Detection of Fake News Through Heterogeneous Graph Interactions.
NETYS(2023)
摘要
Fake news is one of the most prominent forms of disinformation. Unfortunately, today’s advanced social media platforms allow for the rapid transmission of fake news, which may negatively impact several aspects of human society. Despite the significant progress in detecting fake news, the focus of most current work lies in the detection based on content-based or user context-based methods. We believe that such methods suffer from two limitations: the lack of characterizing the news variation (fake news can appear in different forms via tweets, such as writing different tweets about the same news article) and news repetition (fake news is shared repeatedly via retweets); and the absence of the temporal engagement among different social interactions. Thus, we propose a novel detection framework, namely the Temporal graph Fake News Detection Framework (T-FND), that is effectively able to capture heterogeneous and repetitive characteristics of fake news behavior, resulting in better prediction performance. We empirically evaluate the effectiveness of our model on two real-world datasets, showing that our solution outperforms the state-of-the-art baseline methods
更多查看译文
关键词
fake news,graph
AI 理解论文
溯源树
样例
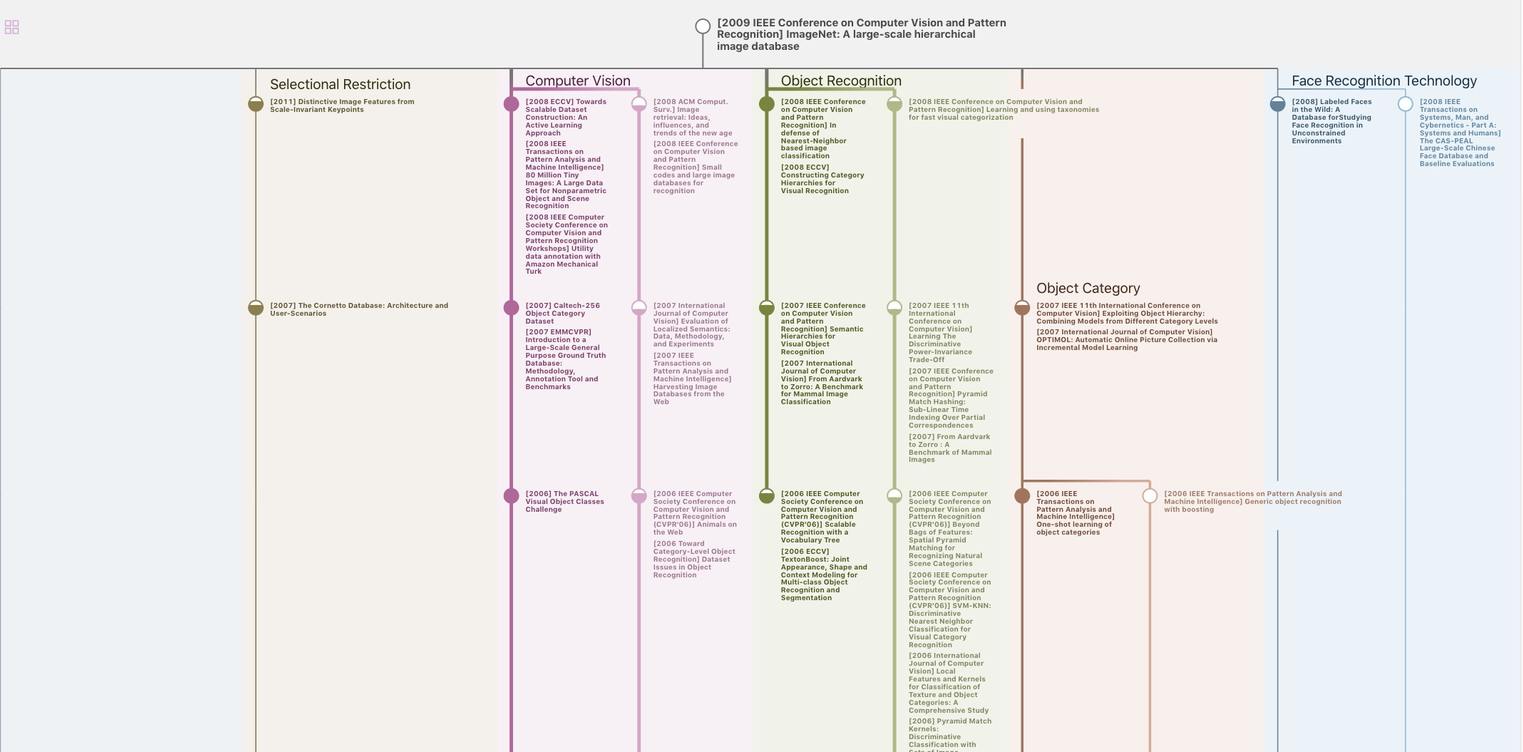
生成溯源树,研究论文发展脉络
Chat Paper
正在生成论文摘要