Neuroevolution for the Sustainable Evolution of Neural Networks.
MIPRO(2023)
摘要
The predictive performance of a neural network depends on its weights and architecture. optimizers based on gradient descent are most commonly used to optimize the weights, and grid search is utilized to find the most suitable architecture from the list of predefined architectures. On the other hand, neuroevolution offers a solution for the simultaneous growth of neural network architecture and the evolution of its weights. Thus, it is not limited by the user-defined list of possible architectures and can find configurations optimal for a specific task. Both approaches can be effectively parallelized and take advantage of modern multi-process systems. In this research, we compare neuroevolution and backpropagation in terms of the time consumed by the algorithm, the predictive performance of the neural network, and the complexity of the neural network. The total time for each algorithm is measured along with the times for each section of the algorithm and the time spent on synchronization due to the multi-process setting. The neural networks are compared by their predictive performance in terms of Matthews correlation coefficient score and their complexity as the number of nodes and connections. The case study is based on two synthetic and two real-world datasets for classification tasks.
更多查看译文
关键词
machine learning,neuroevolution,neural networks,genetic algorithm,sustainability,classification
AI 理解论文
溯源树
样例
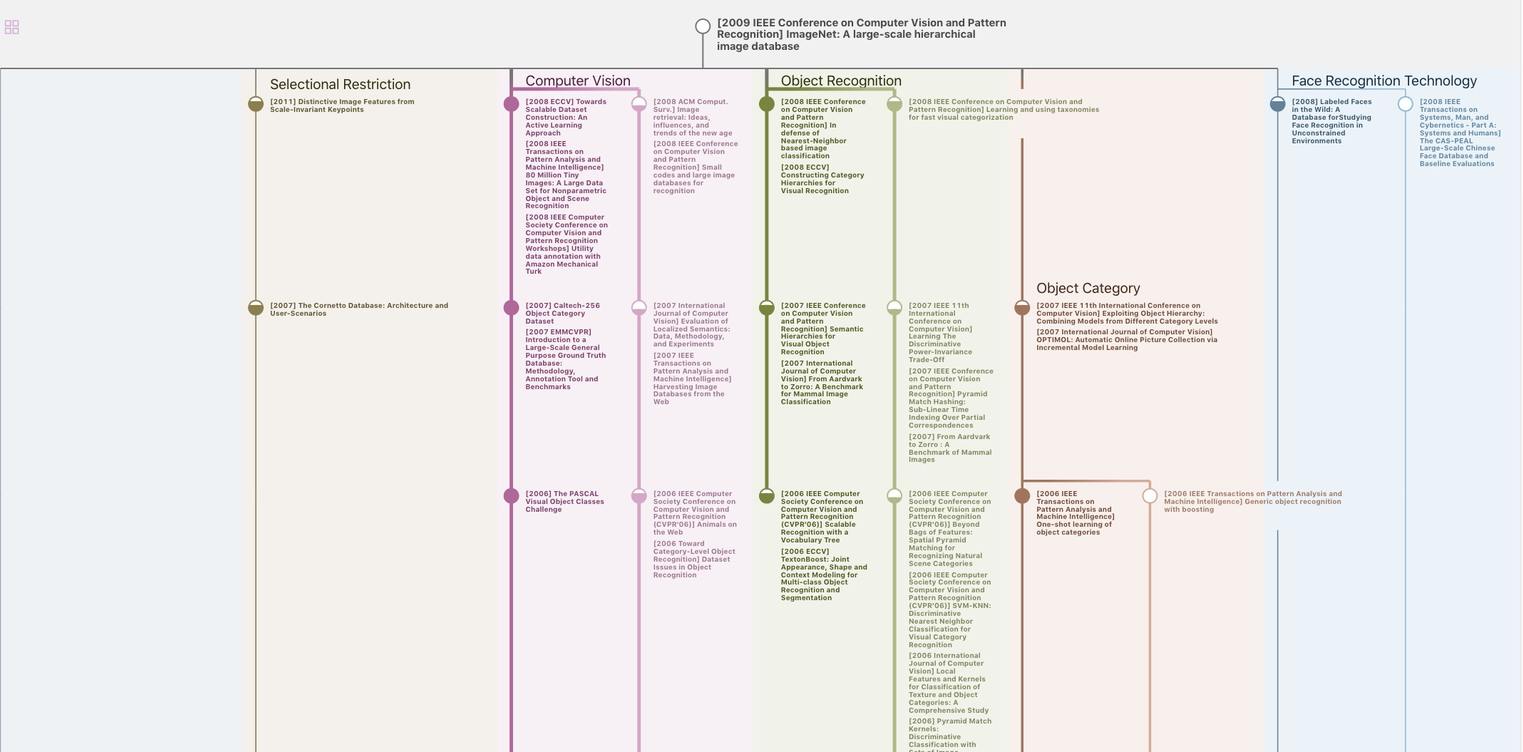
生成溯源树,研究论文发展脉络
Chat Paper
正在生成论文摘要