Optimising Ferroelectric Thin Films with Evolutionary Computation
PROCEEDINGS OF THE 2023 GENETIC AND EVOLUTIONARY COMPUTATION CONFERENCE COMPANION, GECCO 2023 COMPANION(2023)
摘要
This paper presents the integration of machine learning and image analysis techniques into a material science experimental workflow. The aim is to optimise the properties of an Aluminium Scandium Nitride thin film through the manipulation of experimental input parameters. This is formulated as an optimisation problem, were the search space consists in the set of experimental input parameters used during the film's synthesis. The solution's fitness is obtained through the analysis of Scanning-Electron-Microscopy images and corresponds to the surface defect density over a film. An optimum solution to this problem is defined as the set of input parameters that consistently produces a film with no measurable surface defects. The search space is a black box with possibly more than one optimum and the limited amount of experiments that can be undertaken make efficient exploration challenging. It is shown that classification can be used to reduce the problem's search space by identifying areas of infeasibility. Using nested cross-validation, tree-based classifiers emerge as the most accurate, and importantly, interpretable algorithms for this task. Subsequently, Particle Swarm Optimisation is used to find optimal solutions to the surface defect minimisation problem. Preliminary experimental results show a significant decrease in defect density average achieved.
更多查看译文
AI 理解论文
溯源树
样例
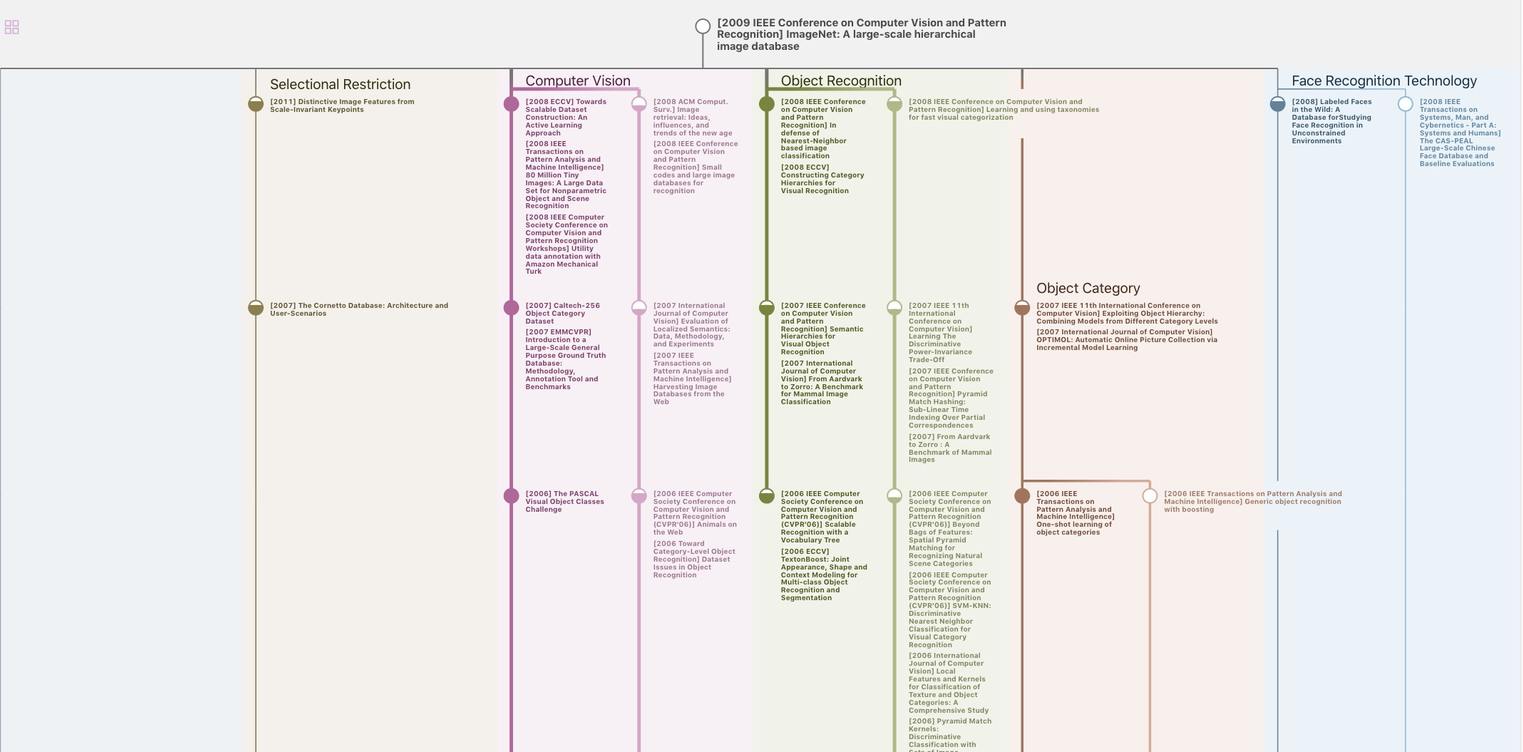
生成溯源树,研究论文发展脉络
Chat Paper
正在生成论文摘要