Robust Video Portrait Reenactment via Personalized Representation Quantization.
AAAI(2023)
摘要
While progress has been made in the field of portrait reenactment, the problem of how to produce high-fidelity and robust videos remains. Recent studies normally find it challenging to handle rarely seen target poses due to the limitation of source data. This paper proposes the Video Portrait via Non-local Quantization Modeling (VPNQ) framework, which produces pose-and disturbance-robust reenactable video portraits. Our key insight is to learn position-invariant quantized local patch representations, then build a mapping between simple driving signals and local textures with non-local spatial-temporal modeling. Specifically, instead of learning a universal quantized codebook, we identify that a personalized one can be trained to preserve desired position-invariant local details. Then, a simple representation of projected landmarks can be used as sufficient driving signals to avoid 3D rendering. In the following, we employ a carefully designed Spatio-Temporal Transformer to predict reasonable and temporally consistent quantized tokens from the driving signal. The predicted codes can be decoded back to robust and high-quality videos. Comprehensive experiments have been conducted to validate the effectiveness of our approach.
更多查看译文
关键词
robust video portrait reenactment,representation
AI 理解论文
溯源树
样例
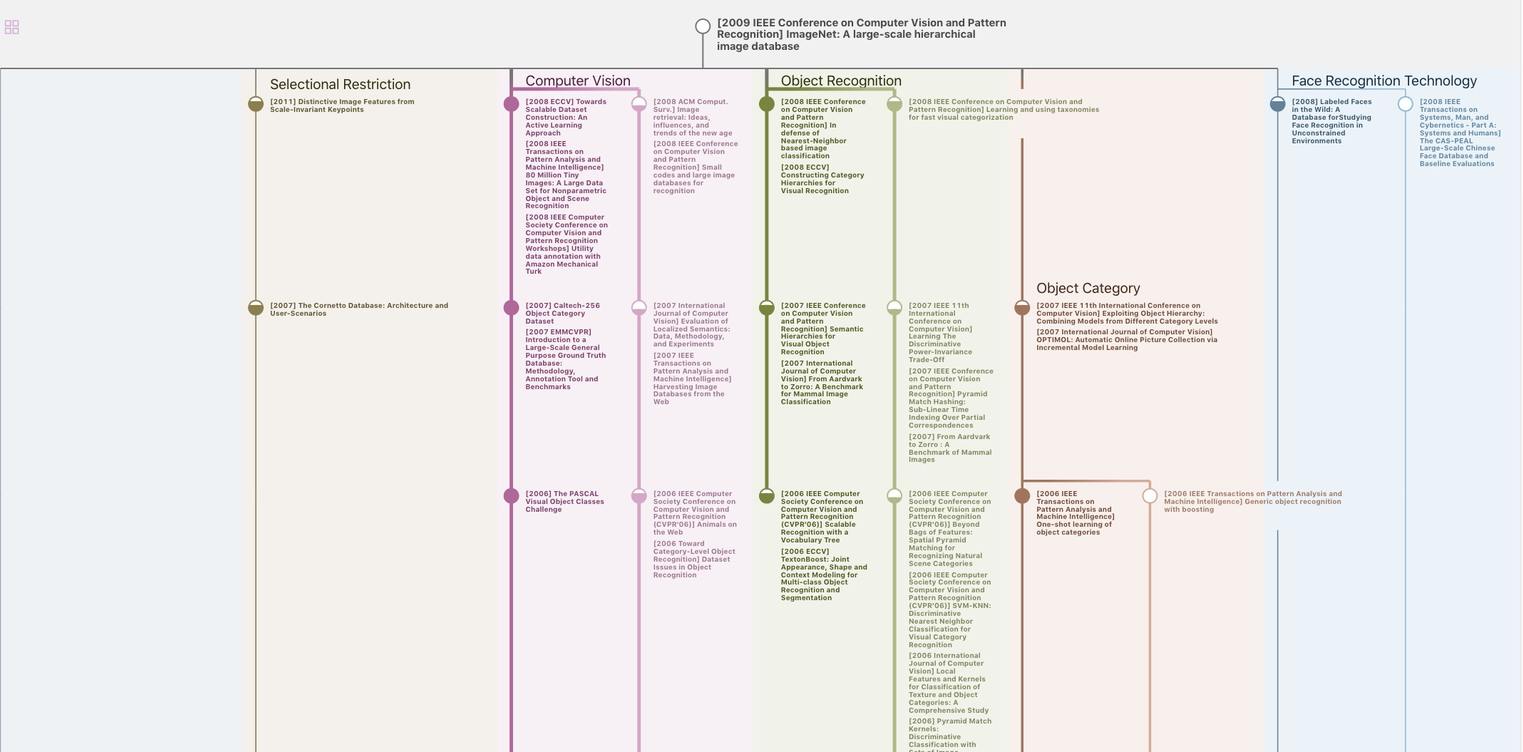
生成溯源树,研究论文发展脉络
Chat Paper
正在生成论文摘要