A Simulation Environment for Reducing Food Waste via Reinforcement Learning.
ANNSIM(2023)
摘要
Food retailers discard astonishing amounts of spoiled produce as waste. Reinforcement learning could greatly improve retail store product ordering and pricing decisions to simultaneously reduce food waste and improve business profits. We present a discrete-event-based food retail simulation framework which simulates wholesaler, store, and customer interactions. This simulator is essential for driving development and testing of future reinforcement learning methods to help economically reduce food waste for food retail stores. Simulation provides an efficient learning feedback system across a massive number of possible scenarios, which cannot be replicated from live observation or pure historical data alone. We demonstrate our simulator on an example built from historical consumption and price data. A simple realistic baseline resulted in more than US$1M of food waste (2010-2015). A soft actor critic (SAC) RL agent increased profit by 42% and reduced food waste by almost US$500k over three years (2012-2015) after learning from simulations (2010-2012).
更多查看译文
关键词
discrete event simulation, reinforcement learning, food retail, food waste
AI 理解论文
溯源树
样例
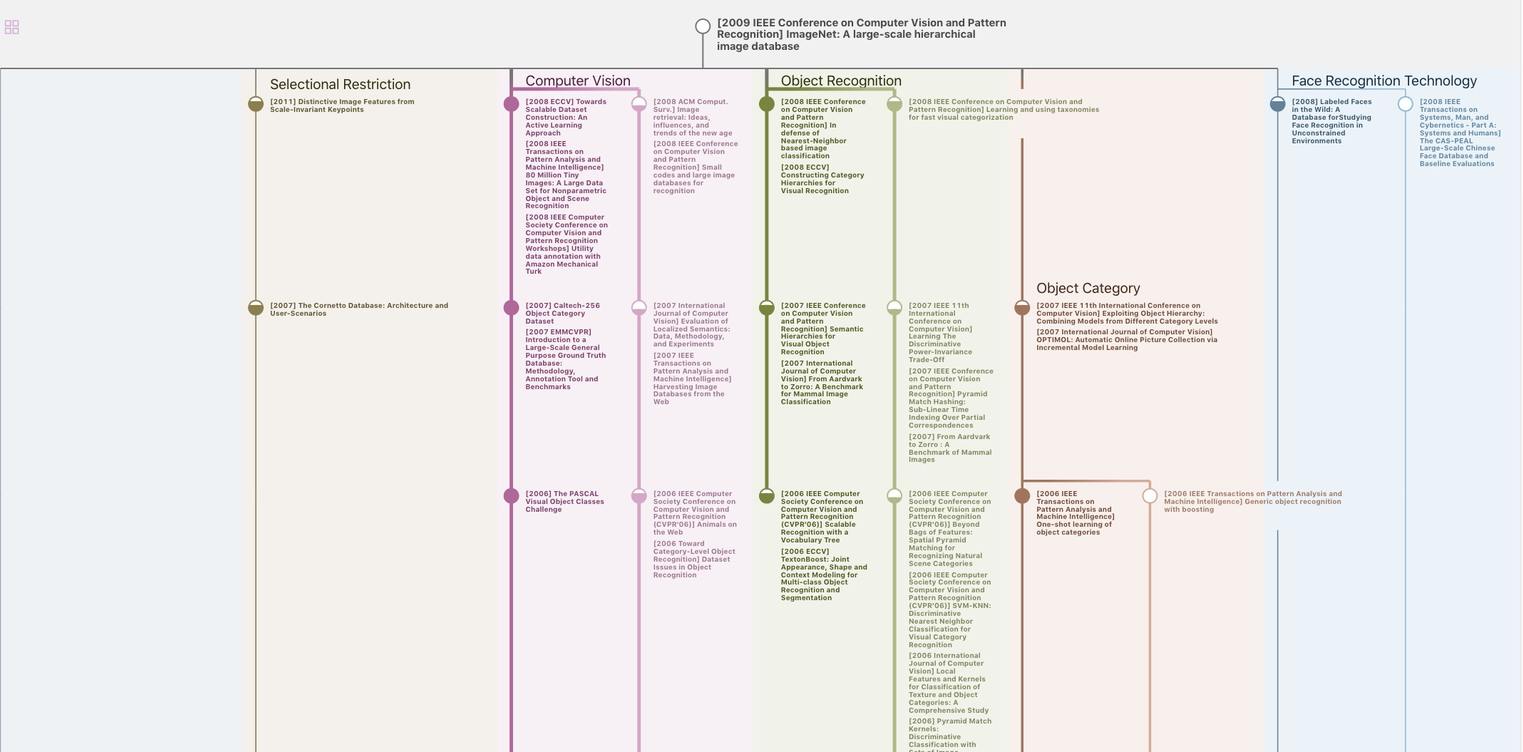
生成溯源树,研究论文发展脉络
Chat Paper
正在生成论文摘要