Empirical Analysis of Full-System Approximation on Non-Spiking and Spiking Neural Networks.
MOCAST(2023)
摘要
Spiking Neural Networks (SNNs) are a promising alternative to conventional neural networks. In particular, Spikeby-Spike (SbS) neural networks offer exceptional noise robustness and reduced complexity. The error resilience of SbS provides a unique opportunity to improve its hardware efficiency with minimal loss of accuracy. Approximate processing is a mechanism that exploits the error resilience of applications to improve hardware cost and efficiency. Most of the previous works have mainly focused on quantization or approximation of a single subsystem, ignoring the potential improvements of simultaneous communication and computation approximation and quantization. This paper, for the first time, investigates the robustness of Convolutional Neural Network (CNN) and SbS inference for a combination of quantization and approximate communication and computation. We have shown that the full system approximation and quantization results in a better accuracy-cost trade-off rather than applying approximation or quantization individually. Furthermore, we observed that the SbS is more robust than the CNN in most cases, and can even improve the accuracy by more than 0.6% compared to an exactly unquantized network.
更多查看译文
关键词
Spiking neural networks,Convolutional neural networks,Approximate processing,Approximate communication,Quantization
AI 理解论文
溯源树
样例
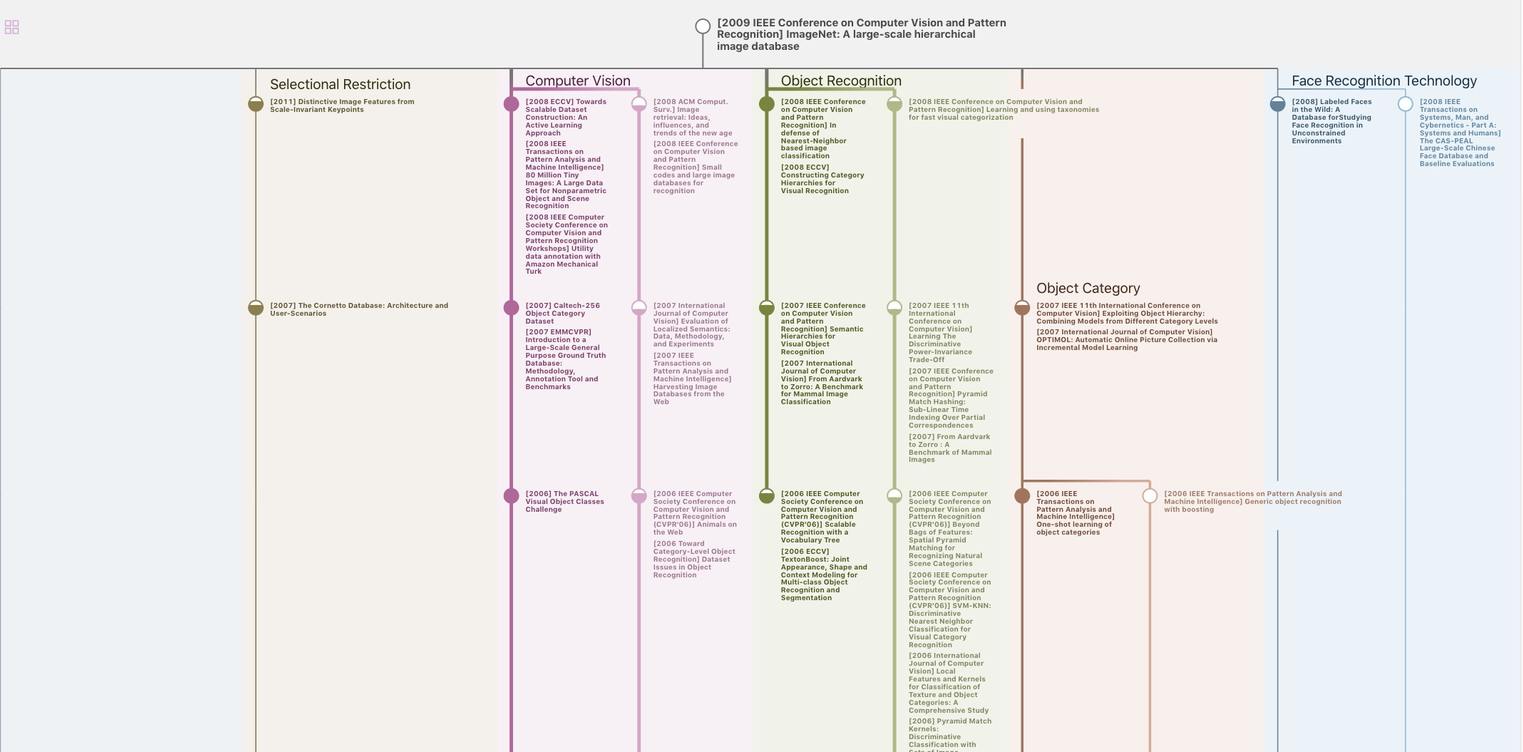
生成溯源树,研究论文发展脉络
Chat Paper
正在生成论文摘要