The Problem Solving Benefits of Down-sampling Vary by Selection Scheme
PROCEEDINGS OF THE 2023 GENETIC AND EVOLUTIONARY COMPUTATION CONFERENCE COMPANION, GECCO 2023 COMPANION(2023)
摘要
Genetic programming systems often use large training sets to evaluate candidate solutions, which can be computationally expensive. Down-sampling training sets has long been used to decrease the computational cost of evaluation in a wide range of application domains. Indeed, recent studies have shown that both random and informed down-sampling can substantially improve problem-solving success for GP systems that use lexicase parent selection. We use the PushGP framework to experimentally test whether these downsampling techniques can also improve problem-solving success in the context of two other commonly used selection methods, fitness-proportionate and tournament selection, across eight GP problems (four program synthesis and four symbolic regression). We verified that down-sampling can benefit the problem-solving success of both fitness-proportionate and tournament selection. However, the number of problems wherein down-sampling improved problem-solving success varied by selection scheme, suggesting that the impact of down-sampling depends both on the problem and choice of selection scheme. Surprisingly, we found that down-sampling was most consistently beneficial when combined with lexicase selection as compared to tournament and fitness-proportionate selection. Overall, our results suggest that down-sampling should be considered more often when solving test-based GP problems.
更多查看译文
关键词
down-sampling,program synthesis,regression,genetic programming,selection
AI 理解论文
溯源树
样例
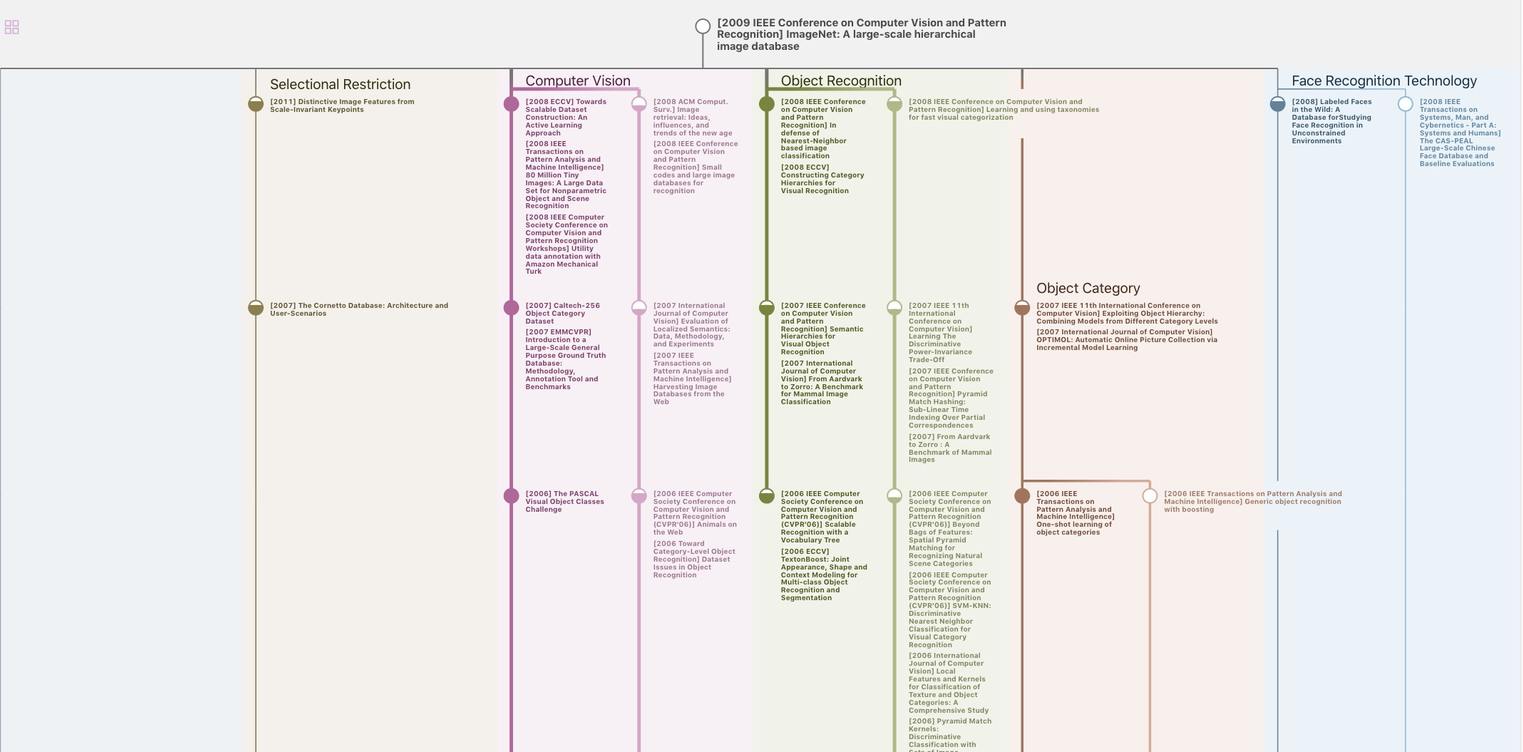
生成溯源树,研究论文发展脉络
Chat Paper
正在生成论文摘要