Locally Differentially Private Frequency Estimation Based on Convolution Framework.
SP(2023)
摘要
Local differential privacy (LDP) collects user data while protecting user privacy and eliminating the need for a trusted data collector. Several LDP protocols have been proposed and deployed in real-world applications. Frequency estimation is a fundamental task in the LDP protocols, which enables more advanced tasks in data analytics. However, the existing LDP protocols amplify the added noise in estimating the frequencies and therefore do not achieve optimal performance in accuracy. This paper introduces a convolution framework to analyze and optimize the estimated frequencies of LDP protocols. The convolution framework can equivalently transform the original frequency estimation problem into a deconvolution problem with noise. We thus add the Wiener filter-based deconvolution algorithms to LDP protocols to estimate the frequency while suppressing the added noise. Experimental results on different real-world datasets demonstrate that our proposed algorithms can lead to significantly better accuracy for state-of-the-art LDP protocols by orders of magnitude for the smooth dataset. And these algorithms also work on non-smooth datasets, but only to a limited extent. Our code is available at https://github.com/SEUNICK/LDP.
更多查看译文
关键词
Local-Differential-Privacy,Convolution-Framework,Wiener-Deconvolution
AI 理解论文
溯源树
样例
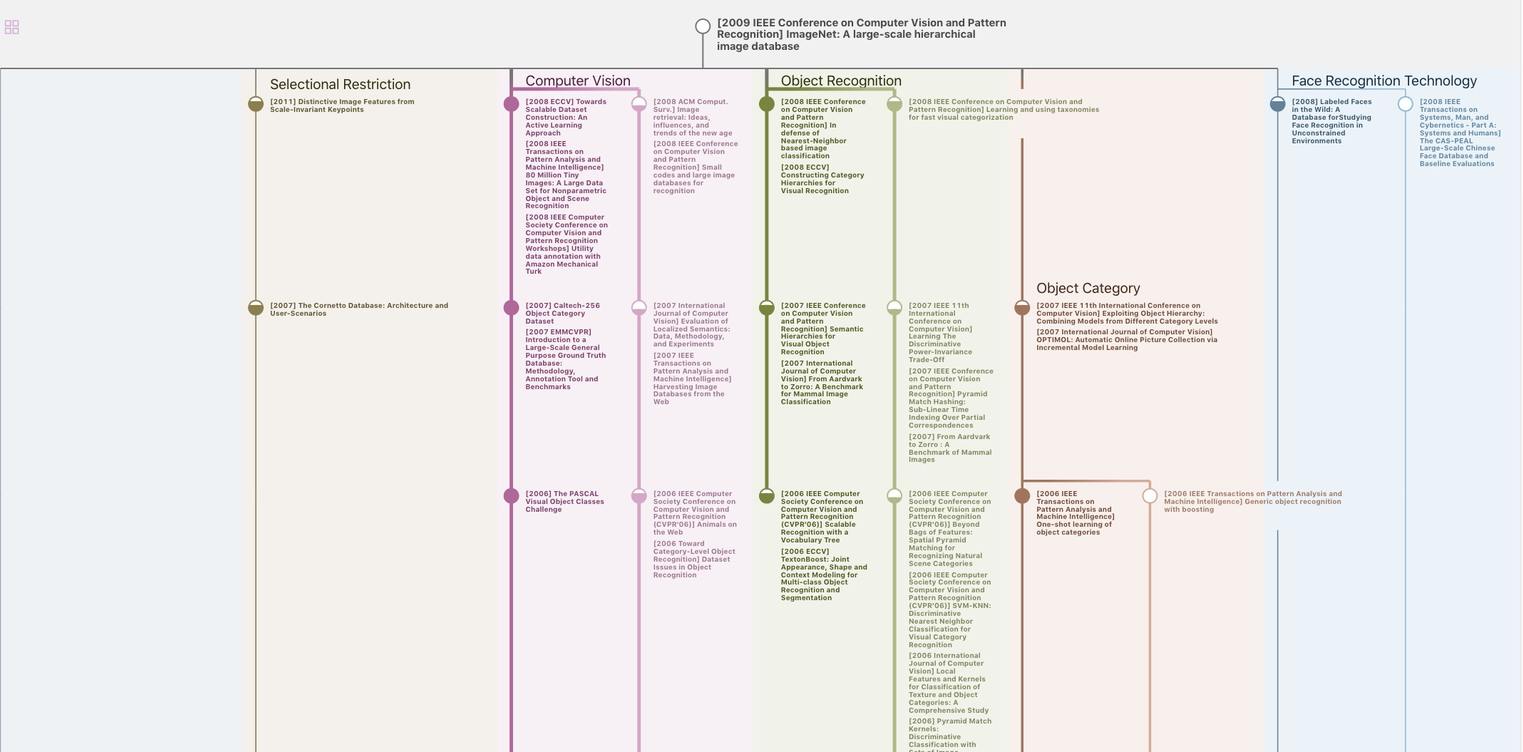
生成溯源树,研究论文发展脉络
Chat Paper
正在生成论文摘要