Model-Agnostic Meta-Learning Techniques: A State-of-The-Art Short Review.
MOCAST(2023)
摘要
In the last few years, novel meta-learning techniques have established a new field of research. Great emphasis is given to few-shot learning approaches, where the model is trained by using only a few training examples. In this work, a review of several model-agnostic meta-learning methodologies (MAML) is presented. Firstly, we identify and discuss the typical characteristics of the first proposed MAML algorithm. Next, we classify the model-agnostic approaches into three main categories: Regular gradient descent MAML, Hessian-free MAML, and Bayesian MALM, by presenting their advantages and limitations. Finally, we conclude this work with further discussion and highlight the future research directions.
更多查看译文
关键词
Model-agnostic meta-learning,meta-learning,deep learning,few-shot learning,one-shot learning
AI 理解论文
溯源树
样例
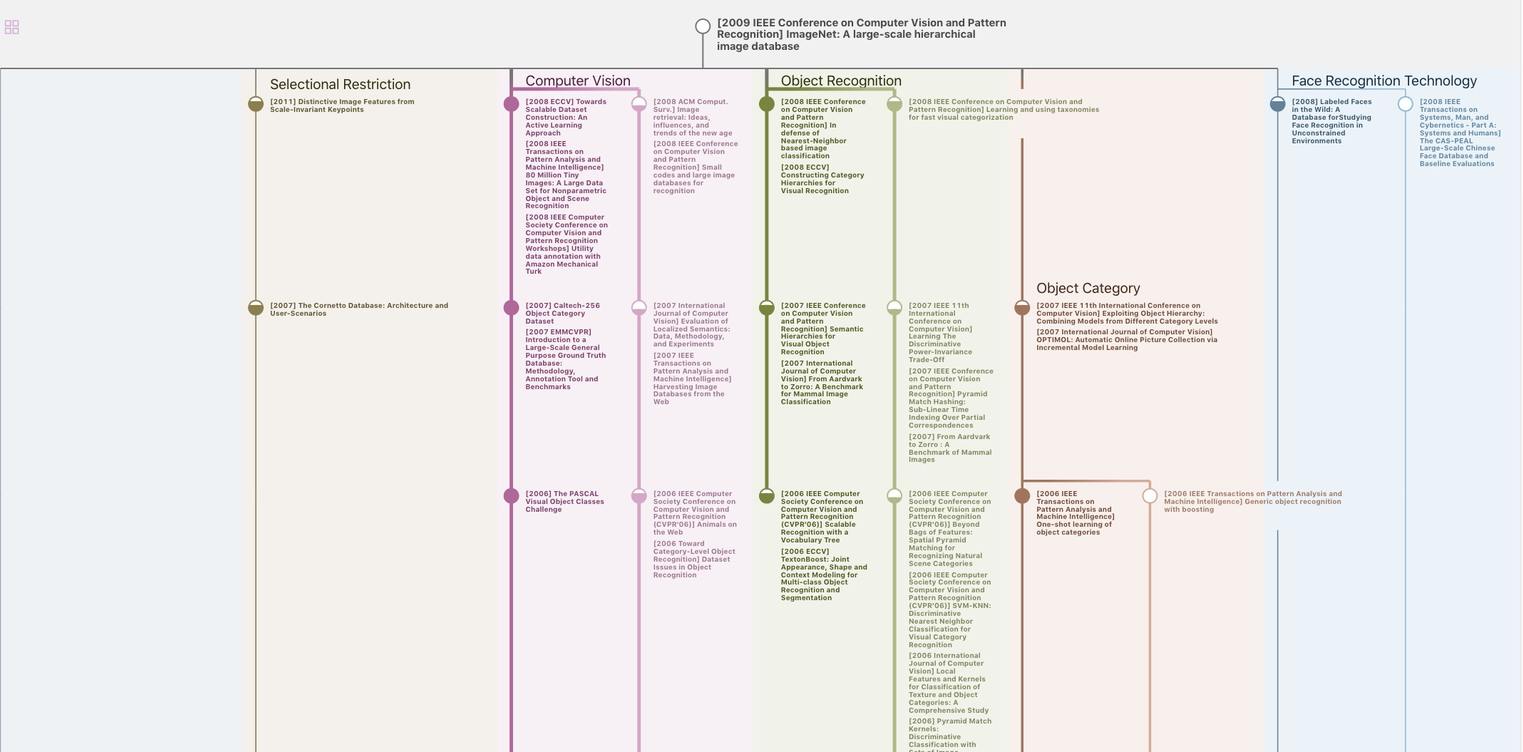
生成溯源树,研究论文发展脉络
Chat Paper
正在生成论文摘要