HEV Energy Management Strategy Based on TD3 with Prioritized Exploration and Experience Replay
2023 AMERICAN CONTROL CONFERENCE, ACC(2023)
摘要
This paper presents a novel energy management strategy for hybrid electric vehicles (HEVs) that is based on an expert twin-delayed deep deterministic policy gradient with prioritized exploration and experience replay (TD3-PEER). State-of-the-art TD3 requires critic networks to generate predicted Q value for state-action pairs to update a policy network. However, the critic networks may struggle with predicting Q values for certain states when the Q values of these states are sensitive to action selection. To address this issue, this paper proposes a prioritized exploration technique that encourages the agent to visit action-sensitive states more frequently in the application of HEV energy management. The proposed algorithm is tested and validated on a P0+P4 HEV model. To simplify the control design, a motor activation threshold is introduced into the final layer of the agent's actor. In addition, dynamic programming results are incorporated into the training of the TD3, helping the agent avoid inefficient operations. Simulation results demonstrate that with expert knowledge considered for all learning-based methods, the proposed TD3-PEER outperforms other RL-based energy management strategies, including DDPG-PER and deep Q-network, by an average of 2.3% and 3.74% over the training and validation cycles, respectively.
更多查看译文
AI 理解论文
溯源树
样例
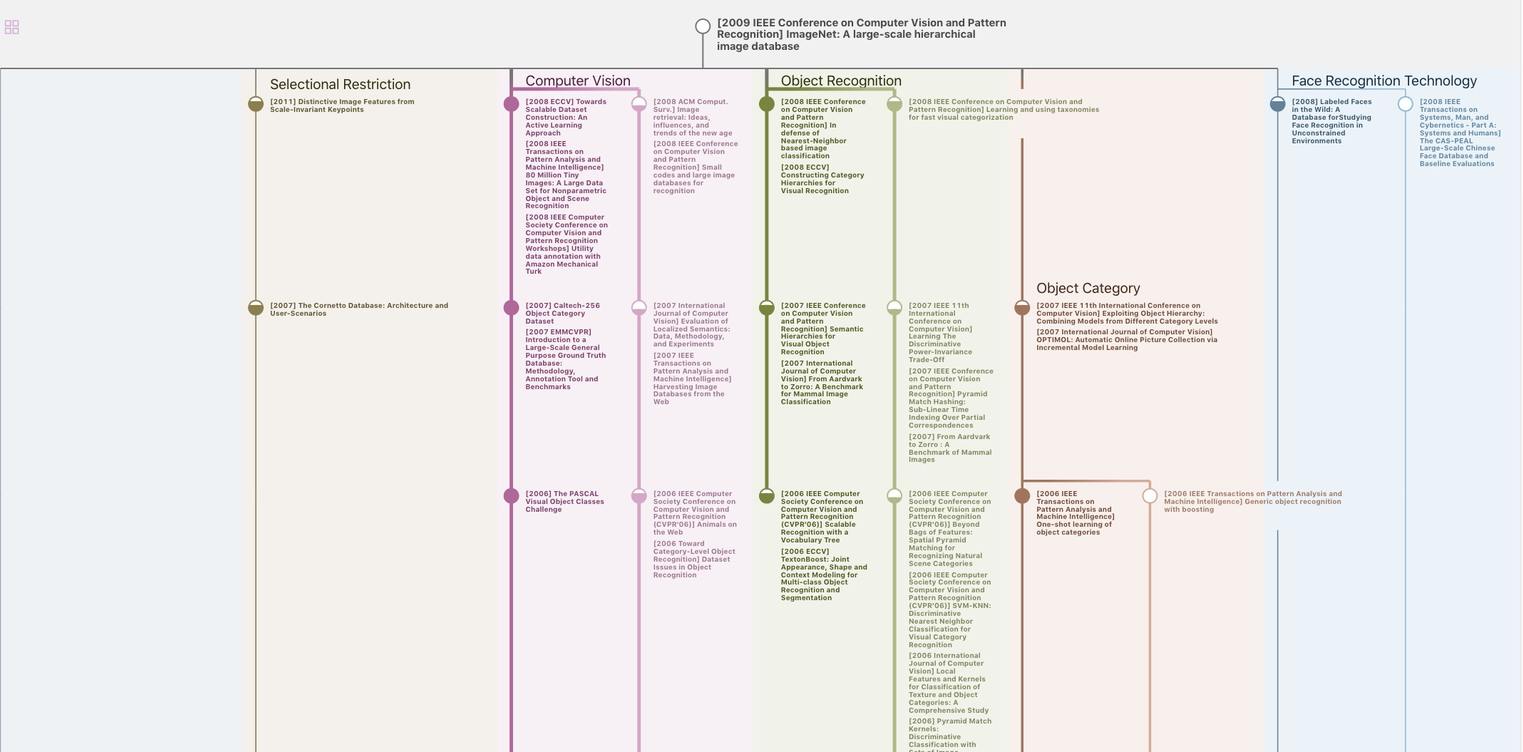
生成溯源树,研究论文发展脉络
Chat Paper
正在生成论文摘要