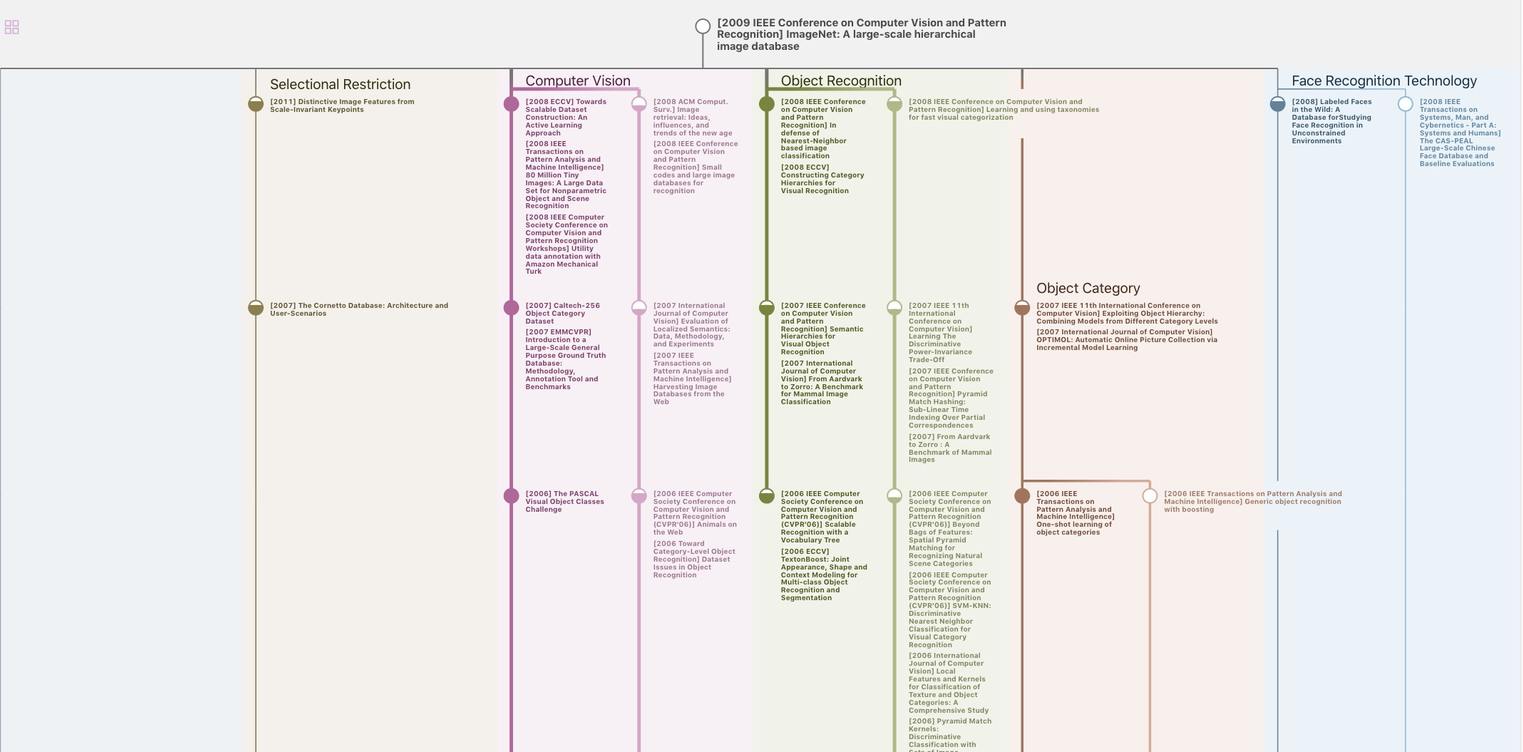
生成溯源树,研究论文发展脉络
Chat Paper
正在生成论文摘要