Soteria: Preserving Privacy in Distributed Machine Learning
38TH ANNUAL ACM SYMPOSIUM ON APPLIED COMPUTING, SAC 2023(2023)
摘要
We propose Soteria, a system for distributed privacy-preserving Machine Learning (ML) that leverages Trusted Execution Environments (e.g. Intel SGX) to run code in isolated containers (enclaves). Unlike previous work, where all ML-related computation is performed at trusted enclaves, we introduce a hybrid scheme, combining computation done inside and outside these enclaves. The conducted experimental evaluation validates that our approach reduces the runtime of ML algorithms by up to 41%, when compared to previous related work. Our protocol is accompanied by a security proof, as well as a discussion regarding resilience against a wide spectrum of ML attacks.
更多查看译文
关键词
Apache Spark,Machine Learning,Intel SGX,Privacy-Preserving
AI 理解论文
溯源树
样例
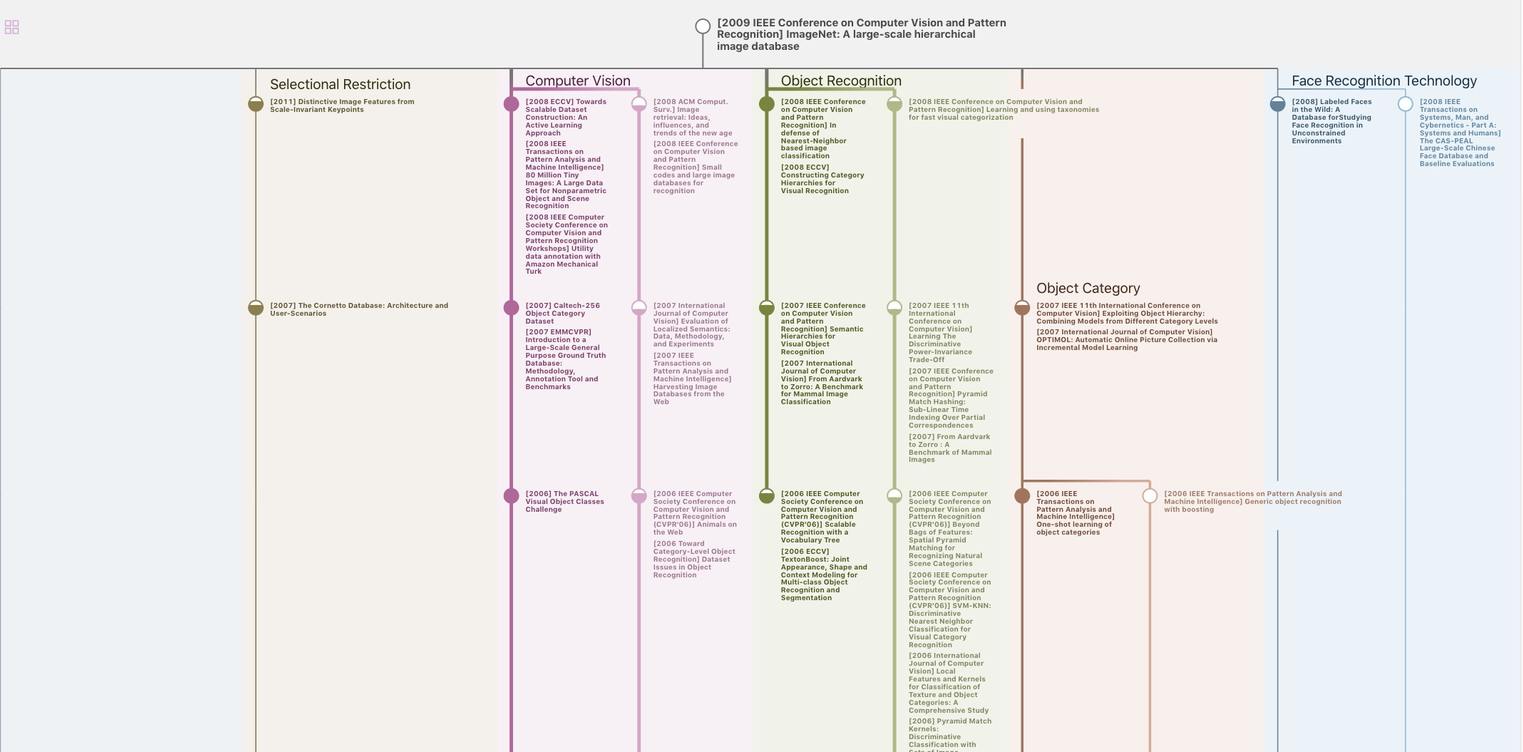
生成溯源树,研究论文发展脉络
Chat Paper
正在生成论文摘要