TrEP: Transformer-Based Evidential Prediction for Pedestrian Intention with Uncertainty.
AAAI(2023)
摘要
With rapid development in hardware (sensors and processors) and AI algorithms, automated driving techniques have entered the public's daily life and achieved great success in supporting human driving performance. However, due to the high contextual variations and temporal dynamics in pedestrian behaviors, the interaction between autonomous-driving cars and pedestrians remains challenging, impeding the development of fully autonomous driving systems. This paper focuses on predicting pedestrian intention with a novel transformer-based evidential prediction ( TrEP ) algorithm. We develop a transformer module towards the temporal correlations among the input features within pedestrian video sequences and a deep evidential learning model to capture the AI uncertainty under scene complexities. Experimental results on three popular pedestrian intent benchmarks have verified the effectiveness of our proposed model over the state-of-the-art. The algorithm performance can be further boosted by controlling the uncertainty level. We systematically compare human disagreements with AI uncertainty to further evaluate AI performance in confusing scenes.
更多查看译文
关键词
pedestrian intention,evidential prediction,uncertainty,transformer-based
AI 理解论文
溯源树
样例
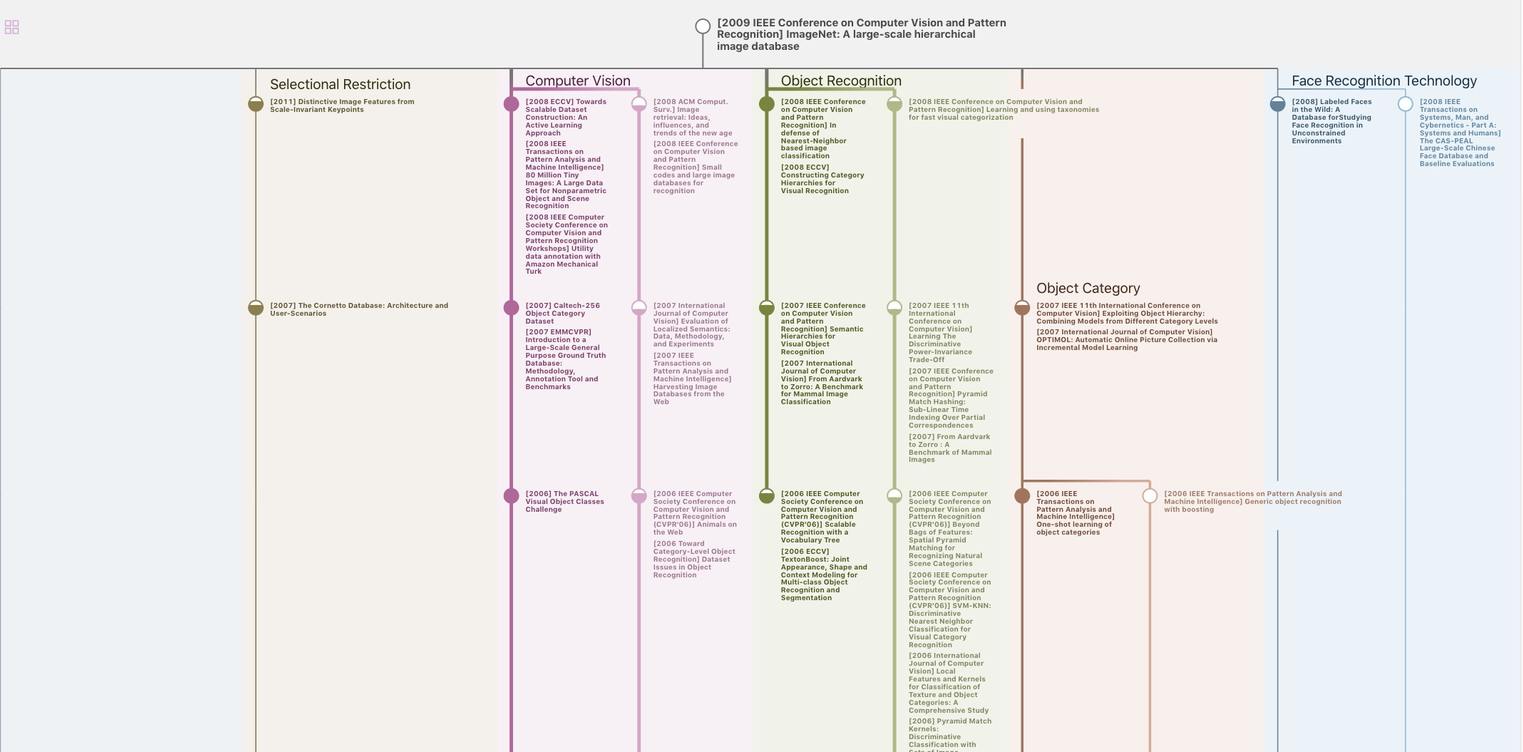
生成溯源树,研究论文发展脉络
Chat Paper
正在生成论文摘要