Graph-Structured Nonlinear Programming: Properties and Algorithms
2023 AMERICAN CONTROL CONFERENCE, ACC(2023)
摘要
A graph-structured nonlinear program (NLP) is a nonlinear optimization problem whose algebraic structure is induced by a graph. These problems arise in diverse applications such as dynamic optimization, network optimization, partial differential equation-constrained optimization, and multi-stage stochastic programming. Building on the NLP sensitivity theory, we show that the nodal solution sensitivity against parametric perturbation decays exponentially in the distance on the graph. Remarkably, this result (exponential decay of sensitivity; EDS) holds under standard regularity assumptions: second-order sufficiency conditions and the linear independence constraint qualification. EDS allows the creation of a novel computing strategy, the overlapping Schwarz method. This method decomposes a graph-structured NLP into multiple smaller subproblems and solves them iteratively with the exchange of information at boundaries. Based on EDS, we prove that the convergence rate of the overlapping Schwarz method for uniformly regular graph-structured NLPs improves exponentially with the size of overlap.
更多查看译文
AI 理解论文
溯源树
样例
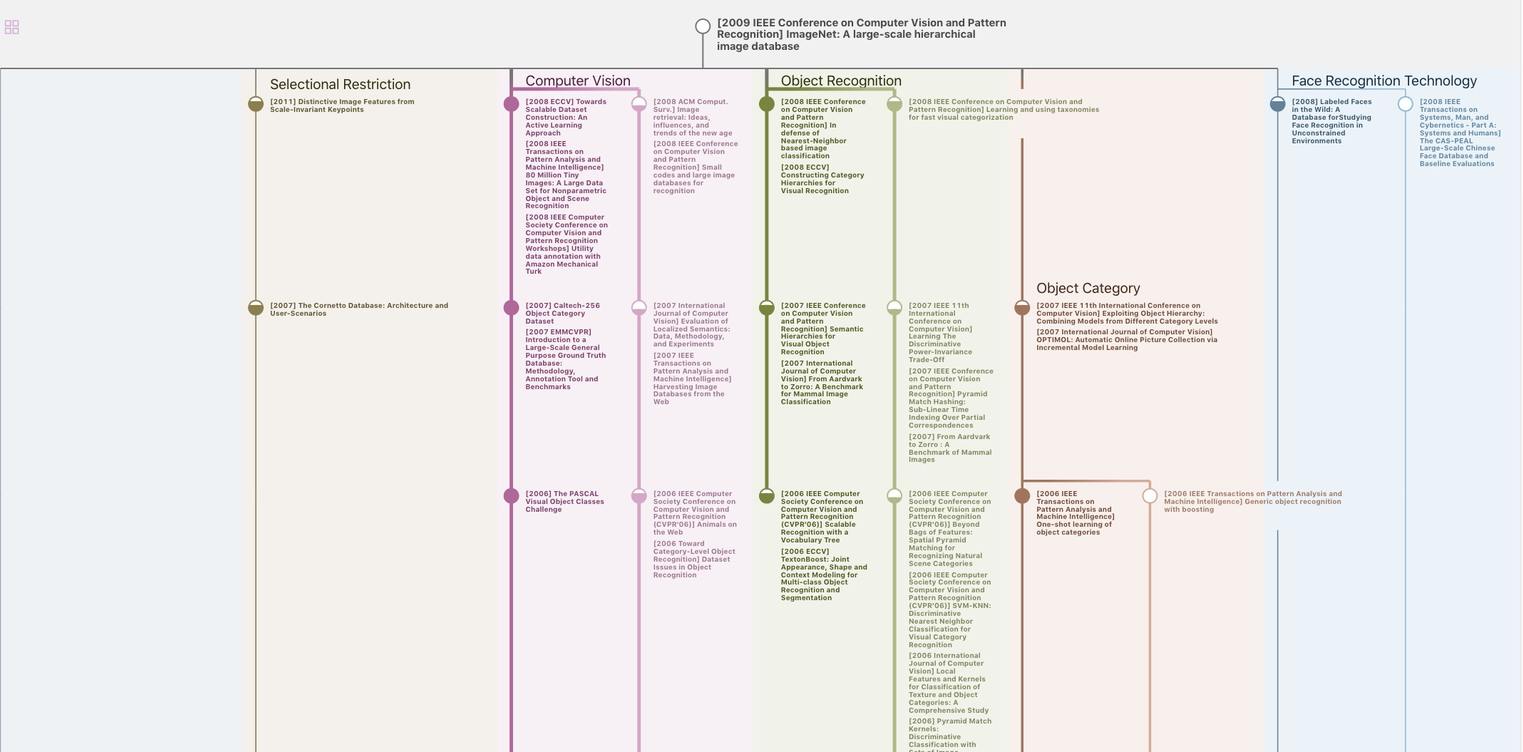
生成溯源树,研究论文发展脉络
Chat Paper
正在生成论文摘要