Real-life Performance of Fairness Interventions Introducing a New Benchmarking Dataset for Fair ML
38TH ANNUAL ACM SYMPOSIUM ON APPLIED COMPUTING, SAC 2023(2023)
摘要
Some researchers evaluate their fair Machine Learning (ML) algorithms by simulating data with a fair and biased version of its labels. The fair labels reflect what labels individuals deserve, while the biased labels reflect labels obtained through a biased decision process. Given such data, fair algorithms are evaluated by measuring how well they can predict the fair labels, after being trained on the biased ones. The big problem with these approaches is, that they are based on simulated data, which is unlikely to capture the full complexity and noise of real-life decision problems. In this paper, we show how we created a new, more realistic dataset with both fair and biased labels. For this purpose, we started with an existing dataset containing information about high school students and whether they passed an exam or not. Through a human experiment, where participants estimated the school performance given some description of these students, we collect a biased version of these labels. We show how this new dataset can be used to evaluate fair ML algorithms, and how some fairness interventions, that perform well in the traditional evaluation schemes, do not necessarily perform well with respect to the unbiased labels in our dataset, leading to new insights into the performance of debiasing techniques.
更多查看译文
关键词
Fair ML,Fairness Evaluation,Benchmarking Dataset
AI 理解论文
溯源树
样例
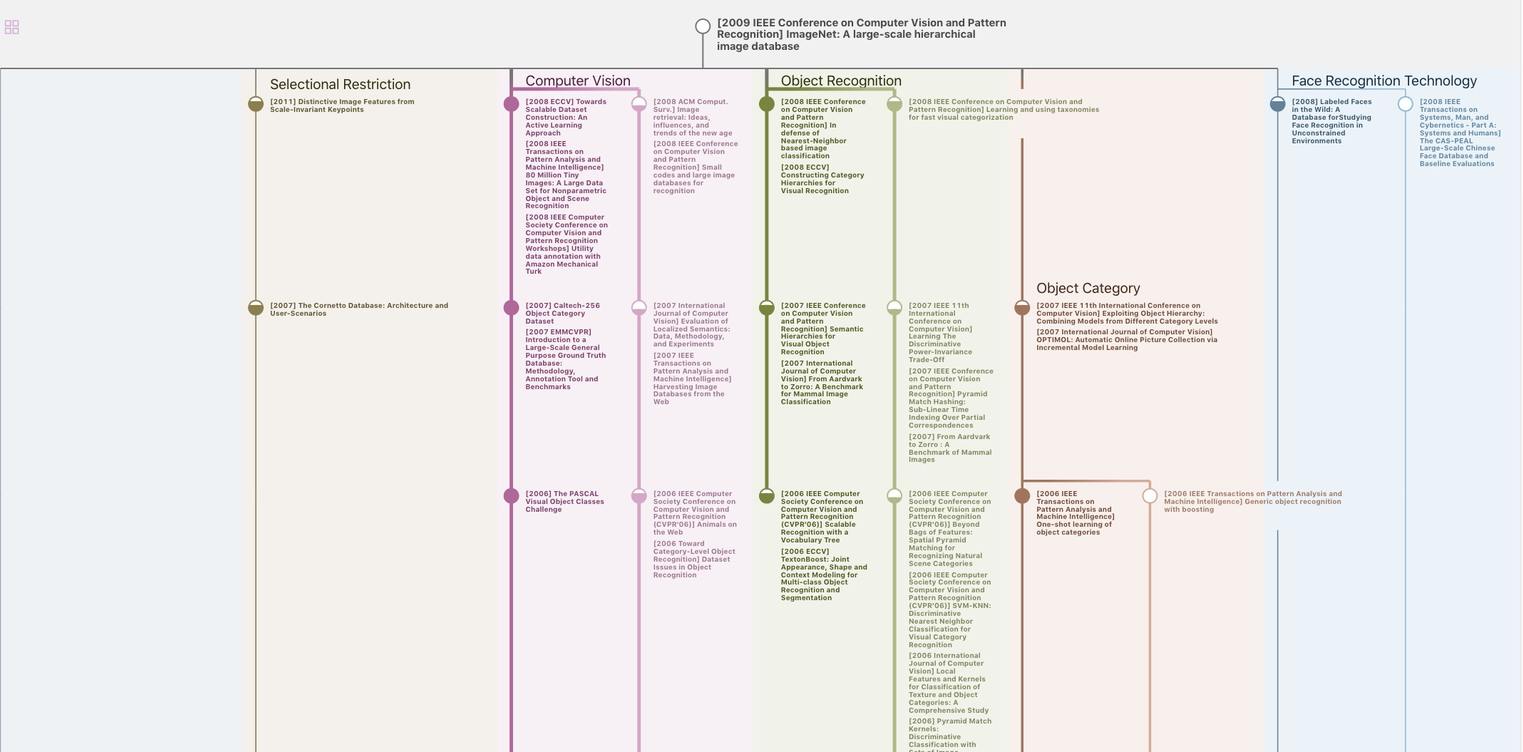
生成溯源树,研究论文发展脉络
Chat Paper
正在生成论文摘要