Adaptive Online Fault Mitigation using Hierarchical Engine Control
2023 AMERICAN CONTROL CONFERENCE, ACC(2023)
摘要
This paper proposes a hierarchical control architecture to mitigate faults and modeling errors in an engine system. A hybrid approach is used to model the complete engine system with the main cylinder combustion process represented using a neural network model and the rest of the system is modeled using well-studied physics-based analytical equations. A control calibration map that consists of the optimal engine control parameters to maintain the desired engine torque using the minimum fuel consumption rate is generated offline by performing Bayesian optimization on this high-fidelity hybrid engine model. We then use proportional-integral (PI) and extremum seeking (ES) controllers on top of the offline map to compensate for any engine faults and modeling errors for online calibration. The work is motivated by optimally reconfiguring autonomous systems. We test three different scenarios through numerical simulations which require online calibration of the engine control parameters. It is shown that the PI+ES controller can overcome the fault by maintaining the desired torque and also lowers the fuel consumption rate compared to a PI controller.
更多查看译文
AI 理解论文
溯源树
样例
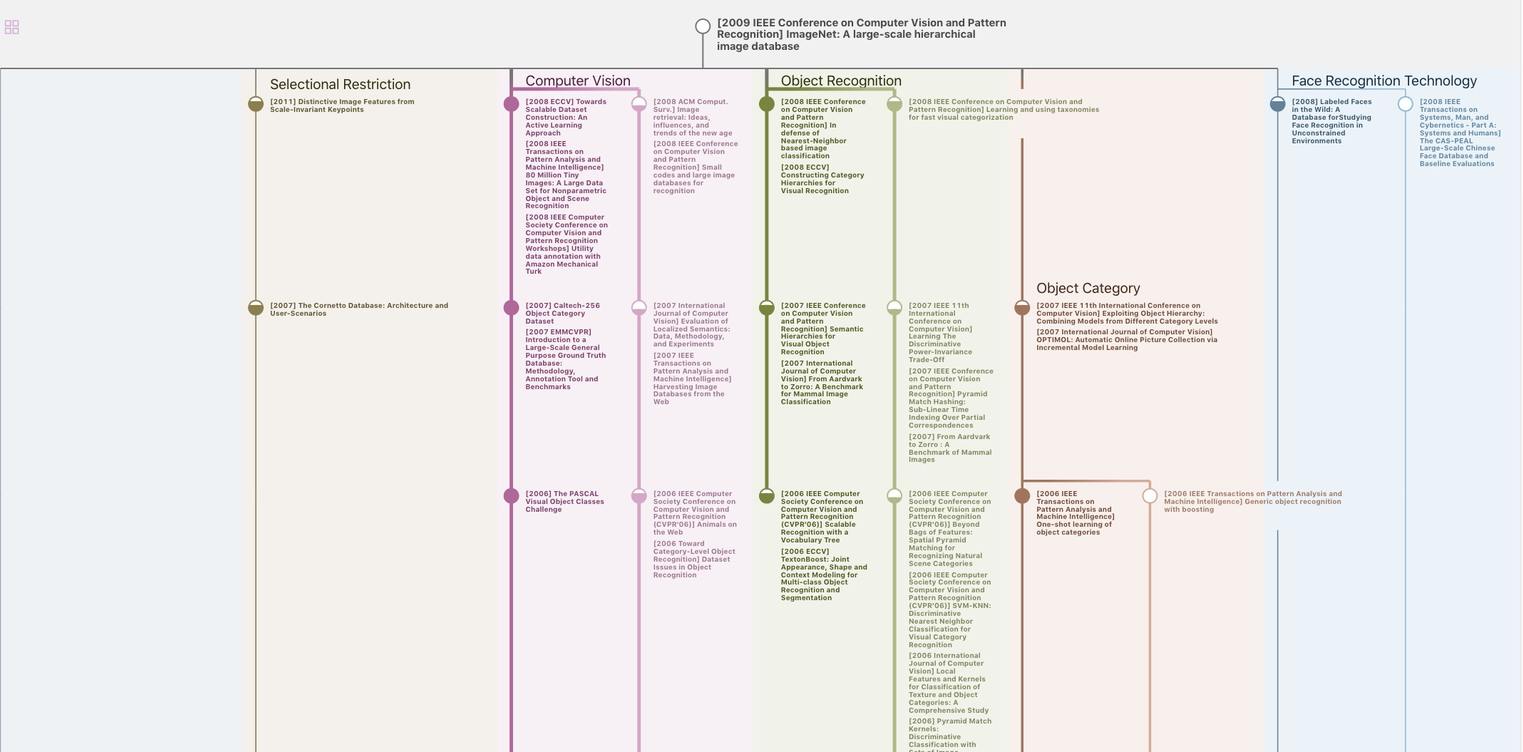
生成溯源树,研究论文发展脉络
Chat Paper
正在生成论文摘要