A Two-Stage Multi-Objective Evolutionary Reinforcement Learning Framework for Continuous Robot Control
GECCO(2023)
摘要
Real-world continuous control problems often require optimizing for multiple conflicting objectives. Various works in multi-objective reinforcement learning have been conducted to tackle such issues and obtained impressive performance. At the same time, evolutionary algorithms (EAs), which are extensively used in multi-objective optimization, have recently been demonstrated their competitiveness to RL algorithms, including multi-objective control for environments with discrete action spaces. However, using EAs for multi-objective continuous robot control is still an under-explored topic. For the single-objective setting, the Proximal Distilled Evolutionary Reinforcement Learning (PDERL) framework succeeds in combining the robustness of EA and the efficiency of RL methods. In this work, we bring the strengths of PDERL to the multiobjective realm to create the novel multi-objective PDERL framework called MOPDERL that consists of a warm-up stage and an evolution stage. In particular, MOPDERL collaboratively optimizes policies for each separate objective in the warm-up stage, and then exchanges that knowledge for further policy improvement during the multi-objective evolution stage. We benchmark MOPDERL on six MuJoCo robot locomotion environments, which have been modified for the multi-objective context. The results show that MOPDERL produces better-quality Pareto fronts and higher metric scores than the state-of-the-art PGMORL algorithm across five out of six environments.
更多查看译文
关键词
reinforcement learning,multi-objective optimization,genetic algorithms
AI 理解论文
溯源树
样例
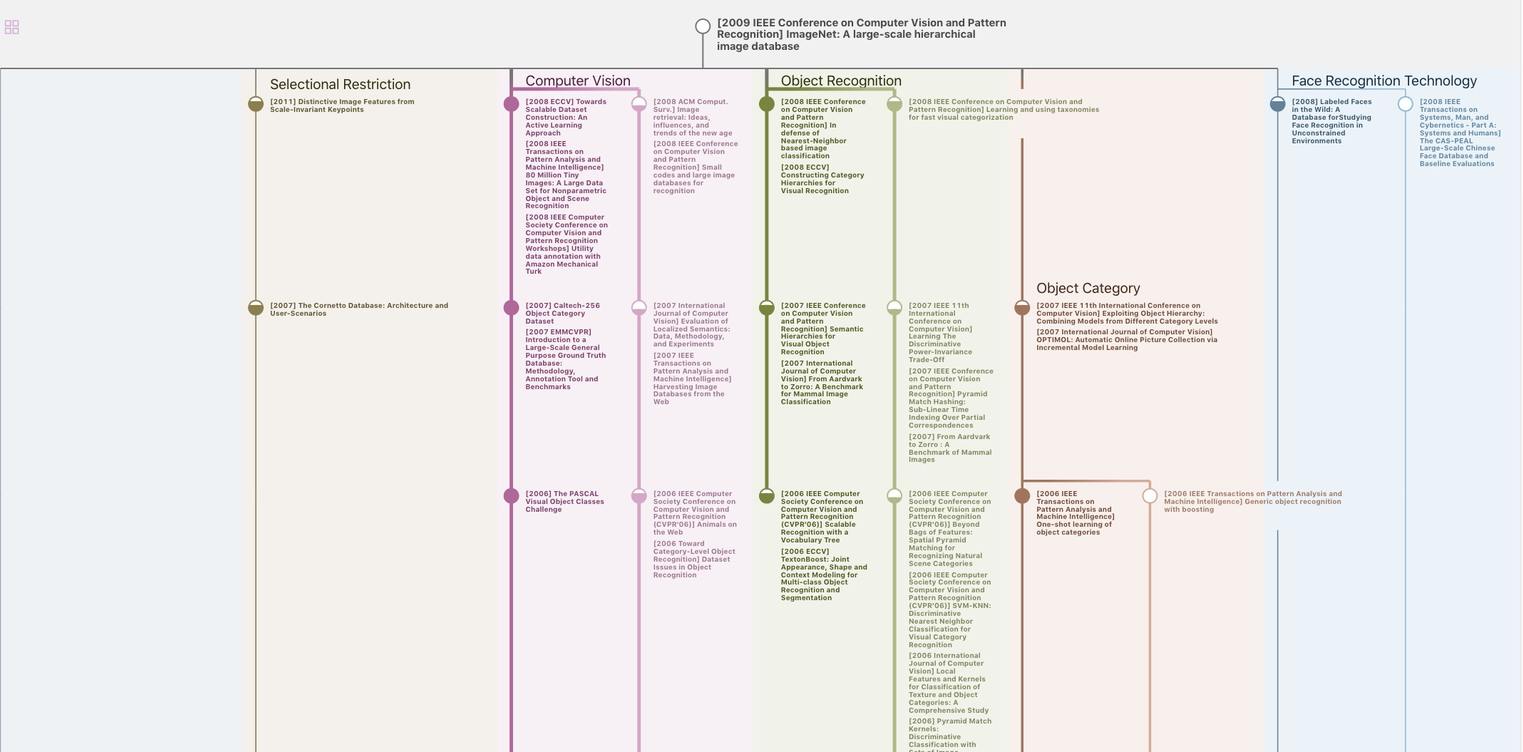
生成溯源树,研究论文发展脉络
Chat Paper
正在生成论文摘要