Neuroevolution of Physics-Informed Neural Nets: Benchmark Problems and Comparative Results
PROCEEDINGS OF THE 2023 GENETIC AND EVOLUTIONARY COMPUTATION CONFERENCE COMPANION, GECCO 2023 COMPANION(2023)
摘要
The potential of learned models for fundamental scientific research and discovery is drawing increasing attention worldwide. Physics-informed neural networks (PINNs), where the loss function directly embeds governing equations of scientific phenomena, is one of the key techniques at the forefront of recent advances. PINNs are typically trained using stochastic gradient descent methods, akin to their deep learning counterparts. However, analysis in this paper shows that PINNs' unique loss formulations lead to a high degree of complexity and ruggedness that may not be conducive for gradient descent. Unlike in standard deep learning, PINN training requires globally optimum parameter values that satisfy physical laws as closely as possible. Spurious local optimum, indicative of erroneous physics, must be avoided. Hence, neuroevolution algorithms, with their superior global search capacity, may be a better choice for PINNs relative to gradient descent methods. Here, we propose a set of five benchmark problems, with open-source codes, spanning diverse physical phenomena for novel neuroevolution algorithm development. Using this, we compare two neuroevolution algorithms against the commonly used stochastic gradient descent, and our baseline results support the claim that neuroevolution can surpass gradient descent, ensuring better physics compliance in the predicted outputs.
更多查看译文
关键词
Neuroevolution,stochastic gradient descent,physics-informed neural networks,benchmarks
AI 理解论文
溯源树
样例
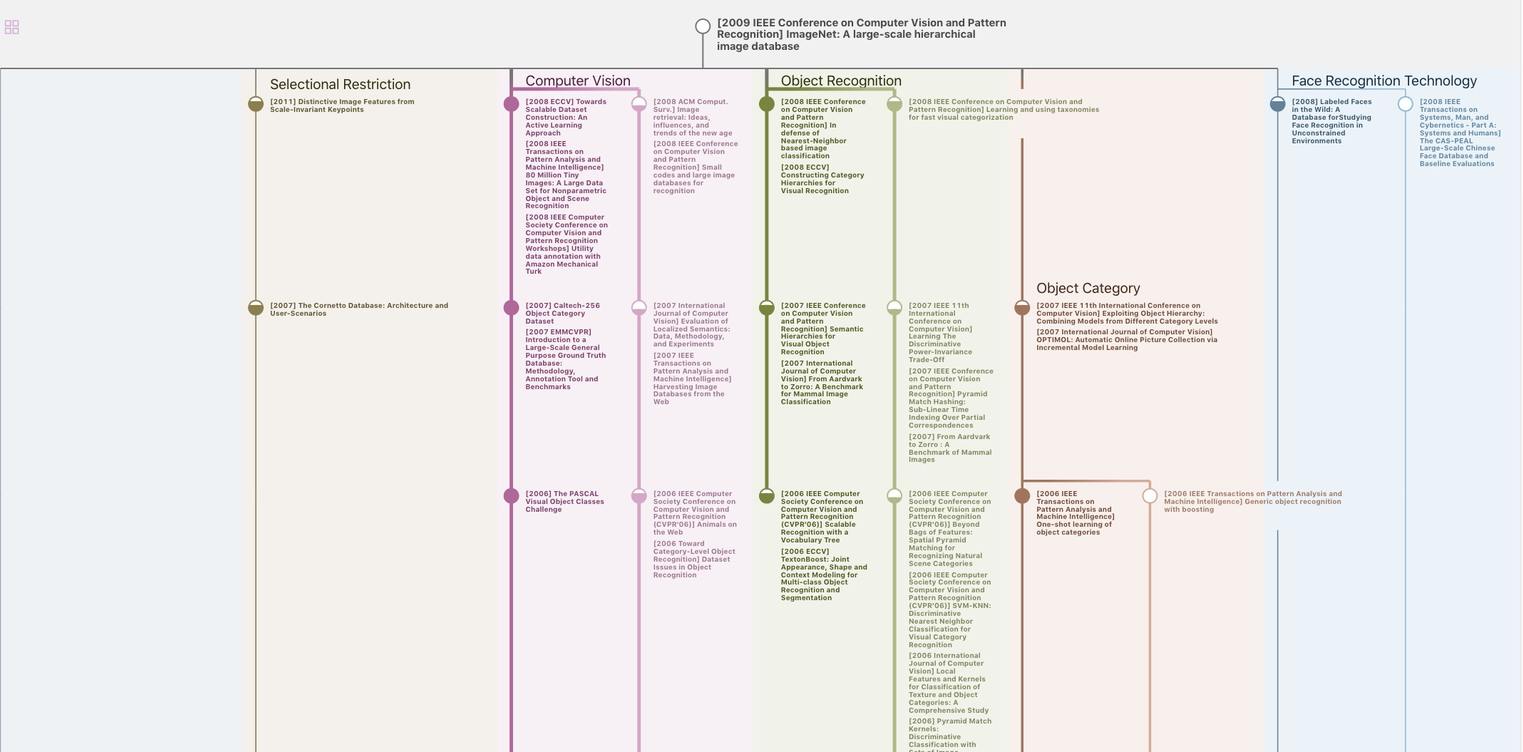
生成溯源树,研究论文发展脉络
Chat Paper
正在生成论文摘要