Explainable Human-Machine Teaming using Model Checking and Interpretable Machine Learning.
FormaliSE(2023)
摘要
The human-machine teaming paradigm promotes tight teamwork between humans and autonomous machines that collaborate in the same physical space. This paradigm is increasingly widespread in critical domains, such as healthcare and domestic assistance. These systems are expected to build a certain level of trust by enforcing dependability and exhibiting interpretable behavior. However, trustworthiness is negatively affected by the black-box nature of these systems, which typically make fully autonomous decisions that may be confusing for humans or cause hazards in critical domains. We present the EASE approach, whose goal is to build better trust in human-machine teaming leveraging statistical model checking and model-agnostic interpretable machine learning. We illustrate EASE through an example in healthcare featuring an infinite (dense) space of human-machine uncertain factors, such as diverse physical and physiological characteristics of the agents involved in the teamwork. Our evaluation demonstrates the suitability and cost-effectiveness of EASE in explaining dependability properties in human-machine teaming.
更多查看译文
关键词
Human-machine teaming,formal analysis,statistical model checking,interpretable machine learning
AI 理解论文
溯源树
样例
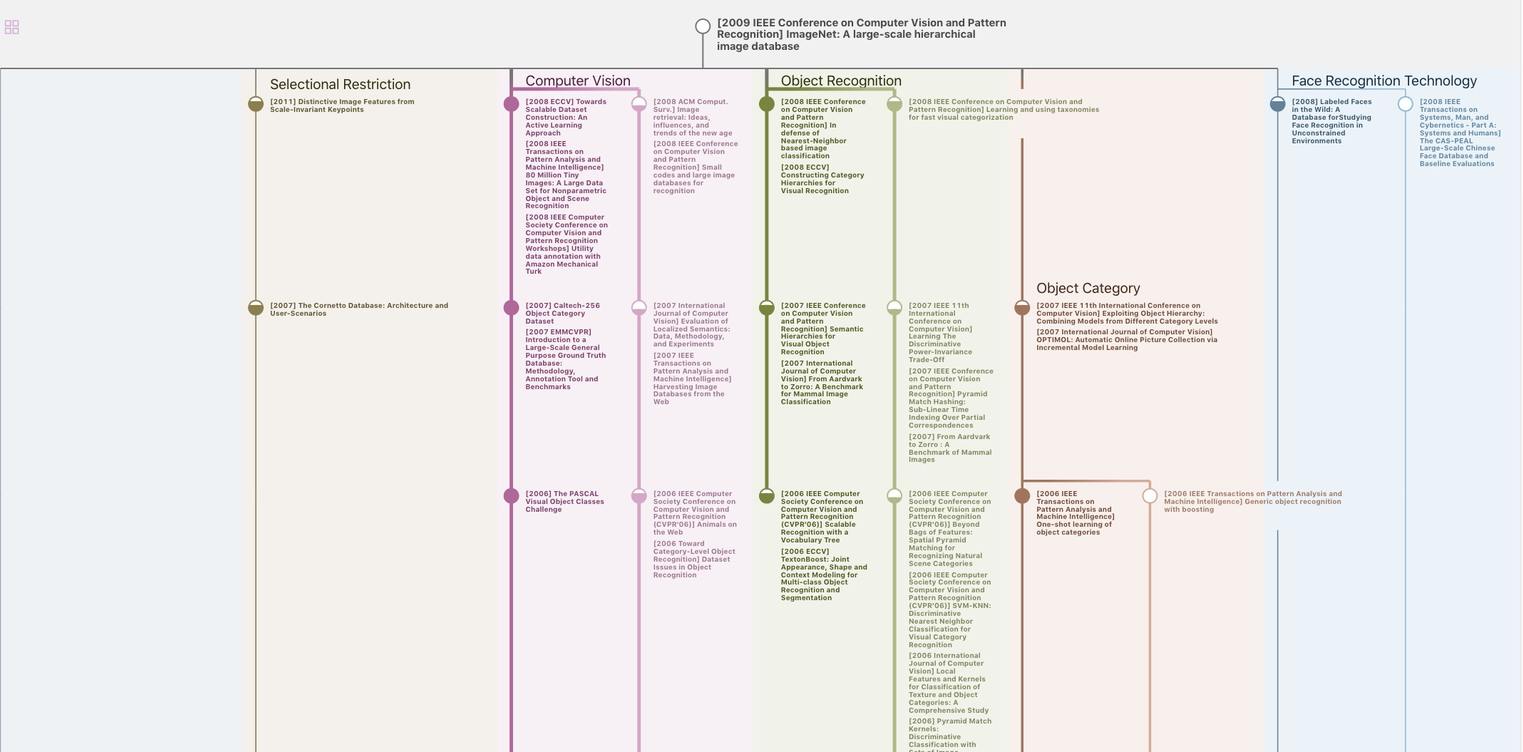
生成溯源树,研究论文发展脉络
Chat Paper
正在生成论文摘要