Stochastic Hybrid Model Predictive Control using Gaussian Processes for Systems with Piecewise Residual Dynamics
2023 AMERICAN CONTROL CONFERENCE, ACC(2023)
摘要
Due to their ability to model complex functions and their suitability for control design, Gaussian Processes (GPs) have recently found widespread use to learn residual dynamics that account for the mismatch between the nominal system model and the true underlying system dynamics. However, in cases where the residual dynamics differ drastically over regions of the state/input space, a single GP-based residual model could be inaccurate. When used to design controllers, this could result in controllers with poor performance that could violate state/input constraints. We propose the use of a GP-based hybrid residual dynamics model, which switches between different residual models across regions of the state and input space. We also design a Model Predictive Controller (MPC) that can leverage this hybrid residual dynamics model to ensure probabilistic constraint satisfaction. Through numerical studies, we demonstrate how the proposed controller outperforms a baseline single GP-based MPC baseline. Simulations show a 45% improvement in control performance in the best-case and probability of constraint violations within the desired threshold in contrast to the baseline approach.
更多查看译文
AI 理解论文
溯源树
样例
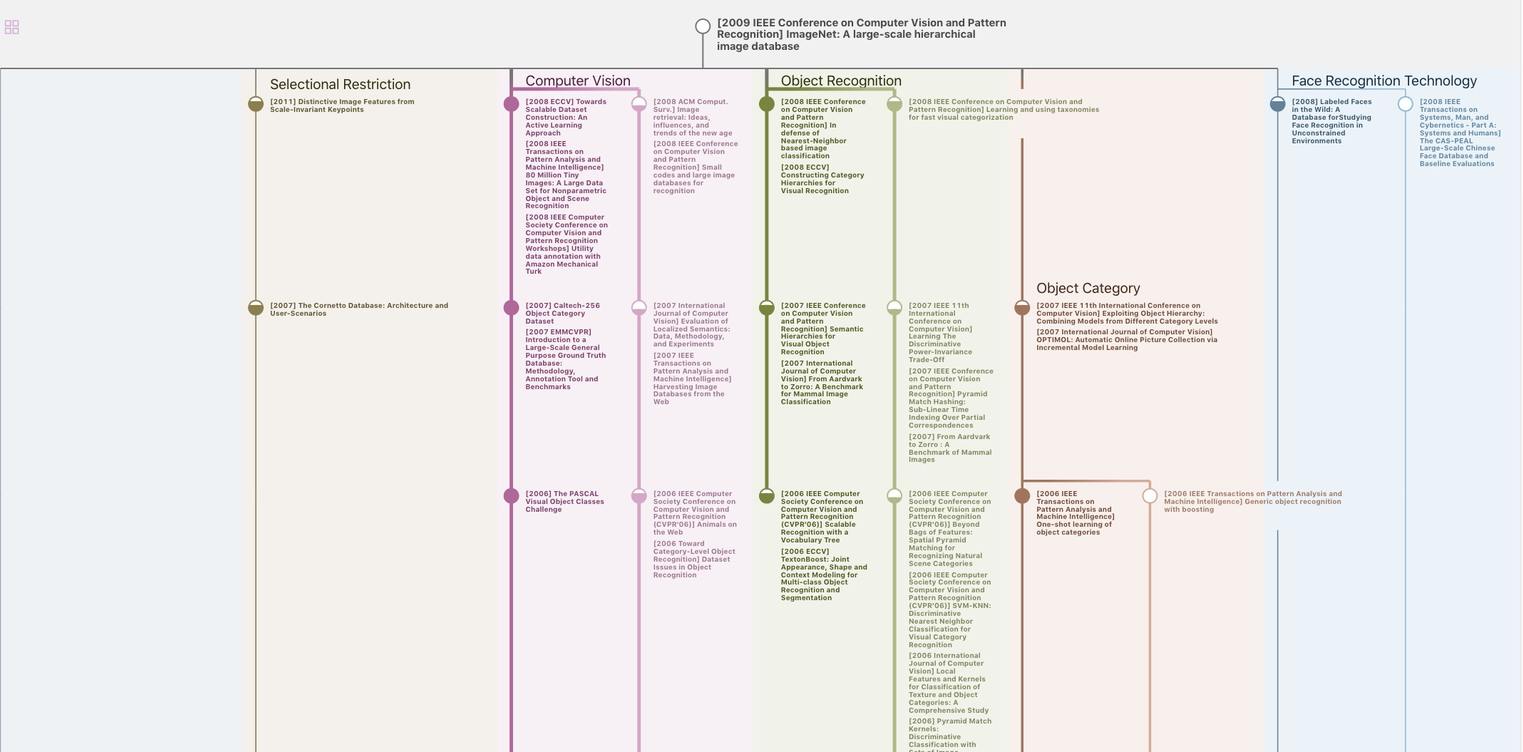
生成溯源树,研究论文发展脉络
Chat Paper
正在生成论文摘要