A Hierarchical Approach to Anomalous Subgroup Discovery.
ICDE(2023)
摘要
Understanding peculiar and anomalous behavior of machine learning models for specific data subgroups is a fundamental building block of model performance and fairness evaluation. The analysis of these data subgroups can provide useful insights into model inner working and highlight its potentially discriminatory behavior. Current approaches to subgroup exploration ignore the presence of hierarchies in the data, and can only be applied to discretized attributes. The discretization process required for continuous attributes may significantly affect the identification of relevant subgroups.We propose a hierarchical subgroup exploration technique to identify anomalous subgroup behavior at multiple granularity levels, along with a technique for the hierarchical discretization of data attributes. The hierarchical discretization produces, for each continuous attribute, a hierarchy of intervals. The subsequent hierarchical exploration can exploit data hierarchies, selecting for each attribute the optimal granularity to identify subgroups that are both anomalous, and with enough elements to be statistically and practically significant. Compared to non- hierarchical approaches, we show that our hierarchical approach is more powerful in identifying anomalous subgroups and more stable with respect to discretization and exploration parameters.
更多查看译文
关键词
model evaluation,fairness,subgroup discovery,discretization,generalized itemsets,hierarchies,data slicing
AI 理解论文
溯源树
样例
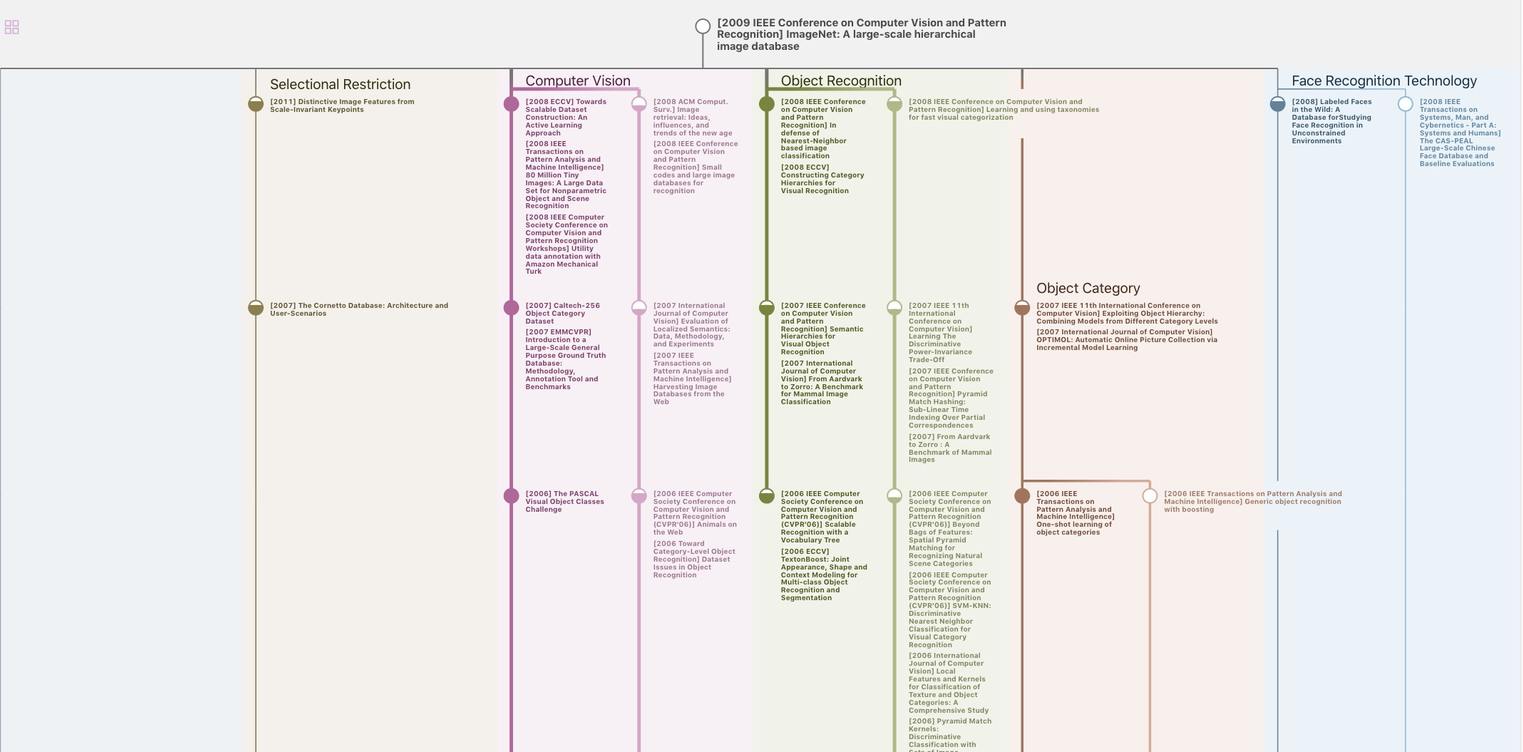
生成溯源树,研究论文发展脉络
Chat Paper
正在生成论文摘要