Timing-Aware Resilience of Data-driven Off-policy Reinforcement Learning for Discrete-Time Systems
2023 AMERICAN CONTROL CONFERENCE, ACC(2023)
摘要
In this paper, we study the impact of clock offsets among different components of cyber-physical systems on data-driven off-policy reinforcement learning (RL) for linear quadratic regulation (LQR). Our results show that under certain conditions the control policies generated by data-driven off-policy RL with clock offsets are stabilizing policies. With clock offsets what directly influences the learning behavior is not only the values of clock offsets but also the dynamics change caused by clock offsets. In particular, larger values of clock offsets do not necessarily lead to non-stabilizing policies. The proposed conclusions are illustrated by numerical simulations.
更多查看译文
AI 理解论文
溯源树
样例
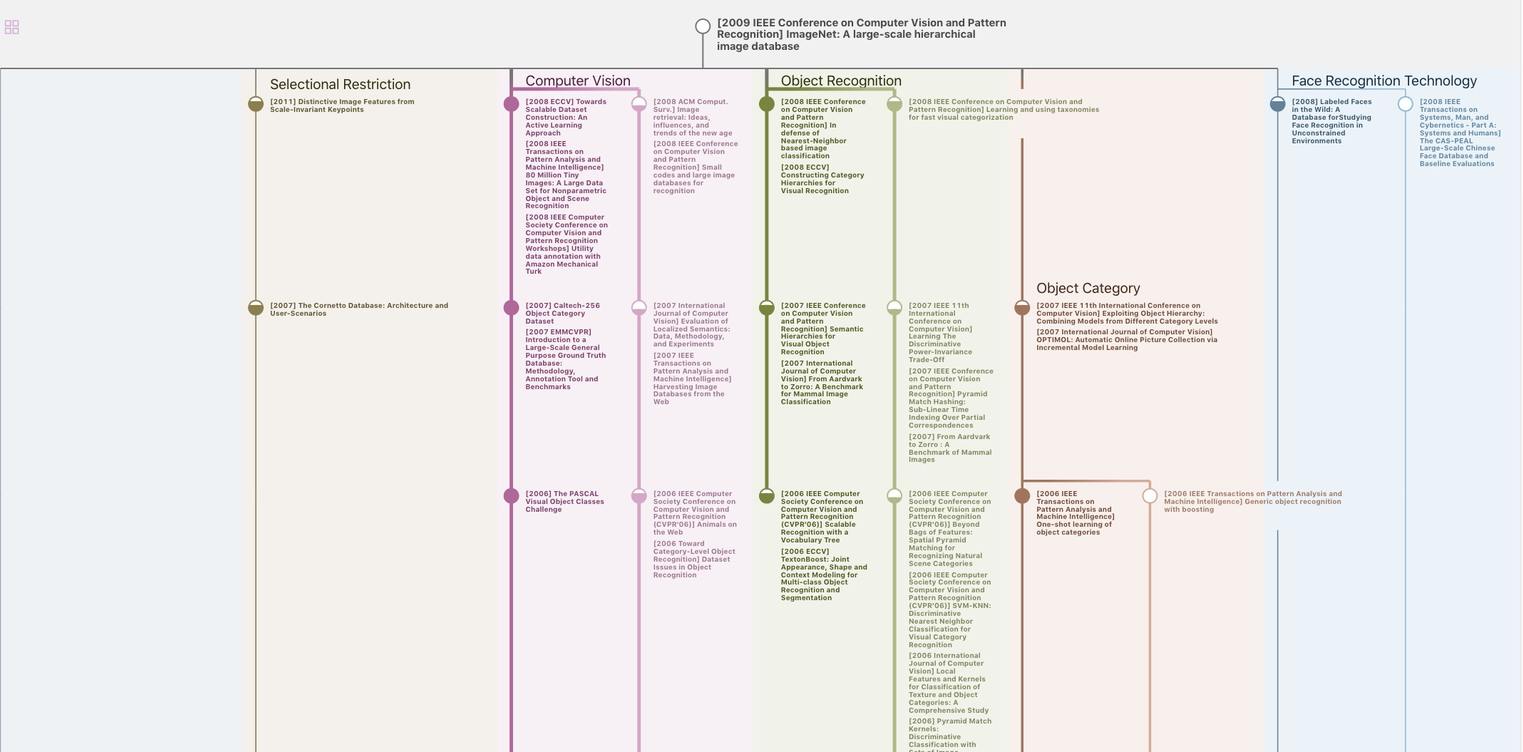
生成溯源树,研究论文发展脉络
Chat Paper
正在生成论文摘要