GradPU: Positive-Unlabeled Learning via Gradient Penalty and Positive Upweighting.
AAAI(2023)
摘要
Positive-unlabeled learning is an essential problem in many real-world applications with only labeled positive and unlabeled data, especially when the negative samples are difficult to identify. Most existing positive-unlabeled learning methods will overfit the positive class to some extent due to the existence of unidentified positive samples. This paper first analyzes the overfitting problem and proposes to bound the generalization errors via Wasserstein distances. Based on that, we develop a simple yet effective positive-unlabeled learning method, GradPU, which consists of two key ingredients: A gradient-based regularizer that penalizes the gradient norms in the interpolated data region, which improves the generalization of positive class; An unnormalized upweighting mechanism that assigns larger weights to those positive samples that are hard, not-well-fitted and less frequently labeled. It enforces the training error of each positive sample to be small and increases the robustness to the labeling bias. We evaluate our proposed GradPU on three datasets: MNIST, FashionMNIST, and CIFAR10. The results demonstrate that GradPU achieves state-of-the-art performance on both unbiased and biased positive labeling scenarios.
更多查看译文
关键词
gradient penalty,positive-unlabeled upweighting,learning
AI 理解论文
溯源树
样例
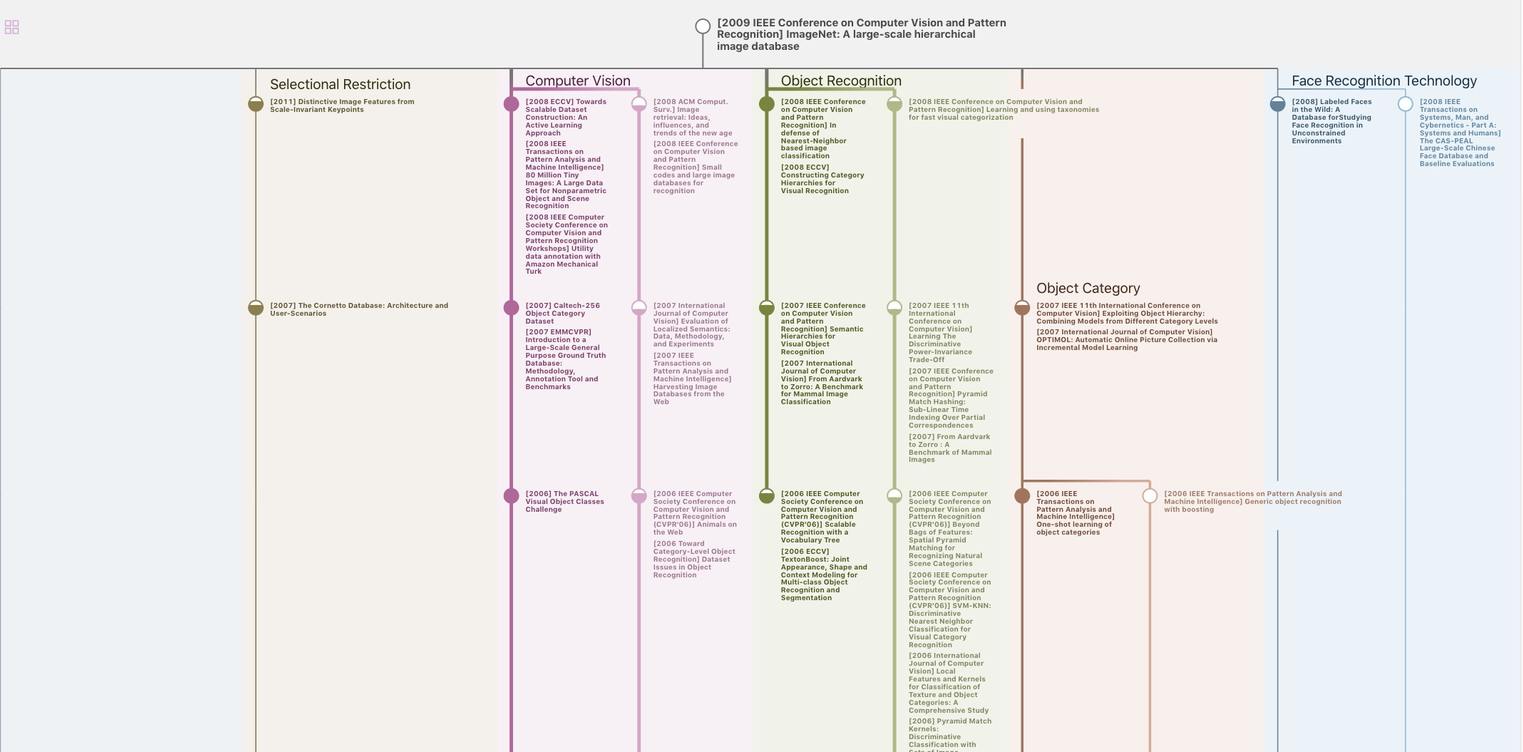
生成溯源树,研究论文发展脉络
Chat Paper
正在生成论文摘要