Subject-Independent P300 Speller Classification using Time-Frequency Representation and Double Input CNN with Feature Concatenation.
DSP(2023)
摘要
This study proposes a Double Input Convolutional Neural Network with Feature Concatenation (DiCNN-FC) for the classification task of the P300 speller. Two time-frequency representations of electroencephalography (EEG); namely, power and phase spectrograms; have been employed as input for the DiCNN-FC. Each spectrogram has been processed separately using convolutional layers and concatenated with each other for decision-making in the dense layers. The use of DiCNN-FC produces reliable results as the decision is made based on two sets of features. Two P300 datasets, one from amyotrophic lateral sclerosis (ALS) patients, and another from healthy subjects have been used to evaluate the performance of the proposed method. The performance comparison has been performed for two classical methods for P300 classification, namely Support Vector Machine (SVM) and Linear Discriminant Analysis (LDA). The achieved results show the DiCNN-FC model’s ability to perform subject-independent P300 component identification based on single-trial data on both datasets.
更多查看译文
关键词
Brain-Computer Interface,P300 Speller,Time-Frequency Representation,Convolutional Neural Network
AI 理解论文
溯源树
样例
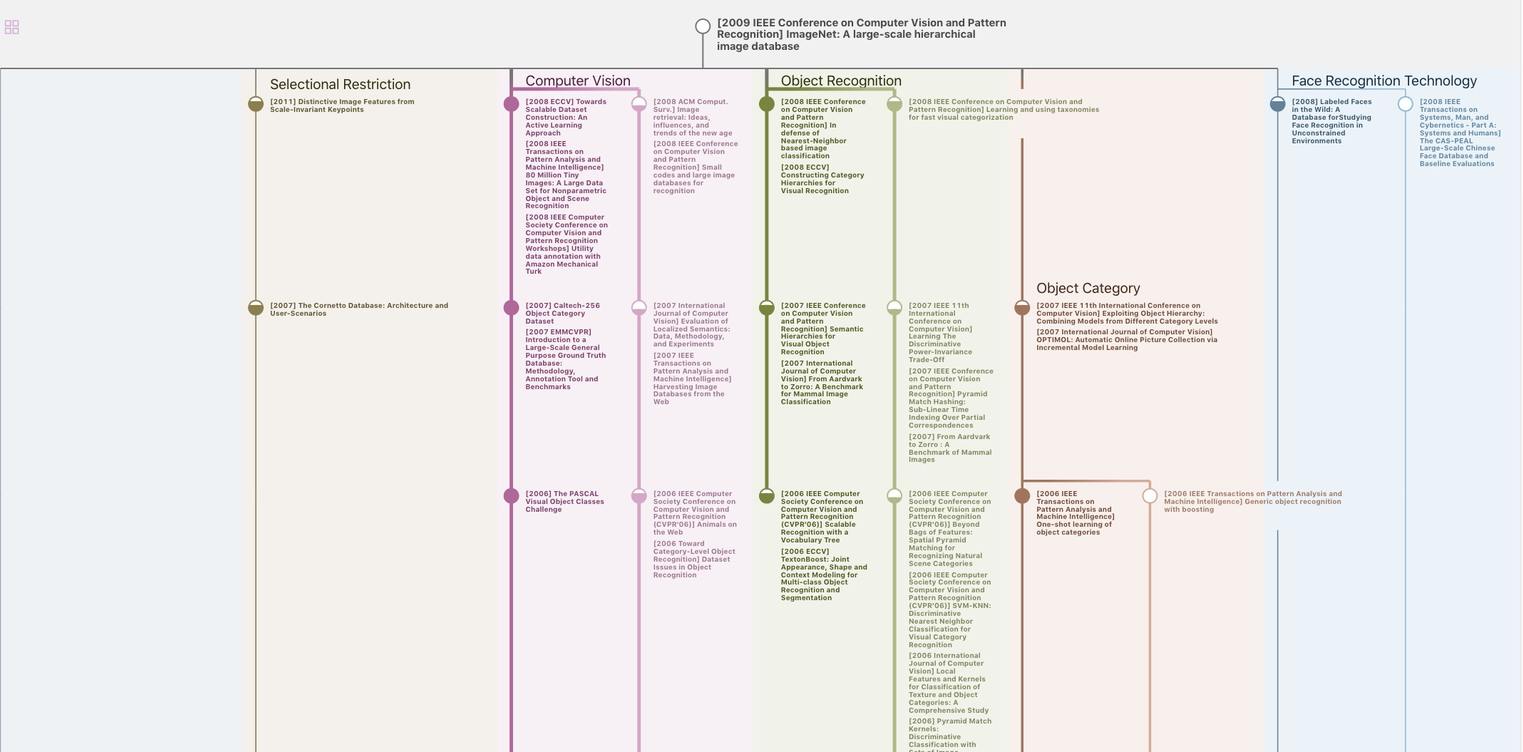
生成溯源树,研究论文发展脉络
Chat Paper
正在生成论文摘要