Unsupervised Anomaly Detection using Deep Autoencoding Mixture of Probabilistic Principal Component Analyzers.
ICIIT(2023)
摘要
Although many unsupervised anomaly detection algorithms with outstanding performance have been proposed in last few decades, their performance for high-dimensional data is not guaranteed. Therefore, this paper proposes a new framework called deep autoencoding mixture of probabilistic principal component analyzers (DA-MPPCA). This framework uses deep autoencoder (DAE) as a compression network to prepare the low-dimensional representations for a subsequent estimation network NN-MPPCA. Different to conventional MPPCA that is trained by EM algorithm, NN-MPPCA is the neural network form of MPPCA which parameters can be updated via back-propagation algorithm. By jointly training DAE and NN-MPPCA in an end-to-end manner using a defined loss function, we force both dimensionality reduction and density estimation tasks into the unified framework. The experimental results on a variety of public datasets have demonstrated the superior performance of DA-MPPCA over both shallow and deep baseline models with an improvement on F1-score up to 7% over the best baseline.
更多查看译文
关键词
Anomaly detection, deep autoencoder, mixture of probabilistic principal component analyzes
AI 理解论文
溯源树
样例
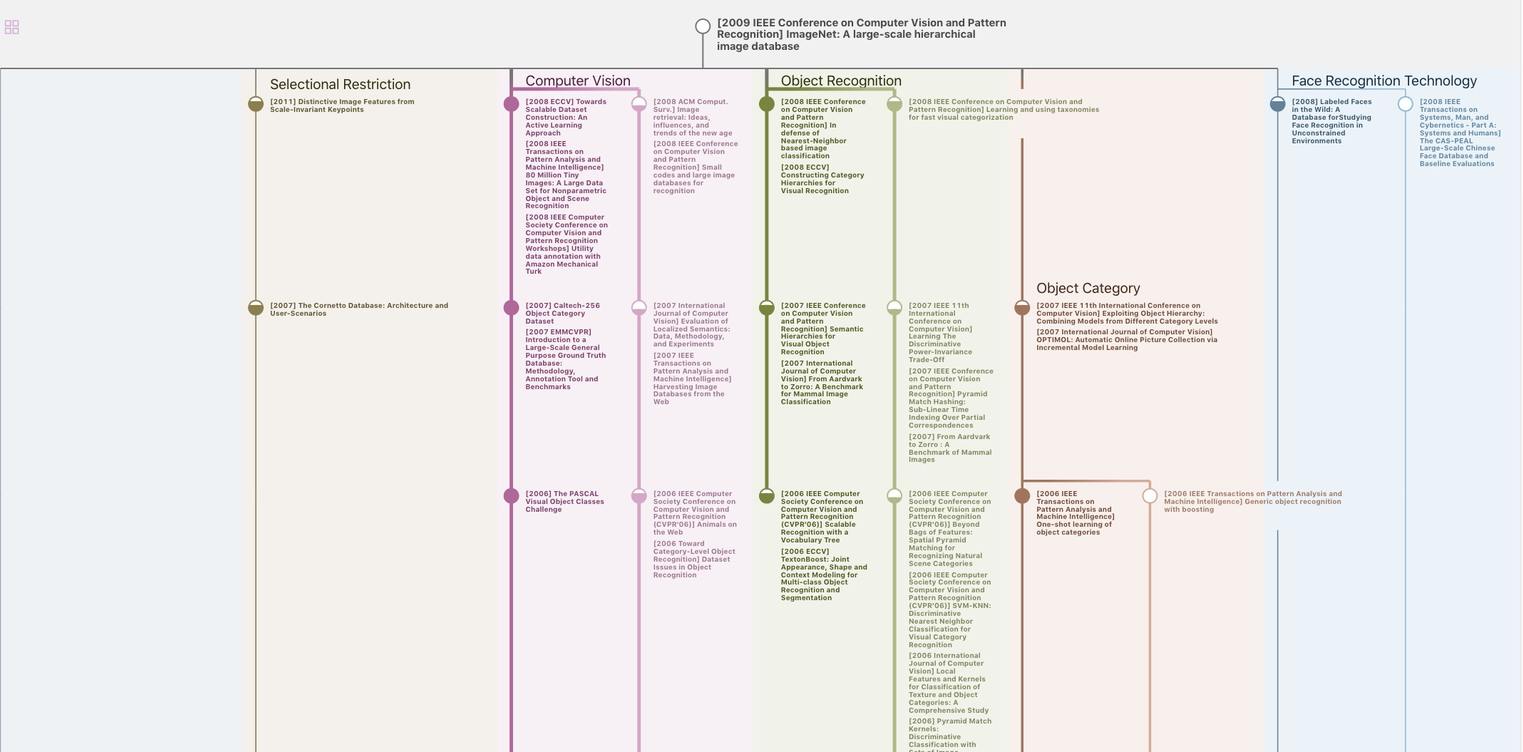
生成溯源树,研究论文发展脉络
Chat Paper
正在生成论文摘要