Optimized hybrid imbalanced data sampling for decision tree training
PROCEEDINGS OF THE 2023 GENETIC AND EVOLUTIONARY COMPUTATION CONFERENCE COMPANION, GECCO 2023 COMPANION(2023)
摘要
For many real-world decision-making tasks, a key feature is decision explainability. Hence, the so-called glass-box models offer full explainability and are still prevalent. An important area of application is the classification of imbalanced data. We require that the proposed classifiers not make errors on the minority class while minimizing errors on the majority class. This paper proposes a method for preprocessing imbalanced data by generating minority class objects. We use a multi-criteria optimization method (NSGA-II) to avoid optimizing a single aggregate criterion. The method returns a group of non-dominated solutions from which the end user can choose the best solution from his point of view. The automatic solution selection from a Pareto front is also proposed for comparison purposes. The proposed method returns good-quality classifiers, often surpassing the quality of baseline single-objective methods, and is additionally characterized by full interpretability.
更多查看译文
关键词
imbalanced data,multi-objective optimization,data sampling,decision tree classifier
AI 理解论文
溯源树
样例
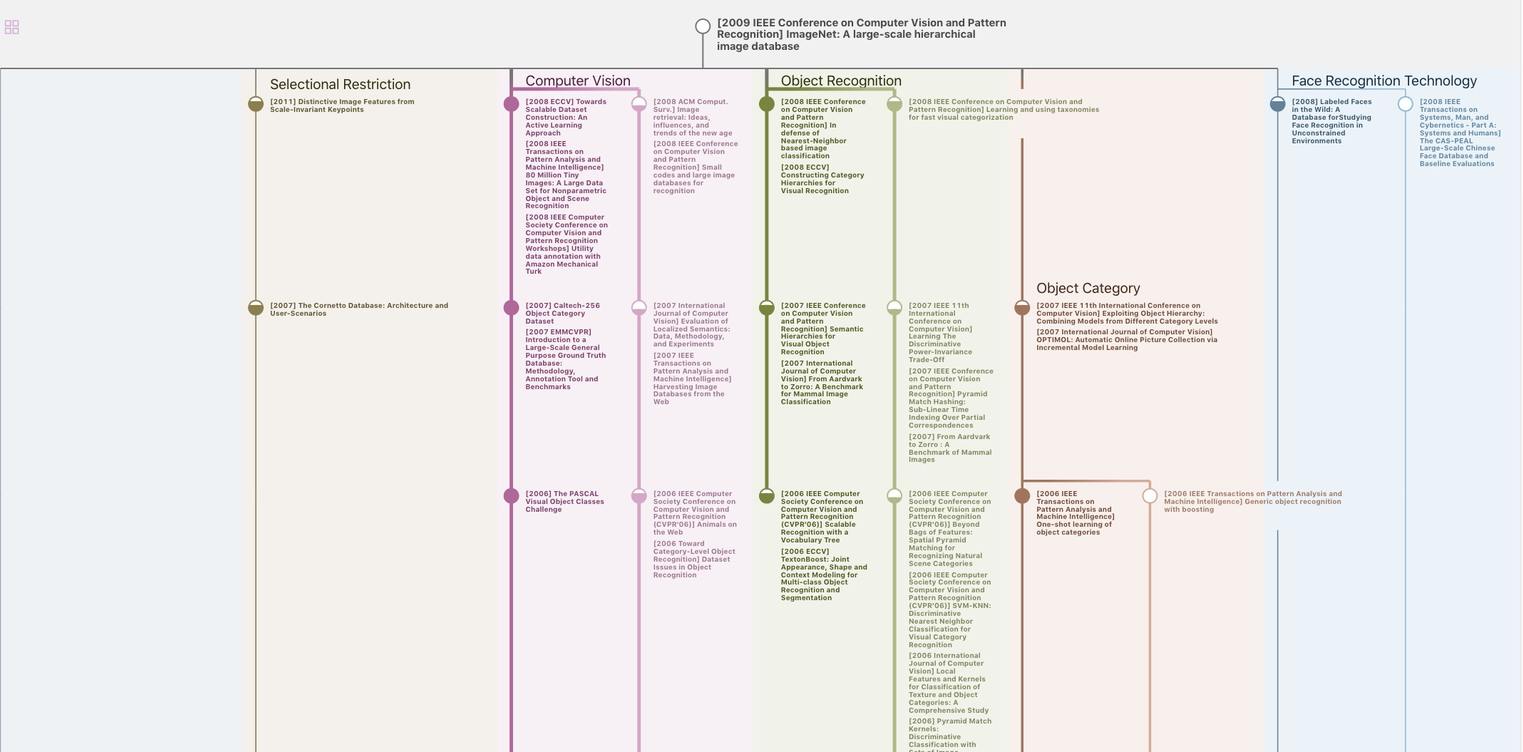
生成溯源树,研究论文发展脉络
Chat Paper
正在生成论文摘要