Semantic-Aware Superpixel for Weakly Supervised Semantic Segmentation.
AAAI(2023)
摘要
Weakly-supervised semantic segmentation aims to train a semantic segmentation network using weak labels. Among weak labels, image-level label has been the most popular choice due to its simplicity. However, since image-level labels lack accurate object region information, additional modules such as saliency detector have been exploited in weakly supervised semantic segmentation, which requires pixel-level label for training. In this paper, we explore a self-supervised vision transformer to mitigate the heavy efforts on generation of pixel-level annotations. By exploiting the features obtained from self-supervised vision transformer, our superpixel discovery method finds out the semantic-aware superpixels based on the feature similarity in an unsupervised manner. Once we obtain the superpixels, we train the semantic segmentation network using superpixel-guided seeded region growing method. Despite its simplicity, our approach achieves the competitive result with the state-of-the-arts on PASCAL VOC 2012 and MS-COCO 2014 semantic segmentation datasets for weakly supervised semantic segmentation.
更多查看译文
关键词
weakly supervised semantic-aware segmentation
AI 理解论文
溯源树
样例
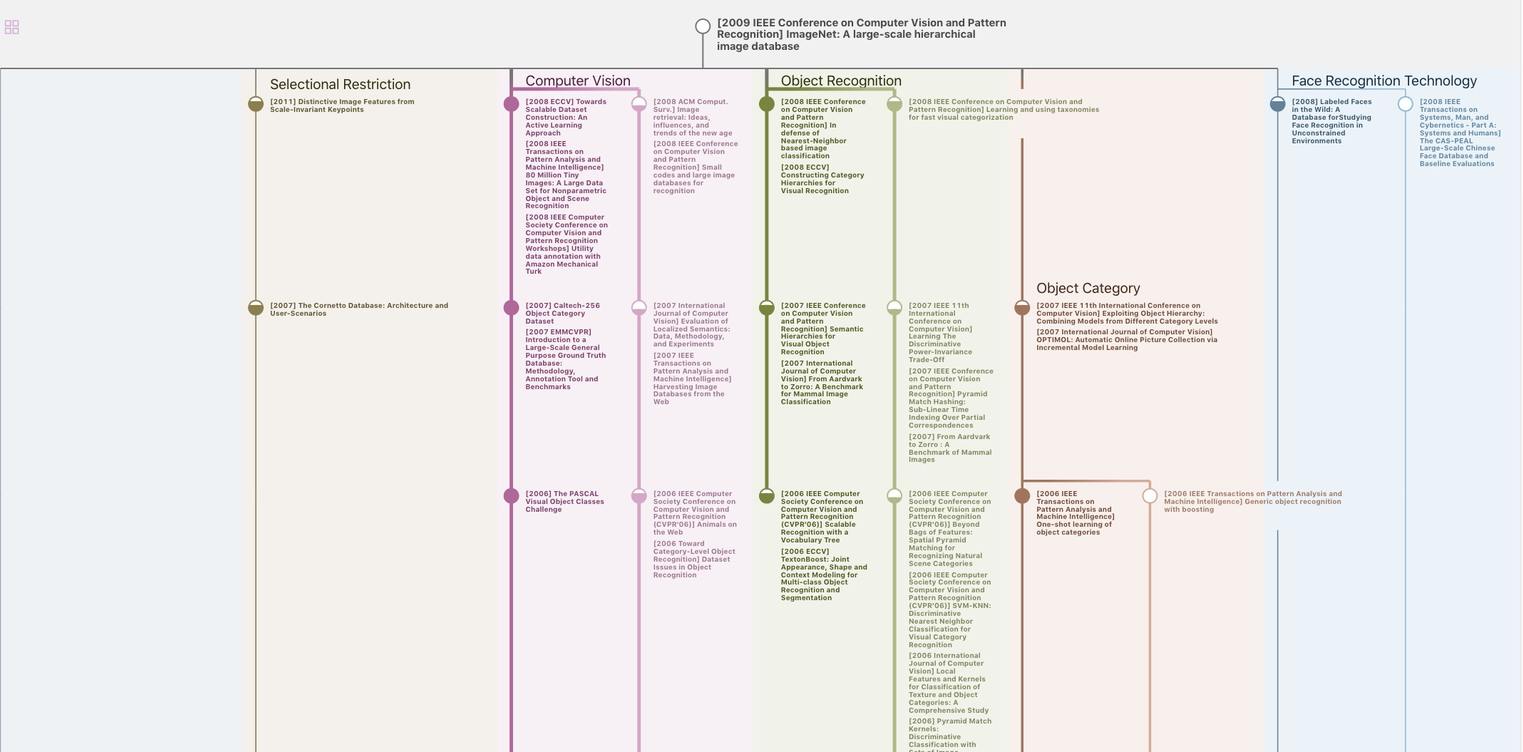
生成溯源树,研究论文发展脉络
Chat Paper
正在生成论文摘要