Unsupervised Feature Learning Architecture with Multi-clustering Integration RBM.
ICDE(2023)
摘要
Feature learning is a crucial phase machine learning [1] – [3] . How to obtain appropriate features distribution without any background is still a hard problem in machine learning. In this paper, we present a novel unsupervised feature learning architecture (see Fig. 1 ), which consists of a multi-clustering integration module and a variant of RBM termed multi-clustering integration RBM (MIRBM). In the multi-clustering integration module, we choose three clusterers to obtain three different global clustering partitions (CPs). Then, an unanimous voting strategy is used to generate the local clustering partition (LCP) of visible layer data. Hence, the LCP only has partial visible layer data. The novel MIRBM model is a core feature encoding part of the proposed unsupervised feature learning architecture. The novelty of it is that the LCP as an unsupervised guidance is integrated into the CD 1 learning to guide the distribution of the hidden layer features. For the instance in the same LCP cluster, the hidden and reconstructed hidden layer features of the MIRBM model in the proposed architecture tend to constrict together in the training process. Meanwhile, each LCP center tends to disperse from each other as much as possible in the hidden and reconstructed hidden layer during training. This work has three main contributions: 1) A novel unsupervised feature learning architecture is proposed, which consists of a multi-clustering integration module and an MIRBM model. 2) In the multi-clustering integration module of the proposed architecture, three unsupervised algorithms are employed to obtain three different global CPs without any background knowledge or label. 3) The MIRBM model in the proposed architecture uses the LCP as an unsupervised guidance to guide the distribution of the hidden layer features by integrating the LCP into the CD 1 learning.
更多查看译文
关键词
appropriate features distribution,CD 1 learning,core feature,different global clustering partitions,hidden layer features,LCP cluster,local clustering partition,machine learning,MIRBM model,multiclustering integration module,multiclustering integration RBM,novel unsupervised feature learning architecture,unsupervised guidance
AI 理解论文
溯源树
样例
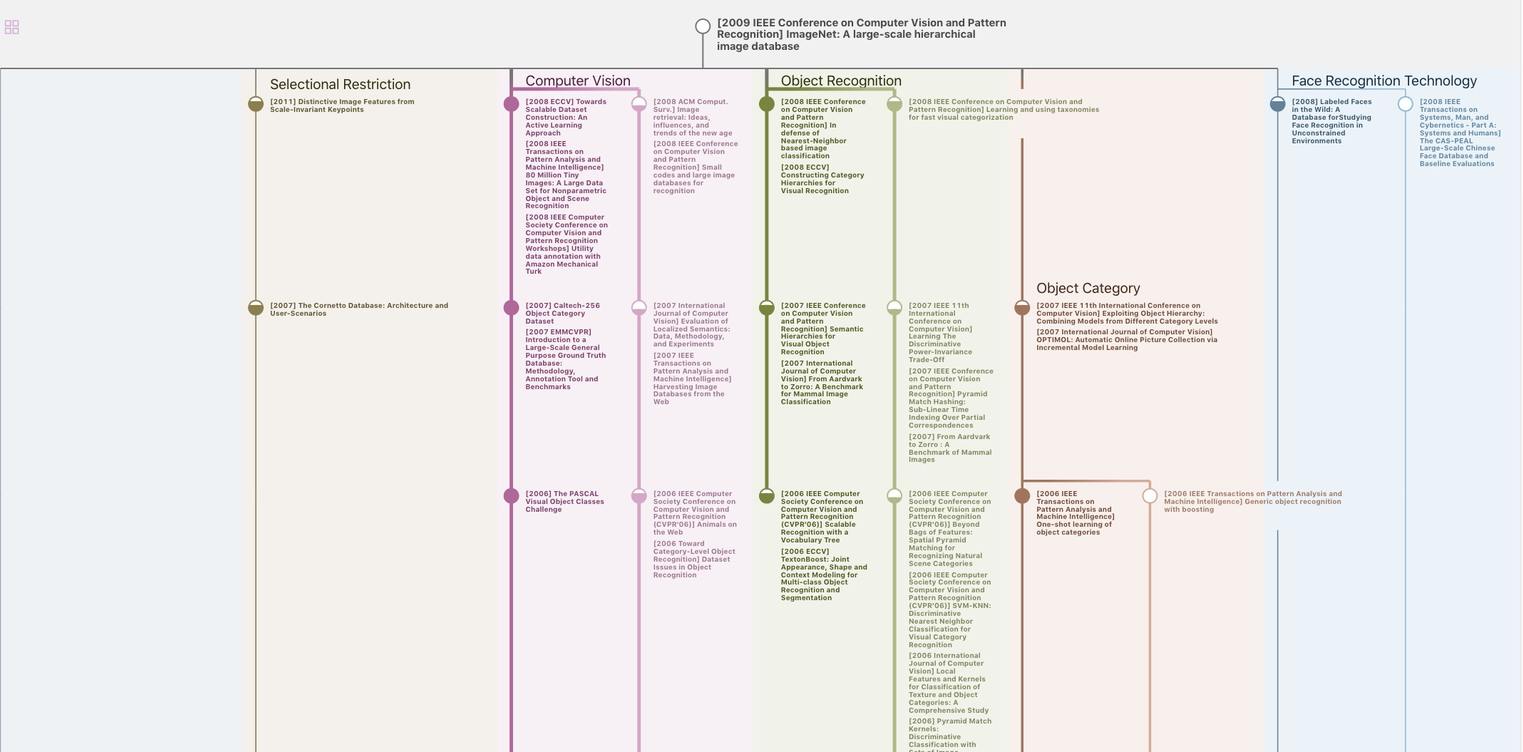
生成溯源树,研究论文发展脉络
Chat Paper
正在生成论文摘要