Siamese Autoencoder-Based Approach for Missing Data Imputation.
ICCS (1)(2023)
摘要
Missing data is an issue that can negatively impact any task performed with the available data and it is often found in real-world domains such as healthcare. One of the most common strategies to address this issue is to perform imputation, where the missing values are replaced by estimates. Several approaches based on statistics and machine learning techniques have been proposed for this purpose, including deep learning architectures such as generative adversarial networks and autoencoders. In this work, we propose a novel siamese neural network suitable for missing data imputation, which we call Siamese Autoencoder-based Approach for Imputation (SAEI). Besides having a deep autoencoder architecture, SAEI also has a custom loss function and triplet mining strategy that are tailored for the missing data issue. The proposed SAEI approach is compared to seven state-of-the-art imputation methods in an experimental setup that comprises 14 heterogeneous datasets of the healthcare domain injected with Missing Not At Random values at a rate between 10% and 60%. The results show that SAEI significantly outperforms all the remaining imputation methods for all experimented settings, achieving an average improvement of 35%.
更多查看译文
关键词
missing data imputation,autoencoder-based
AI 理解论文
溯源树
样例
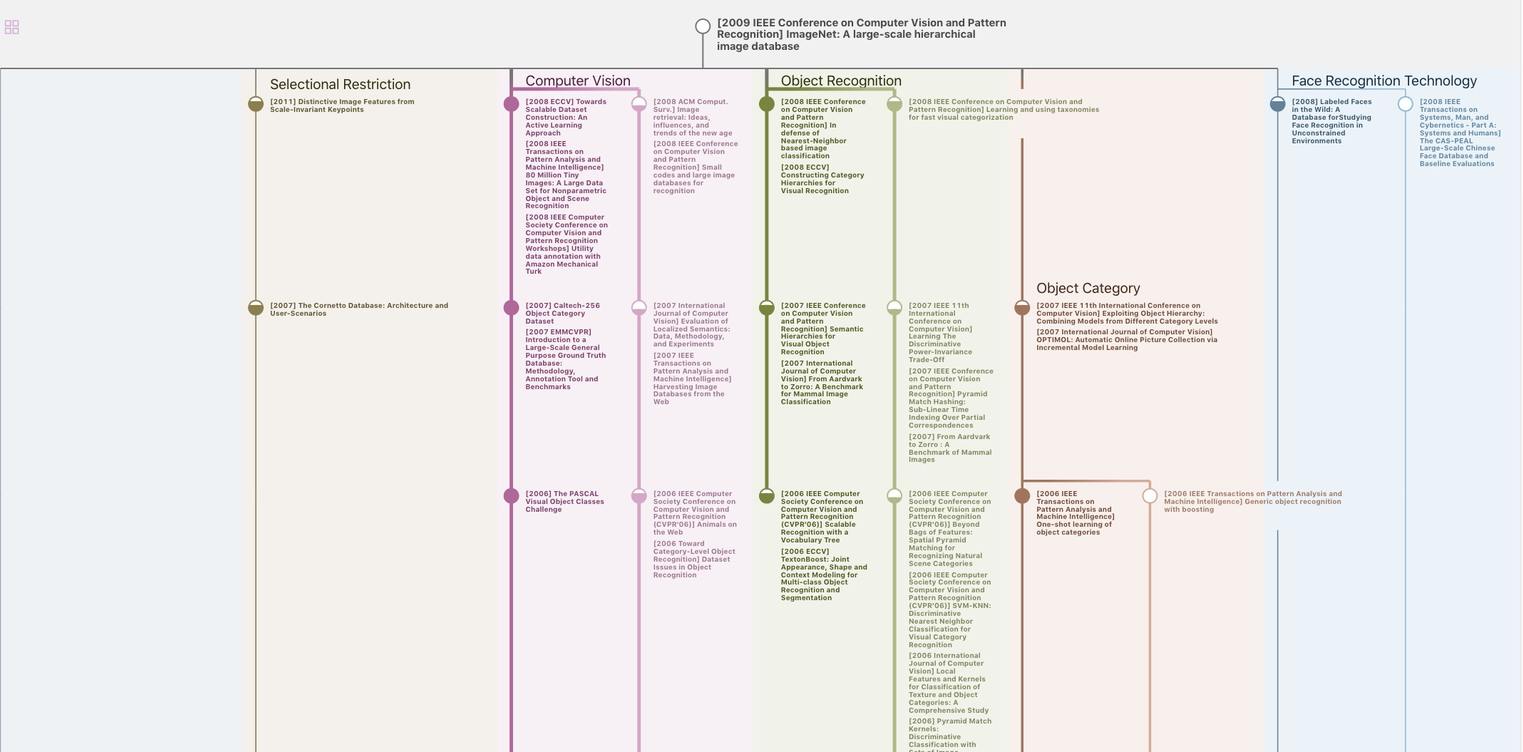
生成溯源树,研究论文发展脉络
Chat Paper
正在生成论文摘要