Generalization Properties of Adversarial Training for `0-Bounded Adversarial Attacks
ITW(2023)
摘要
We have widely observed that neural networks are vulnerable to small additive perturbations to the input causing misclassification. In this paper, we focus on the l(0) -bounded adversarial attacks, and aim to theoretically characterize the performance of adversarial training for an important class of truncated classifiers. Such classifiers are shown to have strong performance empirically, as well as theoretically in the Gaussian mixture model, in the l(0)-adversarial setting. The main contribution of this paper is to prove a novel generalization bound for the binary classification setting with l(0)-bounded adversarial perturbation that is distribution-independent. Deriving a generalization bound in this setting has two main challenges: (i) the truncated inner product which is highly non-linear; and (ii) maximization over the l(0) ball due to adversarial training is non-convex and highly non-smooth. To tackle these challenges, we develop new coding techniques for bounding the combinatorial dimension of the truncated hypothesis class.
更多查看译文
关键词
adversarial training,binary classification setting,bounded adversarial attacks,bounded adversarial perturbation,coding techniques,Gaussian mixture model,generalization bound,neural networks
AI 理解论文
溯源树
样例
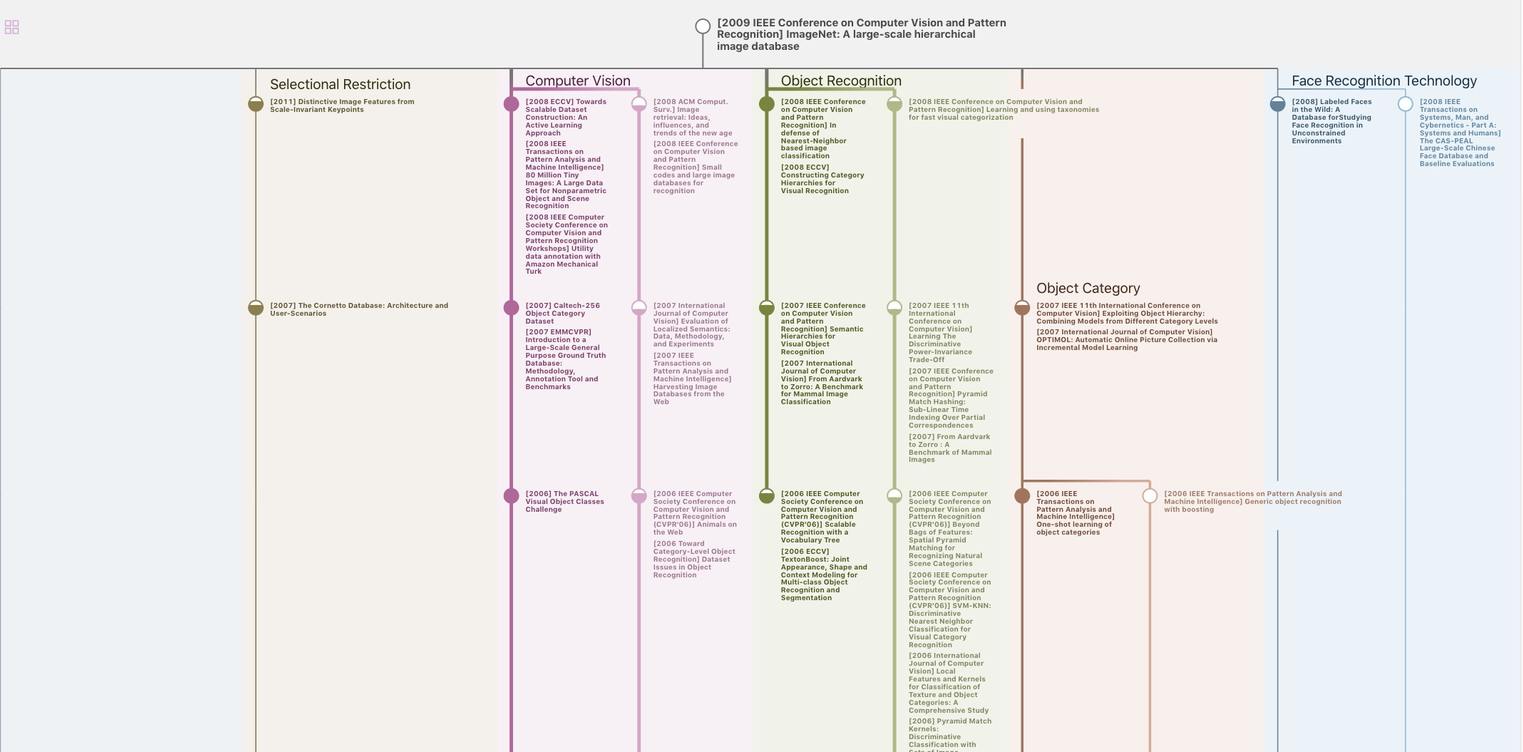
生成溯源树,研究论文发展脉络
Chat Paper
正在生成论文摘要